What is Generative AI (GAI)?
See how generative AI links creativity with computation, bringing data-driven artistry to life
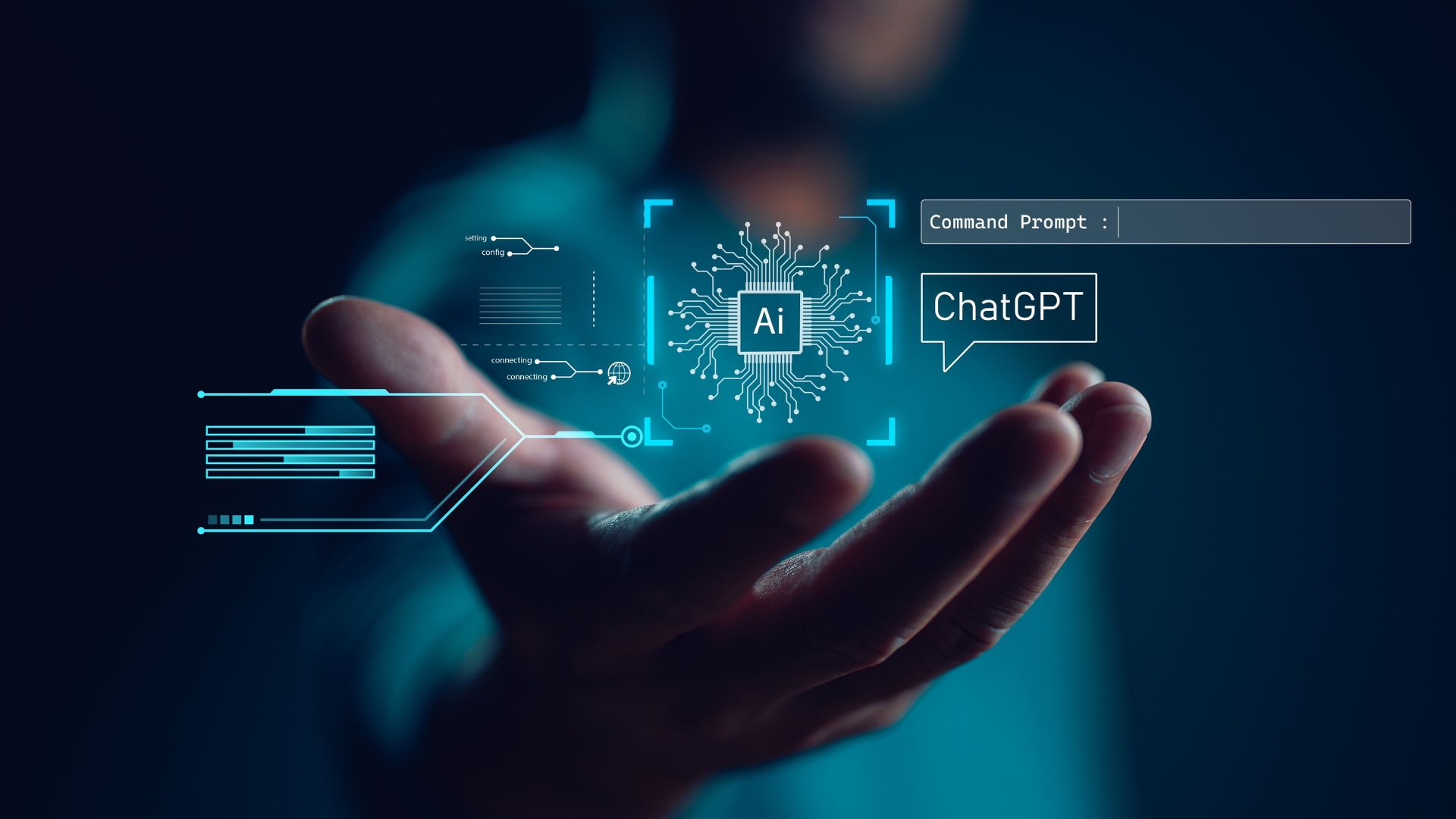
Picture a world where machines can write stories, create stunning artwork, or compose music - no, it’s not the plot of a sci-fi movie, it’s the magic of generative AI (GAI).
In recent years, artificial intelligence (AI) has gone from playing a supporting role to taking center stage in technological innovation. Among its many branches, generative AI acts as the “artist” of the AI family, with algorithms that can create completely new content from scratch.
In today’s tech-driven world, where creativity meets automation, generative AI is transforming industries, reshaping how we think about creativity, and proving that machines are capable of far more than just number-crunching. But how does it work, and what sets it apart from the AI we’re used to?
Now, let’s explore the fascinating world of generative AI and its boundless potential - don’t worry, it’s much less artificial than it appears.
What is generative AI?
Generative AI goes beyond simply obeying commands - it also creates. You could call it the creative mastermind of the AI world, capable of generating fresh content - whether it’s AI images, text, music, or videos - all from a basic prompt or dataset. Unlike “old-school” AI models, which typically analyze data to make decisions or predictions, generative AI goes one step further - it crafts something new.
At its core, GAI uses algorithms and vast amounts of data to learn patterns. Once trained, it can generate content that mimics what it has learned but with its own unique twist.
In short, generative AI isn’t just automating tasks - it’s creating whole worlds of possibility, where machines not only process but also produce.
Sign up to the TechRadar Pro newsletter to get all the top news, opinion, features and guidance your business needs to succeed!
What's the difference between generative AI and other types of AI
Artificial intelligence includes a range of technologies, with “traditional” AI and generative AI (GAI) at the forefront.
Traditional AI, or narrow AI, is like a specialist with a focused expertise. It performs specific tasks based on pre-programmed algorithms. For instance, AI chatbots, autonomous vehicles, and spam filters use traditional AI. It excels in areas where predefined algorithms can handle well-defined problems, operating with clear, predictable rules that make its decision-making process relatively transparent.
Meanwhile, GAI is its creative counterpart. Unlike traditional AI, which sticks to analyzing and predicting, GAI creates new content from existing data. It uses advanced algorithms like GANs (generative adversarial networks) and VAEs (variational autoencoders) to generate original text, images, and music based on our prompts.
However, this creativity comes with a trade-off. The complex algorithms used in generative AI can make its output generation less transparent, as it’s often harder to trace exactly how the results are produced.
So, while traditional AI is a whiz at analyzing data and handling repetitive tasks, generative AI is where the magic happens, bringing new media to life.
Evolution of AI leading to generative AI
AI has come a long way from its early days, evolving through various stages to become the sophisticated technology we see today. This journey from simple algorithms to cutting-edge technology marks a significant leap in innovation and progress.
In the beginning, AI was restricted to narrow tasks - systems designed for specific functions such as processing data. While these early AI systems were advanced for their time, they were restricted by their programming and could only handle a handful of tasks well. Fortunately, as technology advanced, so did AI.
The arrival of machine learning (ML) was a game-changer, letting systems learn from data and get better over time. This new era brought us predictive models that could make forecasts by spotting patterns and trends, taking AI beyond simple automation and into more exciting, dynamic territory.
The next breakthrough was deep learning, a twist on machine learning that uses neural networks with lots of layers to handle complex data. This breakthrough lets AI take on more advanced tasks, like understanding speech and translating languages, and with surprising accuracy.
The real game-changer, however, was generative AI. Building on the advances of ML and deep learning, it brought something new to the table: the ability to create fresh content from scratch. Using advanced algorithms like GANs and VAEs, GAI goes beyond just analyzing data.
How does generative AI work?
At its core, GAI uses advanced algorithms to learn and recreate patterns from large sets of data. It begins with neural networks - systems modeled after the human brain - with layers of interconnected nodes working in harmony to process information.
One key player in this creative process is the GAN. Imagine it as a creative showdown between two neural networks: the “generator” makes new content, while the “discriminator” checks it against real data. This competition helps the generator polish its creations, leading to ever more realistic and original outputs.
Another important tool is the variational autoencoder (VAE). These simplify data into a more abstract form and then reconstruct it, allowing them to produce new content that resembles the original but with a unique spin.
Generative AI also taps into reinforcement learning, where the system refines its output based on feedback from its environment. This helps the AI get better at creating content that meets specific goals.
In practice, generative AI works its magic by taking a prompt or set of guidelines - like a text description - and turning it into something fresh and new. It’s about igniting creativity and crafting something truly one-of-a-kind.
Core AI technologies
AI’s progress is driven by impressive technologies like ML, deep learning, and GANs. Let’s take a quick peek at how these powerhouses work their AI magic.
Machine learning (ML) and deep learning
ML is a core aspect of AI, providing machines with the ability to learn from data and adapt, rather than relying on predefined rules for every task. You can think of ML as a bookworm who improves their skills based on what they've studied. It’s all about recognizing patterns and making decisions or predictions. For example, ML enables spam filters to continuously improve their accuracy by learning from new email patterns and identifying unwanted messages more effectively.
Deep learning is a bit like ML’s upgrade. It uses neural networks with many layers. Inspired by the human brain, these networks are highly effective at recognizing intricate patterns in large volumes of data, automatically extracting key features without requiring much manual input.
Now, let’s dive into generative adversarial networks and see how they fit into the world of AI.
Generative adversarial networks (GANs)
Enter generative adversarial networks, the real show-stealers in the world of generative AI. Think of GANs as a creative duo with a bit of a competitive streak. They consist of two neural networks: the generator and the discriminator. The generator is like the artist, whipping up new content, while the discriminator is the critic, evaluating how well the new content stacks up against real data.
In this creative contest, the generator aims to create data so realistic that the discriminator can’t tell it apart from the real thing. It’s a bit like a friendly rivalry where the generator and discriminator push each other to improve. Over time, this back-and-forth helps the generator produce astonishingly realistic content, whether it’s generating new images, composing music, or even crafting text.
In simple terms, ML and deep learning work together, with ML recognizing patterns and deep learning handling complex tasks. GANs bring creativity, making AI not just smarter but also more innovative.
Now that we’ve shown how ML, deep learning, and GANs make AI smarter and more creative, it’s time to talk about the backbone of it all - training models. Think of them as the way AI gets its "education" allowing it to learn and grow over time.
Training models: What are they and how do they work?
To put it simply, training models are how we teach AI to recognize patterns and make decisions. Just like humans learn from experience, AI models are trained using large datasets to identify relationships and predict outcomes. During training, the model processes the data, identifies features, and adjusts its parameters to minimize errors. The aim? To improve with each training cycle, much like a student honing their skills with feedback on their assignments.
Training starts with feeding the model input data, which can be anything from images to text, depending on the task. The model makes predictions or generates results based on that data, and then we evaluate how well it did. If it gets things wrong (early errors are pretty common), adjustments are made. Over time, as it practices, the model becomes better at spotting patterns and delivering accurate results, much like becoming more adept at puzzles with practice.
This fine-tuning is a big deal because it helps the AI become more reliable and effective in real-world scenarios. Whether it's blocking spam emails, generating creative art, or forecasting the weather, well-trained models are the secret sauce to effective AI.
Having a grasp of training models sets the stage for us to dive into the crucial algorithms behind generative AI.
Key algorithms used in GAI
Now that we’ve nailed down how training models work, let’s turn our attention to the key algorithms that drive GAI. These algorithms are crucial for helping AI systems learn, adapt, and produce innovative results. We’ll explore the main techniques: supervised learning, unsupervised learning, and reinforcement learning.
Supervised vs unsupervised learning in GAI
When it comes to training models for GAI, supervised and unsupervised learning are two fundamental approaches, each with its strengths and applications.
In supervised learning, the model is trained on a labeled dataset, meaning each piece of data comes with a correct answer or outcome. For instance, if we’re teaching an AI to recognize cats in photos, it would be trained on a set of images labeled as “cat” or “not cat.” The model learns to map inputs to the right outputs by analyzing these examples. Over time, it becomes proficient at predicting the labels for new, unseen data. It’s crucial for tasks that need precise guidance, such as generating text from specific prompts or crafting images with specific details.
Meanwhile, you can think of unsupervised learning as the AI’s chance to explore on its own. Here, the model is given data without explicit labels or outcomes. The goal is for the AI to find patterns and structures within the data by itself. For instance, in clustering tasks, the AI might group similar items together without knowing what those groups should be. This method is useful for discovering hidden patterns or generating new content without predefined categories, making it ideal for creative tasks like generating novel music or art.
Reinforcement learning and its role in GAI
Reinforcement learning (RL) changes things up by teaching AI through trial and error. The AI acts like an agent exploring an environment, making decisions to achieve rewards.
If you’re wondering how it works, you can imagine teaching a robot to navigate a maze. The robot gets feedback through rewards (like reaching the end) or penalties (like hitting walls). With each attempt, it learns which actions get it closer to the goal.
Just like a robot learning to navigate a maze, reinforcement learning in GAI involves models exploring different approaches and receiving feedback on their success. This technique shines in scenarios that require making a series of decisions, like crafting interactive stories or fine-tuning creative projects in real-time.
To wrap it up, supervised learning, unsupervised learning, and reinforcement learning each contribute their strengths to generative AI. When used together, they help AI systems learn from data, detect patterns, and continually enhance their capabilities, driving creativity and progress.
Applications of generative AI across industries
Now that we’ve explored the nuts and bolts of generative AI (GAI) and its algorithms, it’s time to see how this revolutionary tech is making a splash across different fields. Whether unleashing new creative possibilities, revolutionizing business practices, or driving scientific breakthroughs, generative AI is making waves across the board.
Creative industries
Artists, designers, and musicians are leveraging AI to push the boundaries of their crafts. Picture AI crafting artwork, composing melodies, or designing fashion pieces tailored to the latest trends. Let's take DALL-E as an example. It can transform text descriptions into images, helping artists bring their concepts to life in no time.
At the same time, musicians can utilize AI to compose new melodies or mix tracks. The way AI generates art, music, and other creative content boosts the process and invites more people to explore their creative side like never before.
Business, commerce, and ecommerce
In the corporate landscape, generative AI is changing the game for how companies operate and build customer relationships. For ecommerce, AI-powered tools can personalize product recommendations, design marketing materials, and generate engaging product descriptions.
Generative AI-powered chatbots provide instant, human-like support. Though they might miss the mark occasionally, they’re still effective in handling routine queries, allowing human agents to tackle more immediate issues.
AI algorithms can also study market trends and consumer habits, giving businesses data-driven insights to make smarter decisions. While not perfect, they help shape strategies that connect with audiences. Whether it’s automating content or improving customer experiences, generative AI is proving to be a must-have in business.
Science and scientific research
In the sphere of science, generative AI is speeding up discoveries and fueling innovation. Researchers are leveraging AI to sift through massive datasets, simulate experiments, and propose new hypotheses.
For instance, in drug discovery, AI can predict how new compounds might interact with biological targets, which could speed up the creation of new treatments. In climate science, AI can create simulations of environmental changes, helping scientists predict future conditions. By automating data analysis and offering new insights, generative AI helps scientists solve complex problems and advance their research more swiftly.
In a nutshell, generative AI is reshaping industries far and wide - whether it’s crafting compelling art, supercharging business strategies, or fueling breakthroughs in science.
What are the everyday applications of GAI?
Generative AI isn’t just about sci-fi fantasies or high-tech labs - it’s weaving its way into our daily lives in fascinating ways.
Content with a personal touch
Imagine opening your favorite app and finding a playlist or news feed that seems perfectly tailored to your tastes. That’s what GAI can do for you. It personalizes your digital experience by analyzing your preferences and behaviors and providing recommendations for music, social media content, or news updates that match your interests.
When it comes to online shopping, GAI takes it to the next level by analyzing your shopping history and search patterns to suggest products you'll love. From finding the perfect t-shirt to discovering new gadgets, it makes your shopping experience smoother.
So next time you see that spot-on playlist or perfect product suggestion, you’ll know GAI is working behind the scenes to make your digital life more personalized and a bit more fun.
Virtual assistants and chatbots
Virtual assistants like Siri, Alexa, and Google Assistant use GAI to make our lives easier with voice-activated help. They set reminders, answer questions, and control smart home devices using advanced algorithms that understand and respond like humans.
In customer support, chatbots and virtual agents are stepping up their game. These AI-driven tools can handle everything from resetting passwords to processing orders in a flash. While they’re fantastic for quick fixes and freeing up human agents for more complex issues, they might sometimes miss the finer details of tricky problems.
Nevertheless, AI assistants and chatbots are always getting smarter and working better over time.
AI-driven creativity in arts
From eye-catching graphics to music, GAI tools are helping artists, designers, and musicians push creative boundaries. AI can create custom logos and personalized greeting cards by learning your style preferences.
However, it’s worth noting that AI-generated art can sometimes lack the finesse and depth of human-created pieces, as it relies on existing data and patterns.
Content generation for social media sites
For social media creators, GAI can be a game-changer. AI tools can generate captivating posts, suggest trending hashtags, and even edit your images or videos. This lets you focus more on connecting with your audience and less on content creation, helping you keep your online presence fresh.
Travel and navigation
Generative AI takes your travel experience up a notch by creating personalized itineraries, optimizing your routes, and suggesting top destinations. It can also help you discover the best times to visit and local must-sees, ensuring your travel plans are spot-on.
To sum up, generative AI is making everyday tasks and creative projects easier and sometimes even more fun. As the technology grows, we can expect to see more innovative ways AI will enhance our daily experiences.
Benefits and drawbacks of using GAI
Generative AI has made its way into many aspects of our lives, offering both exciting opportunities and some challenges. Let’s dive into the key benefits it brings, as well as the drawbacks to keep in mind when using this technology.
Benefits of GAI
GAI offers many benefits that are revolutionizing data handling and content creation. Its ability to generate and interpret complex data makes tasks like organizing datasets, converting satellite images into maps, and generating medical images much faster and more accurate.
This speeds up workflows and lowers the risk of errors, becoming important for industries driven by data.
On the creative front, GAI is changing how we approach content generation. Tools like ChatGPT for text and Dall-E or Canva for images allow users to produce original, high-quality work in no time. Media, marketing, and design professionals benefit from this, as it lets them create tailored content faster and offer more engaging, personalized experiences.
One major benefit is efficiency - GAI automates time-intensive tasks, saving resources and time. It also customizes content to better connect with audiences, increasing engagement and improving user satisfaction.
Drawbacks of GAI
While GAI offers numerous perks, there are a few things to watch out for. Privacy concerns can arise since GAI often relies on analyzing extensive personal data to generate recommendations. This raises questions about data collection, usage, and access.
Another point to consider is the risk of over-reliance on AI tools. Relying too much on AI for creativity and decision-making might hinder your creative thinking and problem-solving abilities. Thus, finding the right balance between AI help and your own input is critical.
GAI is far from flawless and may occasionally misjudge your requests or offer inaccurate recommendations. A dash of human oversight is often needed to fine-tune its results. Additionally, some AI systems come with a learning curve, making them a bit of a puzzle to figure out.
Future of generative AI: Trends and challenges
The road ahead for generative AI is brimming with possibilities, but it won’t be without obstacles.
One of the most interesting trends is the move toward multi-modal AI. Think of AI that can generate text, design images, and even engage in real-time conversations - almost like a human (well, some of us). This ability to handle diverse data will make AI tools far more inventive and useful in our daily lives.
Then there's the idea of interactive AI. Here, instead of just chatting with a bot, you could delegate entire projects to AI. Need an app built? Your AI could handle everything - from coding to hiring experts to marketing the final product. Or maybe you want to plan a weekend getaway? The AI could find a hotel, book your table at a restaurant, and even remember your food allergies. Who thought that the future could be that simple?
Of course, the power of generative AI needs to be handled with care. While its potential is incredible, there’s also the risk of misuse. That’s where ethical guidelines and regulations come in, ensuring AI serves humanity without causing harm. Balancing innovation with responsibility will be vital to making AI a positive force for the future.
Future-proofing our path
The evolution of generative AI shows no signs of slowing down, and its reach is sure to keep growing. With multi-modal systems and AI-driven collaboration on the horizon, the future promises remarkable advancements.
But as AI evolves, so does our responsibility to steer it wisely. Future-proofing our path means fostering innovation while keeping ethics and regulation front and center. By striking this balance, we can ensure that AI remains a powerful tool for positive change - enhancing our lives, work, and the world we live in.
Mirza Bahic is a freelance tech journalist and blogger from Sarajevo, Bosnia and Herzegovina. For the past four years, Mirza has been ghostwriting for a number of tech start-ups from various industries, including cloud, retail and B2B technology.