Google just used machine learning to find the first solar system like our own
NASA used the search engine's machine learning tech to discover an eighth planet in the Kepler-90 system
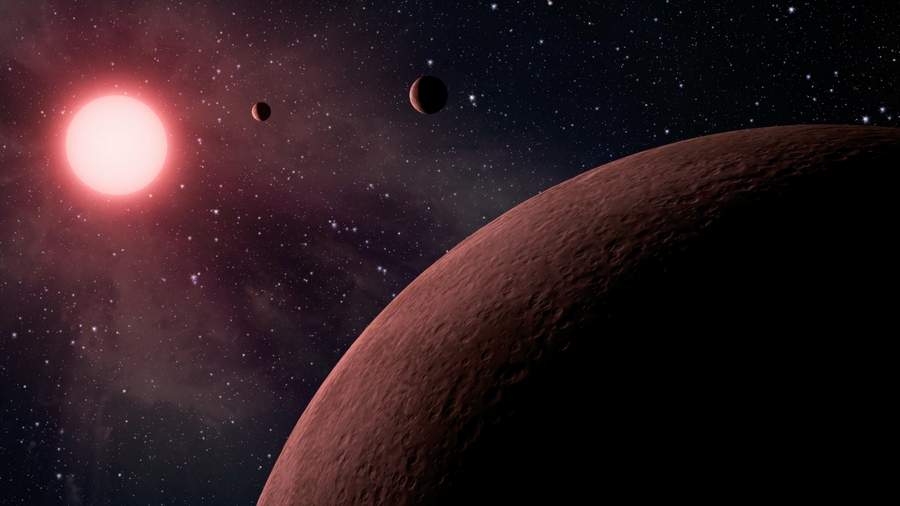
For the first time, another solar system has been found in our galaxy with eight planets, just like our own – and it was Google's artificial intelligence that found it.
Using machine learning and neural networks to achieve something humans could not, weak signal data from NASA's Kepler space telescope was scrutinized using Google machine learning technology to make the discovery.
What has NASA discovered?
The discovery of an eighth planet circling the distant star Kepler-90 system – which lies 2,545 light years away in the constellation of Draco in the northern sky – marks the first time that another solar system has been found with the same number of planets as our own. Kepler-90i is a hot, rocky planet orbiting its star once every 14.4 days, and it was found by University of Texas at Austin astronomer Andrew Vanderburg and Christopher Shallue, a senior software engineer at Google AI in Mountain View, California.
They did it by using a computer that 'learned' to find planets in data from NASA’s Kepler space telescope. The research paper reporting this finding has been accepted for publication in The Astronomical Journal.
What is Kepler-90i like?
“Kepler 90i is a G0 star a bit lighter than our Sun,” said Vanderburg. “It's the smallest of the eight planets, and we think it's rocky and the surface is scorching hot.”
Like in our solar system, the smaller planets in the Kepler-90 system are found nearer the star, and the bigger planets further away. “The Kepler-90 star system is like a mini version of our solar system. You have small planets inside and big planets outside, but everything is scrunched in much closer,” said Vanderburg. “In Kepler data we see this quite a bit – there are a lot of systems that are a lot more tightly packed that our own.” Its outermost planet, Kepler-90h, orbits at a similar distance to its star as Earth does to the Sun.
What is Kepler?
Orbiting the Sun since its launch in 2009, the Kepler Space Telescope's main mission is already over. After finding thousands of exoplanets in a tiny area of the night sky near the constellation of Lyra over the Milky Way, Kepler’s gyroscopes were damaged in 2014. Now in its second 'K2' mission it looks for exoplanets in unplanned regions of space along the ecliptic. So far it's found 2,525 planets in total.
Get daily insight, inspiration and deals in your inbox
Sign up for breaking news, reviews, opinion, top tech deals, and more.
The four-year dataset from Kepler contains more than 35,000 strong signals of possible planets circling distant stars, data that researchers have already sifted through and classified as either 'planet' or 'not planet'. However, the archive also contain many more weak signals, far too many to study more closely. Cue machine learning.
What is machine learning?
A subset of artificial intelligence (AI), machine learning is about automating repetitive tasks, essentially training a computer to recognize patterns and categorize data. The classic example is photographs of cats and dogs; show a computer millions of such pictures, each categorized as either a cat or a dog, and the computer can learn to identify them automatically. The computer creates a neural network like the human brain. This is exactly what Google has done, though on a much smaller scale.
How did Google use its machine learning tech?
The researchers used TensaFlow, an open source software library produced by Google, and it only took two hours to train the computer model using a regular desktop PC. “A neural network is a machine learning algorithm inspired by the human brain,” explained Shallue. “It can be used to classify inputs, in this case signals from the Kepler telescope, as either planet, or not planet. It does that by learning by example, so we train the model by giving it a large set of labelled examples so it can learn which patterns it can use to make the decision on whether the data is in one category or the other.”
Planets are detected using the transit method; when an exoplanet passes across a star as seen from a telescope, that star dims slightly. The light signature recorded by Kepler can then be used to calculate the mass and radius of the planet.
The researchers taught a computer to learn how to identify the weak signals of a planet crossing its star, getting it to search for planets around 670 known multiple-planet systems. “It learned to distinguish patterns caused by actual planets from patterns caused by things other than planets,” said Shallue. “The key is to let the computer learn by example rather than by humans inputting specific rules.”
As well as the key discovery of Kepler-90i, and other planet called Kepler-80g – an Earth-sized planet – was found in the Kepler-80 system in the constellation of Cygnus.
How impressive is this?
There is currently an explosion of interest in machine learning and artificial intelligence, and since the dataset used here is pretty small, it's a big deal – particularly for astronomy.
“The training set of already labeled signals contained 150,000 examples already labelled by human scientists, which is a small training set – typically we work with millions of examples within training sets,” said Shallue.
However, the process used – supervised machine learning – is the most basic. The next step is unsupervised machine learning.
“Another approach is unsupervised learning, where you don't have the right answers, you just have data, and you ask the computer to learn things without specifically telling it what the right answers are,” said Shallue. “It's an exciting and an active area of machine learning, but the large successful applications of machine learning have so far come about from the supervised method.”
Can Google used this technology to find more planets?
Almost certainly.
“We have concrete plans to look at the reminder of the data set from the main Kepler mission, which is at least 150,000 stars,” says Shallue, who pointed out that the key part is that machine learning can be used to look at more signals than it would be reasonably possible for humans to study.
“We searched for new planets around stars already known to host transiting planets, so we knew the Kepler telescope could detect them,” he said. “So when we turn to new stars, there's probably going to be a lot that don't have orbits.” That's the downside of the transiting method; even if multiple planets are orbiting a star that the telescope is pointed at, it will only see them if the the planets pass directly in front of the star – it's all about line-of-sight.
Will machine learning change astronomy?
So will machine learning put astronomers out of work? “What we've developed here is a tool to enable astronomers to have more impact, to increase the productivity and it certainly won't replace them,” said Shallue. “I hope it may give people more of a reason to go into astronomy to use more advanced technology like this.”
Next up for planet-hunters comes the first-ever space-borne all-sky transit survey, the Transiting Exoplanet Survey Satellite (TESS) space telescope, a two-year survey of over 200,000 stars. It's due for launch in March 2018.
Jamie is a freelance tech, travel and space journalist based in the UK. He’s been writing regularly for Techradar since it was launched in 2008 and also writes regularly for Forbes, The Telegraph, the South China Morning Post, Sky & Telescope and the Sky At Night magazine as well as other Future titles T3, Digital Camera World, All About Space and Space.com. He also edits two of his own websites, TravGear.com and WhenIsTheNextEclipse.com that reflect his obsession with travel gear and solar eclipse travel. He is the author of A Stargazing Program For Beginners (Springer, 2015),