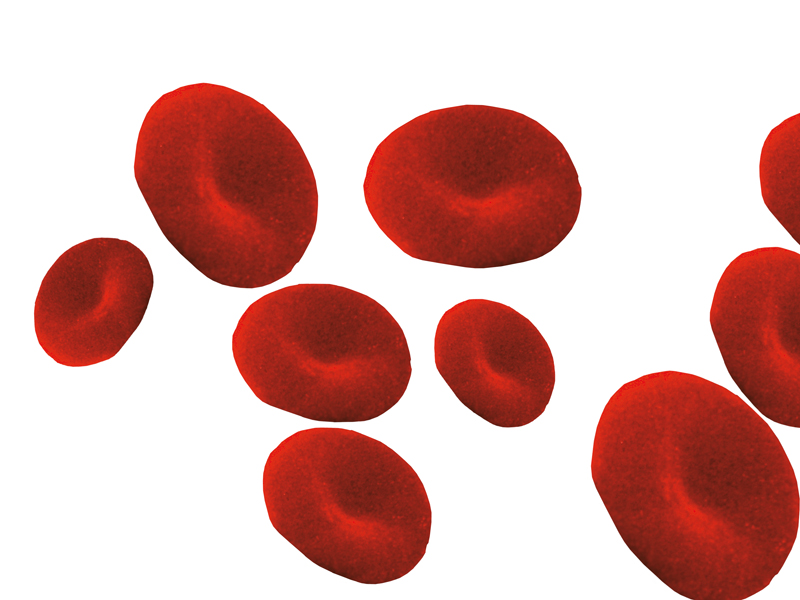
Cancer is one of the most dreaded diseases in the developed world. Its forms are many and its symptoms are diverse, but all variants cause pain and suffering.
Finding a cure for the disease is perhaps the most enduring medical dream of all, and increasingly the hope of medical researchers lies with fast-developing computing technology.
Can computers cure cancer? We don't know the answer to that question yet. But what we do know is that no other field shows us more vividly what computers can do. At Ohio State University Medical Center – home of one of the fastest supercomputers in the world – scientists are weighing proteins in order to find and measure the microscopic differences between healthy and abnormal cells.
At the Swedish Medical Center in Seattle, gene-sequencing techniques are providing rich information about brain cancer – considered the most challenging disease to research – and its potential treatment. And at the School of Informatics at Indiana University, researchers have used colossal computers to create a huge database of cell structures, hoping to understand exactly how they work and – most importantly – how they interact with each other.
Though the study of cancer also shows what computers can't do, it's by focusing on and resolving these problems that a cure may be discovered and the power of the technology advanced further.
Finding the magic bullet
One of the main goals in cancer research is to find a 'magic bullet' that can enter the human body, find mutated cells, target specific proteins in order to switch off the cancer's self-replications and destroy the mutated cells. Part of the obstacle to achieving this cure is knowing enough about the cancer cells and molecules.
Get daily insight, inspiration and deals in your inbox
Sign up for breaking news, reviews, opinion, top tech deals, and more.
Jake Chen, an assistant professor at Indiana University, says that this process – called 'finding drug targets' – requires a massive database of biomedical information. His team has developed one of the largest Oracle-powered relational databases, holding about one half of a terabyte. Chen and his team – who have focused their efforts on breast cancer research, one of the most common forms of cancer – are currently analysing tens of GBs of raw mass spectrometry data from 80 blood samples, with more coming soon.
These samples should help to further research into our understanding of the relationships between cancer and normal cells down at the molecular level, which is a particular difficulty at the moment. To help with this, Chen's team created complex algorithms not widely used in the biomedical field. The algorithms analyse not just the characteristics of individual molecules but also how each one affects others. This is what makes cancer research so complex – the interrelationships that exist and the data analysis required.
Chen says that the closest analogy to this relational study is the Internet itself. For example, the servers for AOL are widely known on the web, and it's easy to see the links between one AOL server and another. Yet there are many servers on the outer edges of the web which only link to a few others. These are the 'molecules' that are harder to understand. When one of them crashes, it can effect that part of the Internet in adverse ways – causing server outages, for example.
Data visualisation software can help researchers understand these 'fringe' areas of systems biology. Correlating the data requires complex algorithms which are still evolving. It might mean culling data from 100 other researchers around the world who have all found a likely protein target, analysing 25,000 genes and a few hundred thousand protein fragments, archiving the data for later retrieval and finally processing the algorithms using the Big Red cluster at Indiana University. It's a highly collaborative effort.
"The answer is in the data set, but the computer is not intelligent enough yet," says Chen. "We need to make the computer smarter. Today's computers are used primarily for managing information; we need to make them smart about interpreting the data."
Chen had an interesting analogy for how this works. When you look at a painting of a face, you can see what it is immediately. A computer can analyse the colours and chemicals of the painting, but it's not clever enough to see the face. Similarly, Chen is trying to produce an algorithm that can see through the noise of intricate molecular interaction networks in cancer and find the critical proteins where drug interventions may occur.
Part of the computational challenge is transferring what we already know about curing cancer in mice to humans. The drugs used to cure cancer in mice could be used for humans, but they might provoke a different set of side effects. The informatics question is how to find a cure that works on 100 per cent of cancer patients. "This exciting conquest will likely go on in the next one to two decades, and will rely on systems biology informatics techniques," says Chen.