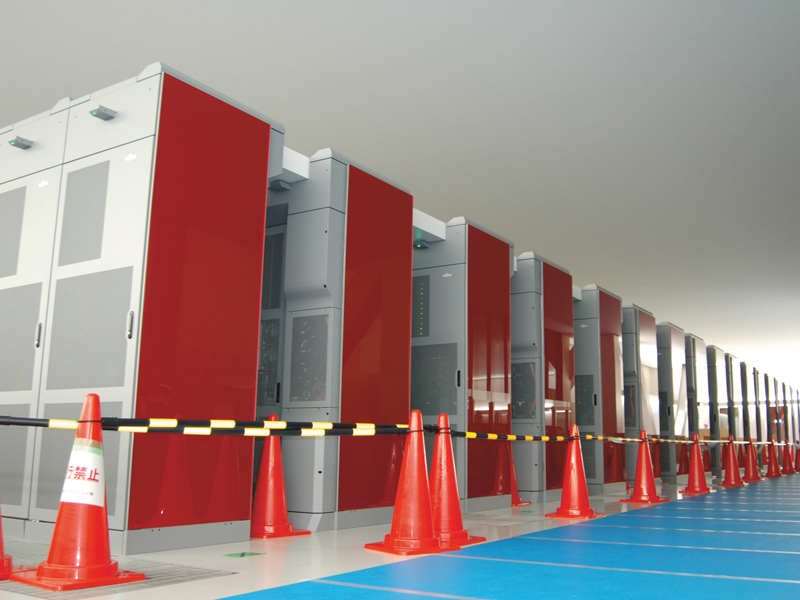
Back in June, Fujitsu's K Computer became the fastest supercomputer on Earth - so fast, in fact, that it outperforms the five next fastest supercomputers combined.
It can process an astonishing eight quadrillion calculations a second (eight petaflops), and Fujitsu had hoped that by 2012 it would have cracked the 10-petaflop barrier.
That would have been a significant achievement for several reasons. The first is that it shows just how quickly technology is progressing - as recently as 2006, scientists were getting excited about supercomputers cracking the one-petaflop barrier.
The second is that some pundits believe 10 petaflops is the processing power of the human brain - so on that basis, the K Computer will outperform the human brain within months.
Will our PCs soon be smarter than we are? Is technology telling us that our time is up?
Special K
Fujitsu's K Computer won't replace humanity any time soon, and not just because it can't climb stairs.
The K is enormous: at the time of writing its 68,544 CPUs require some 672 computer racks, and more are being added. By the time the project is complete, the K Computer will consist of more than 800 computer racks. That will take performance from 8.2 petaflops to in excess of 10 petaflops - the equivalent of linking one million desktop PCs.
Sign up for breaking news, reviews, opinion, top tech deals, and more.
Delivering that performance uses a lot of energy, and while the K Computer has been designed with efficiency as a priority, that's still relative; powering 600,000 CPUs uses enough energy to power 10,000 houses, and Fujitsu's annual electricity bill for the machine is likely to exceed $10,000.
By comparison, the human brain operates on 20-40 watts, and it's much more powerful than the K Computer. It seems the estimate of 10-petaflop processing power was on the low side: the consensus is that our brains are 10 times more powerful than that, delivering at least 100 petaflops, while some estimates are as high as 1,000 petaflops.
Even if the lower estimate is the right one, computers are some way behind: in June 2011, the combined processing power of the world's fastest 500 supercomputers was 58.9 petaflops - or half a brain.
That's not the only area in which computers are lagging behind. Where the human brain fits, handily, into a human skull, supercomputers need considerably more room. The K Computer's planned 800 server racks may be on the large side, but supercomputer power isn't something you can achieve in something significantly smaller.
In 2009, the US defense technology agency DARPA issued a challenge to the IT community, asking it to build a petaflop supercomputer small enough to fit into a single 19-inch cabinet and efficient enough to use no more than 57 kilowatts of power - tiny amounts by computer standards, but still enormous compared to our brains. Nobody has built it yet.
While IBM predicts that a human-rivalling, real-time supercomputer may well exist by 2019, it also predicts that the computer would need a dedicated nuclear power station to run it.
Thanks for the memory
We know roughly how much processing power our electronic brain would need to have, but what about storage? Our brains don't just process data; they store enormous amounts of it for instant access. How many hard disks would we need to emulate that?
The answer depends on where data is actually stored in our brains. If it's stored in our neurons, with one neuron storing one bit of information, then our brains should be able to store 50 to 100 billion bits of data, which works out at around five to 10 gigabytes.
However, if the brain stores data not in the neurons but in the tens of thousands of synapses around each neuron, then our brain capacity would be in the hundreds of terabytes, possibly even petabytes.
That assumes, of course, that the brain stores data like computers do, which it probably doesn't, and that we can measure memories in the same way we measure digital data, which we almost certainly can't. For example, when we store a memory, do we store the whole thing, or is it truncated? Does our brain run data compression routines, and if it does, how lossy are they?
Some neuroscientists are studying that very thing. In February, neuroscientist Ed Connor published a paper in the journal Current Biology with the catchy title A Sparse Object Coding Scheme in Area V4.
In it, Connor described how the brain compresses data in much the same way JPEG compression reduces the size of photographs: while our eyes deliver megapixel images, our brain prefers smaller sizes and concentrates on storing only the key bits of information it needs to recall things correctly in the future. Connor dubbed the results '.brain files', and said in a statement that "for now, at least, the '.brain' format seems to be the best compression algorithm around."
The more we learn about the brain and its systems, the more complicated it appears. In December, Duke University researcher Tobias Egner found evidence that the brain's visual circuits edit what we see before we actually see it, in a model called predictive coding.
Predictive coding reverses our view - no pun intended - of how our visual circuits work. Whereas previously it was believed that our brains processed the entire image with increasing levels of detail, sending data up a 'neuron ladder' until the entire image had been recognised, predictive coding suggests that our brains first take a guess at what they expect to see, then the neurons work out what's different from that guess.
The predictive coding research demonstrates one of the problems with mimicking the human brain: we're not entirely sure what it is we're mimicking, and the more we find out the harder the task becomes.
Thore Graepel is a principal researcher with Microsoft Research in Cambridge, where he specialises in machine learning and probabilistic modelling. "I don't think people are even entirely sure what the computational power of the brain actually is," he told us.
"If we take the standard numbers like 100 billion neurons in the brain, with 1,000 trillion connections between them, then of course if you just transfer that to bits at a certain resolution then you get some numbers - but the differences between the architectures of the systems are so great that it's really hard to compare. In some senses we're competing with five million years of development."
Competing with millions of years of evolution is difficult and expensive. So why not cheat?
Imitation of life
When the Los Alamos National Lab unveiled its petaflop-scale supercomputer Roadrunner in 2008, there was much speculation about its ability to drive in rush-hour traffic - or at least its theoretical ability, as the 227-tonne machine was a bit big to stick in a hatchback. It turns out that just mimicking what we do in traffic requires petaflop-scale processing, which simply isn't portable enough given today's technology.
That doesn't mean computer-controlled cars don't exist yet, though. They do, and they've been bumping around a Californian air base. The DARPA Urban Challenge pitted 11 computer-controlled cars against one another. There were 89 entrants, but only 35 were accepted; of that 35, only 11 made it through a week of pre-race testing.
The 11 competitors were challenged to navigate a range of urban environments that included moving traffic. Six vehicles successfully navigated the course, and the winner was a Chevrolet Tahoe SUV from Carnegie Mellon University in association with General Motors.
The SUV, dubbed Boss, didn't have a petaflop-scale supercomputer, but it did have GPS, long and short range radar, and LIDAR optical range sensors. Those extra senses enabled it to achieve human-like performance without requiring a human-like brain.
There's another, even cheaper way to get human-style performance from a computer: use humans. That's exactly what Facebook did to build its enormous facial recognition database.
By its own account, Facebook's users add more than 100 million tags to Facebook photos every day. By relying on those tags and looking only at possible matches from a user's social circle, Facebook's automatic facial recognition system doesn't have much heavy lifting to do.
Looks familiar
That said, facial recognition software is improving rapidly: in August, Carnegie Mellon University researcher Alessandro Acquisti demonstrated an iPhone app that can take your photo, analyse it with facial recognition software and display your name and other information.
In a few years, Acquisti predicts, "facial visual searches will become as common as today's text-based searches", with software scanning public photos like Facebook profile pictures and using them to identify individuals in crowds.
Accuracy is currently around 30 per cent, but the bigger your database and the more processing power you can throw at the problem, the better the results you'll get. It isn't hard to imagine a Kinect-style sensor that knows who you are, what you're doing and what you're trying to ask your computer to do.
"Having additional sensors is a very powerful technique to get around problems," Graepel says. "For example, in our recent development with Kinect, researchers have spent decades looking at stereo vision: the idea of using two cameras to look at a scene and then using the disparity to infer depth and do the processing of the 3D scene. That was very brittle and required a lot of processing power - and then the Kinect sensor came along and you can do much more.
"But the basic advance was on the sensor side, not on the computational side. Similar examples would include RFID tags: if an object is tagged, you don't need to point a camera to see what it is; you can read the tag and find out what it is. Sensors can make computers appear to be more intelligent, or to emulate intelligence in a way that can be extremely useful."
- 1
- 2
Current page: Could technology make your brain redundant?
Next Page AI and brain-machine interfaces