Digg's lead scientist talks collaborative filtering
SXSWi 2009: Anton Kast on providing personalised content
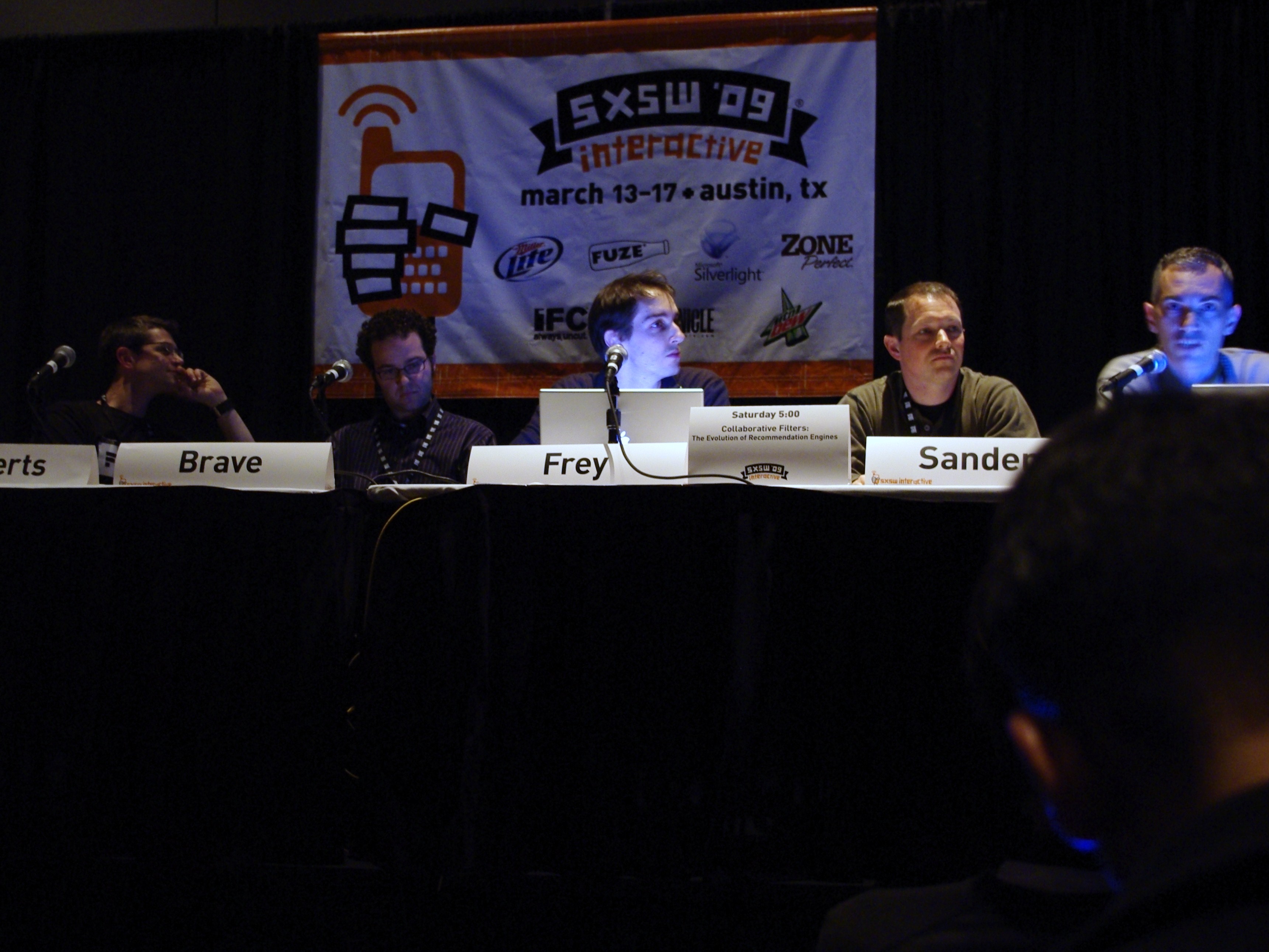
Behind the scenes of many websites, collaborative filters are tapping preferences of people similar to you to help recommend other products, stories or links that you might enjoy.
You'll have seen these filters in action in Amazon's 'Customers who bought this item also bought' feature, in Digg's 'Recommendations in upcoming', and many other places.
At South By South West Interactive a panel consisting of representatives from Digg, The Filter, Baynote, Netflix and Last.fm came together to talk about the importance of these recommendation engines.
Anton Kast, lead scientist at Digg, explained how these filters started out with email and Usenet filtering based on people's ratings, before moving out of the research sphere and onto the everyday web.
"The idea of collaborative filtering is simply to combine the input from many different people to filter information better than would otherwise be possible. In particular, you use information from many independent judgements by many people, to do something you couldn't have done just with computer science and metadata and facts that did not come from real humans."
Kast continues: "This technique is everywhere. It may sound obscure, it may sound specialised, but it's actually so simple that's it nearly universal."
Common examples include Gmail spam filters, PageRank, tagging of YouTube videos, voting up and down of comments on forums and help systems.
Get daily insight, inspiration and deals in your inbox
Sign up for breaking news, reviews, opinion, top tech deals, and more.
So that's collaborative filtering, but what is recommendation?
"Any collaborative filtering where the output is personalised," says Kast, pointing at recommendations on Amazon, of music on Last.fm and movies on Netflis as examples.
And, of course, collaborative filtering appears on Digg. "On Digg anyone can submit a story," says Kast. "And anyone can vote on any story – that's the filtering part, and whatever is most popular wins. It's a giant collaborative filter in the simplest, classic sense. But if you log in we will look at your voting history, correlate you with other people's voting histories, and find stories that these other people liked and show you those, so you get personalised collaborative filtering."
But there are four basic problems with this approach, says Kast.
The first is sparsity: "people doing the filtering are sparse compared with amount of content that needs filtering," Kast explains. "If there's many more Digg stories than there are people voting in there then obviously we're not getting good coverage.
"Second is the early rater problem, where something is just submitted and you do not have a lot of voting information for filtering purposes."
Third is what Kast refers to as "the grey sheep problem" – where whatever is most popular goes on the home page, "and so stuff that is not particularly popular but that a small group of people is crazy about – how do you serve that small group of people?"
And finally, says Kast, there's user opposition. "Digg has this fascinating history where every once in a while a large number of people get incredibly enthusiastic about one thing and it ends up on our home page and fights goals we have to represent small groups or have diverse content but that's just a fundamental problem – when you're relying on people, there's popular will."
After watching War Games and Tron more times that is healthy, Paul (Twitter, Google+) took his first steps online via a BBC Micro and acoustic coupler back in 1985, and has been finding excuses to spend the day online ever since. This includes roles editing .net magazine, launching the Official Windows Magazine, and now as Global EiC of TechRadar.