Earth to AI: why semantic analysis Is critical for AI tools to understand human data
Semantic analysis is essential for organizations in all sectors
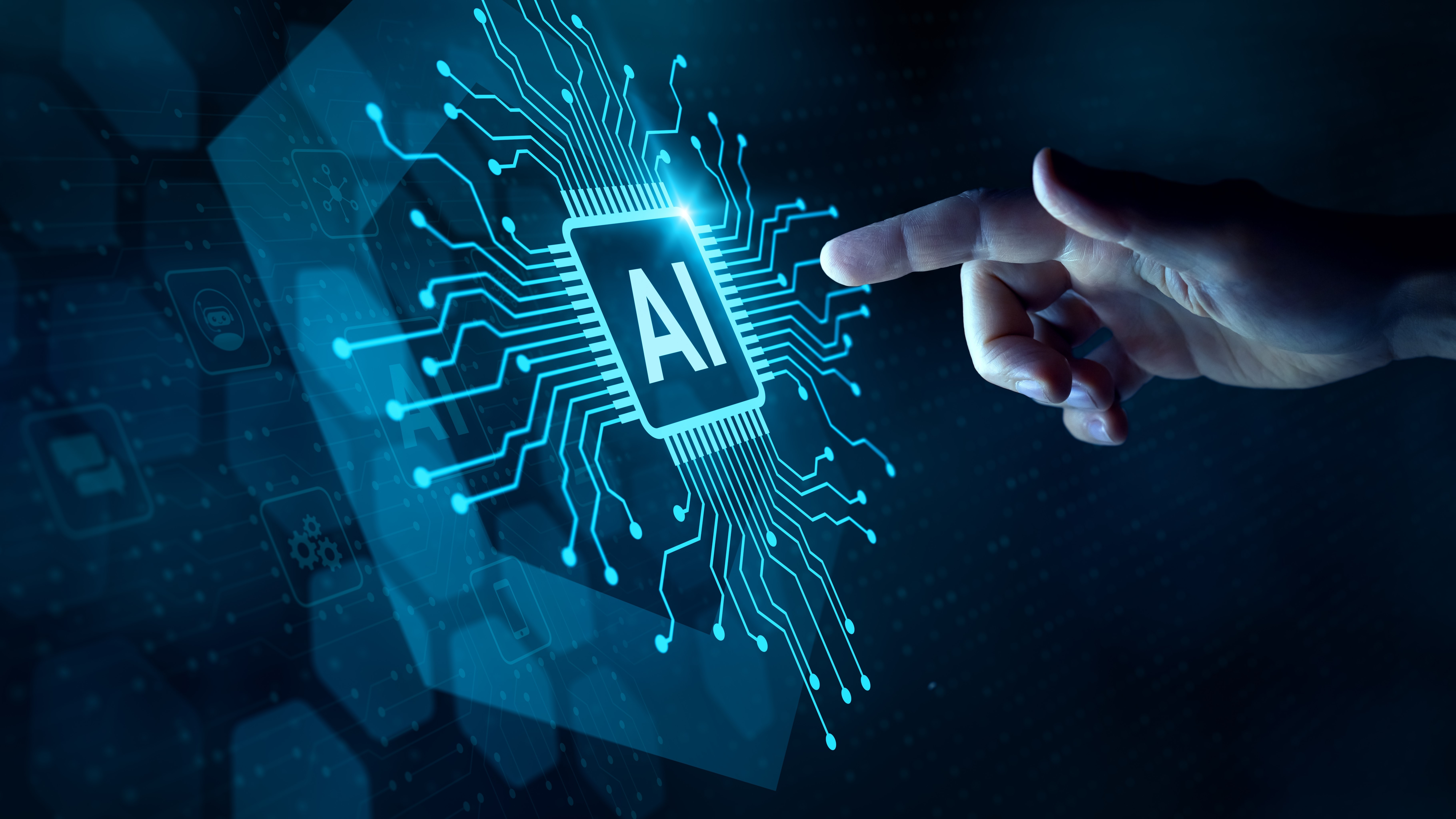
With the vast amount of data that enterprises are generating and storing, leaders are continually reminded to get their data in order so they can generate business insight and prepare for AI initiatives. However, there is a reason why a majority of the enterprise’s human intelligence cannot be utilized to inform the business decisions being made today.
The main issue is that computers are unable to determine the meaning and context of unstructured text—and there is simply too much of it for humans to process. With the global market for AI technologies expected to grow to over 1.8 trillion U.S. dollars by 2030, there is a clear business need for computers to understand the human language, or business leaders will fail to make the most of their AI investments.
However, there is a solution. In using semantic analysis, which has automated and assisted text classification and fact extraction processes, a business can use AI to understand the meaning of its enterprise data with accurate, consistent and transparent outcomes. With this meaning, they are able to unlock valuable insights, enhance user experience and drive smarter decisions.
SVP for Product and Engineering - Application and Data Platform at Progress.
The meaning of semantic analysis
Semantic analysis builds upon natural language processing (NLP) and focuses on understanding and processing the meaning of words and phrases that have multiple meanings. It works by using methods and tools that can efficiently categorize and process data, whilst seeing relationships within data sets. In so doing, computers can finally comprehend the human intent behind words, phrases and sentences.
Semantic analysis can answer questions like: “What does this text mean?”, and “How do these concepts relate to each another?” It also can extract specific information in the data like the age of a patient or the directors of a company. By revealing and encoding what the text really means, semantic analysis enables the most accurate information retrieval and improves communication between machines and humans. Put simply, it can understand business data to generate meaningful insights.
By interpreting communications with customers and gaining insights into habits and preferences, semantic analysis can enable businesses to power chatbots and deliver a new level of agility to the organization. By deriving new insight from communications with customers—helping train chatbots, for example—Semantic analysis can add a new level of agility to the organization.
Getting deeper meaning with text classification and fact extraction
Depending on what an organization is looking to achieve, semantic analysis can use two methods to extract information from text. These methods are text classification and fact extraction.
Text Classification
With this method, text is labelled based on insights you want to gain from the textual data. For example, Topic Classification can organize text within a particular category, for instance putting a school assignment into math, chemistry or physics. Intent Classification assesses the sender’s intent for a message, which can help the organization respond in the best way.
Fact Extraction
With Fact Extraction, organizations can identify and extract facts and relationships in their content to aid in the discovery of latent patterns and knowledge not previously known to their business. If organizations can make information easier to find within their content, it brings efficiency to the organization as it can reuse text for various use cases and audit it.
The Text Re-use feature can help leverage content in different contexts quickly and for different use cases. Text Auditing helps you stay ahead of your competition and fully access the volume of knowledge in your content. Text Creation allows teams to focus resources on creating missing content that fills gaps rather than recreating content that already exists.
Examples of semantic analysis
Here are six ways semantic analysis can add value and agility to an organization:
Intelligent search experience - It can help search engines understand the meaning of text to position it appropriately in results. It goes beyond repetition, improving the results through the richness of the semantic field. This results in an intelligent search experience that can understand user intent and context for better outcomes.
Customer feedback – Since it can understand the positive, negative or neutral context, semantic analysis can help machines better understand customer feedback from social networks, reviews and forums by detecting elements that denote dissatisfaction, discontent or impatience. Trends insight – Semantic analysis can help marketers get closer to their customers and keep on top of market trends by processing large volumes of textual data.
Customer experience – The overall elevated digital experience helps customers to find more relevant information faster and offsets problems before they can escalate.
Regulatory compliance - Semantic analysis can help an organization meet regulatory needs, mitigate risk and streamline processes. It helps apply metadata that organizations can use to implement information governance best practices.
Making your data AI ready – data agility is vital for successful AI applications. Semantic data analysis allows for efficient data integration, classification and contextualization, which will enable organizations to build more adaptable and responsive AI systems.
Benefits of semantic analysis
A well-executed semantic analysis methodology will bolster competitive advantage by elevating the customer experience and company performance, improving search relevance and providing valuable insights for new business initiatives. Some key advantages of semantic analysis include:
Build a customer-centric strategy: Semantic analysis contributes to the deployment of a customer-centric strategy, where each business decision is centered on the customer’s needs, boosting customer loyalty and retention.
Improve company performance: By automating data analysis, semantic analysis offers considerable time savings for teams across the organization, channeling resources to prioritize business-critical functions and improve the bottom line.
Enhance your SEO strategy: Semantic analysis can help uncover a user’s intent during the search process, enabling the organization to serve up more relevant content.
Bolster customer self-service solutions: Semantic analysis allows machines to detect human emotions to extract valuable information from unstructured data so more effective self-service options can be created. Support AI Strategy: When it comes to machine learning and AI, semantic analysis supports feature extraction and understanding relationships in data.
Smarter business decisions: Ultimately, semantic analysis extracts the meaningful information companies need to drive informed business decisions. Enable 360s: By providing a comprehensive view of all data, semantic analysis allows organizations to aggregate information effectively, enabling them to conduct more informed 360 reviews of products.
The value of data Is achieved through its analysis
With the advancement of AI and the rise of unstructured data, semantic analysis is essential for organizations in all sectors who are looking to embrace AI agents and data driven decisioning.
The introduction of semantic analysis marks a significant leap forward for machines to become not only viable but trusted in the business landscape. By harnessing the power of meaning, businesses can unlock valuable insights, enhance user experience and drive smarter decisions.
We've featured the best productivity tool.
This article was produced as part of TechRadarPro's Expert Insights channel where we feature the best and brightest minds in the technology industry today. The views expressed here are those of the author and are not necessarily those of TechRadarPro or Future plc. If you are interested in contributing find out more here: https://www.techradar.com/news/submit-your-story-to-techradar-pro
Sign up to the TechRadar Pro newsletter to get all the top news, opinion, features and guidance your business needs to succeed!
Matthieu Jonglez is SVP for Product and Engineering - Application and Data Platform at Progress.
You must confirm your public display name before commenting
Please logout and then login again, you will then be prompted to enter your display name.