Meet the Nvidia GPU that makes ChatGPT come alive
This Nvidia GPU is the power behind ChatGPT
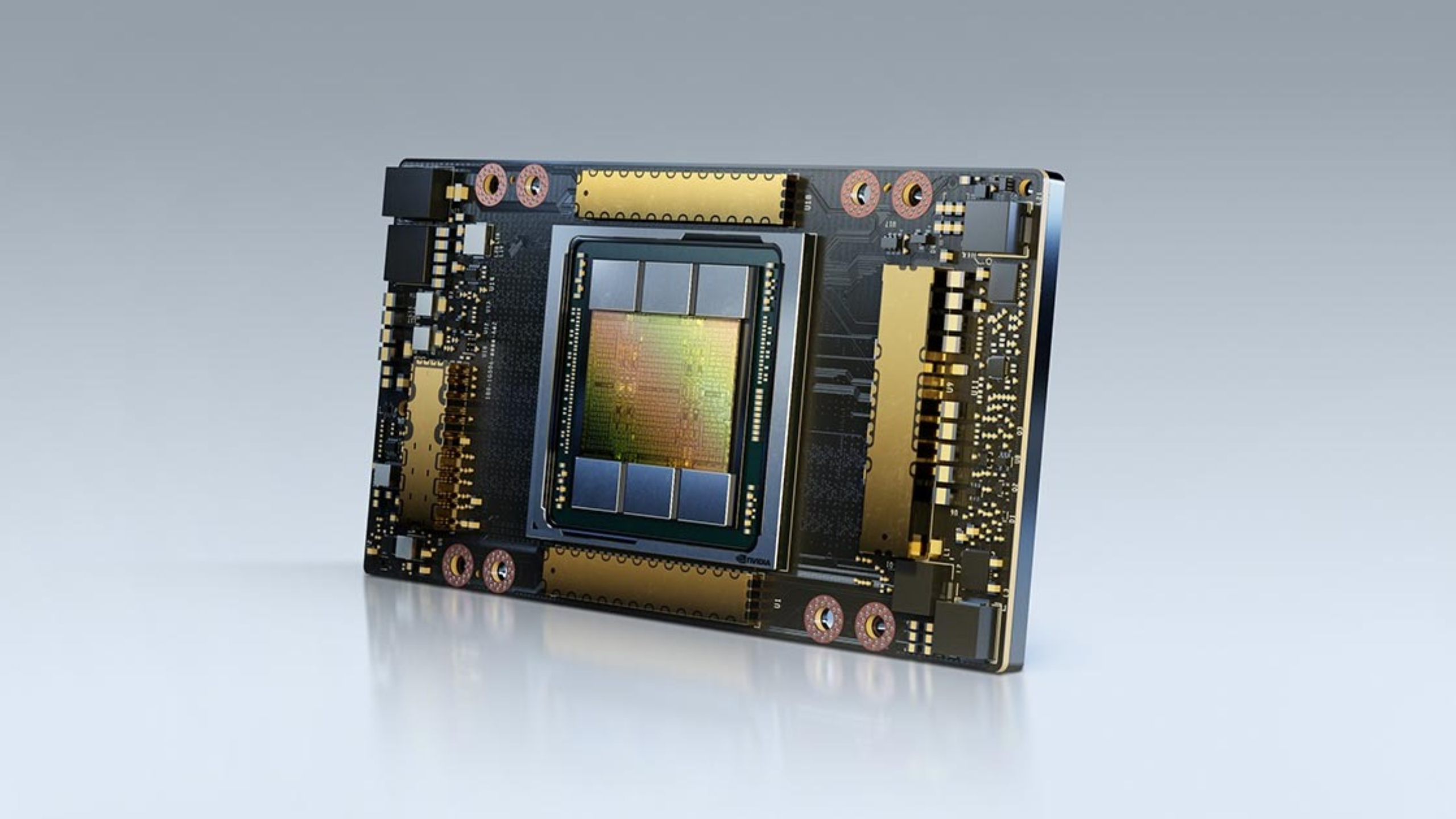
Behind the headlines of how ChatGPT is changing the world is a company that is mostly known for its graphics cards.
Nvidia is the biggest winner when it comes to hardware; its share price has almost doubled since mid-October 2022 as chatter around OpenAI and ChatGPT picked up; this fueled a massive growth in demand to access ChatGPT and in the specialized hardware that makes the technology tick and that doesn’t come cheap.
This partly explains why, with a market capitalization of $556 billion at the time of writing, the company that made Geforce a household name is more valuable than Arm, Intel, IBM and AMD put together.
What is the A100?
If a single piece of technology can be said to make ChatGPT work - it is the A100 HPC (high-performance computing) accelerator. This is a $12,500 tensor core GPU that features high performance, HBM2 memory (80GB of it) capable of delivering up to 2TBps memory bandwidth, enough to run very large models and datasets.
More impressively, it is passively cool - despite a 300W TDP - but requires two slots to accommodate the massive heat sink for the PCIe version. It is based on the 3-year-old Ampere microarchitecture, which is what powers the entire stack of Geforce GPUs in the 30 series (and the RTX 2050 - found in the Honor MagicBook 14 - and the MX570).
The A100 is commonly found in packs of eight, which is what Nvidia included in the DGX A100, its own universal AI system that had a sticker price of $199,000. Serveathome published some close ups of the A100 that feature a special form factor called SXM4. That allows for a much more compact setup, enabling system integrators to pack more of these per unit volume, as well as higher performance thanks to a higher TDP (400W for that model).
Get ready for ChatGPT 4.5 and beyond
We already know what will be coming after the A100 - the H100, based on the Hopper GPU architecture, which runs in parallel with its consumer alter ego, Ada Lovelace (which is what powers the RTX 4090). Launched in 2022, it uses a new form factor (SXM5), has more memory bandwidth available (3TBps) and is much faster than its predecessor. Nvidia says that it can train AI at up to 9x faster than an A100 or in other words, in specific workloads, it can replace nine A100 cards.
However all that comes at a cost; as the new GPU has a much higher TDP (700W) and will almost certainly require a bigger heatsink and potentially liquid cooling (what Serveathome calls rear door immersion direct to chip) in order to maintain stability and performance. There’s also the actual cost of the item: the NV-H10-80 (as it is also known) comes at a whopping $32,000 (ed: That didn’t stop Oracle or Microsoft from purchasing tens of thousands of them apparently).
Instances of the HGX-H100 - which replaces the HGX-A100 and will be the hardware foundation of future generative pretrained transformers - should be available from CoreWeave and Lambdalabs from March 2023, starting according to the former, from as little as $2.23 per hour when reserving capacity in advance, as a bare metal service. Alternatively, you may want to get your own bespoke, customized H100 system from specialist system integrators such as Silicon Mechanics, Microway or Dell from under $300,000.
Nvidia’s ridiculous head start in accelerators has left its rivals, AMD and Intel, far behind, allowing the US company to look ahead to the day when it will become the first trillion dollar semiconductor company. Things will get even more interesting when Grace, its Arm-based Superchip, and Hopper, its GPU behemoth, join forces together.
Are you a pro? Subscribe to our newsletter
Sign up to the TechRadar Pro newsletter to get all the top news, opinion, features and guidance your business needs to succeed!
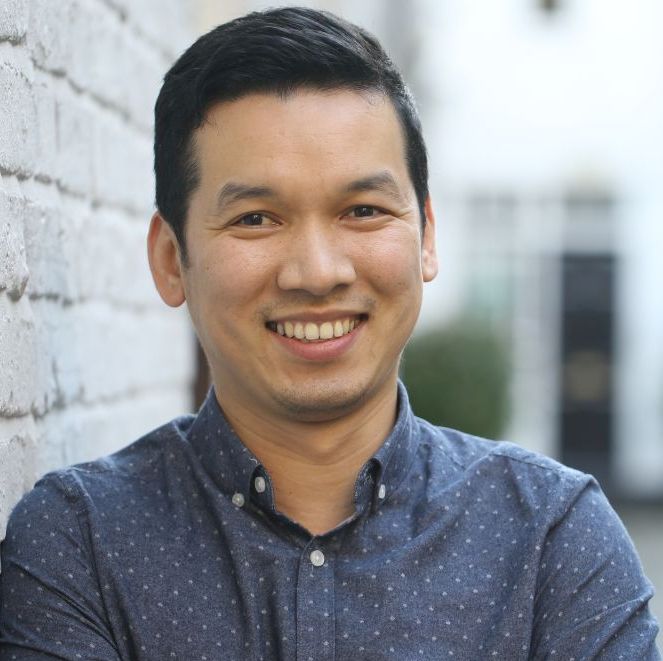
Désiré has been musing and writing about technology during a career spanning four decades. He dabbled in website builders and web hosting when DHTML and frames were in vogue and started narrating about the impact of technology on society just before the start of the Y2K hysteria at the turn of the last millennium.