Don’t dive into GenAI waters without first learning to swim
The foundations for GenAI implementation
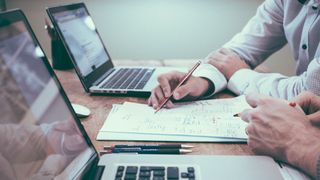
It’s now been over a year since Generative Artificial Intelligence (GenAI) exploded onto the scene and made its way into apps and headlines around the world, and business leaders today are starting to realize that GenAI interest was not just driven by ‘hype’, and instead could be truly transformative for almost any sector.
Companies are scrambling to stay ahead of the competition – often by adopting the latest AI tools on the market. And with the speed of development accelerating at an unprecedented rate, the fear of being ‘left behind’ is creeping through boardrooms. This rush is leading to some unusual patterns, with a recent study from MIT IDE showing high levels of AI adoption in the US Midwest and South, outside the typical coastal tech hubs.
So, what’s the issue?
Typically, well-established companies are less amenable to change or not agile enough to adopt the latest technology as soon as it’s available. As a result, many companies — especially large enterprises — are tied to legacy software due to a combination of outdated procurement processes, familiarity, or concerns about data loss or disruption, making modernization inaccessible.
This creates a dangerous situation for CTOs and CIOs. While they are being pressured by their boards to adopt a plethora of the latest GenAI tools, the foundations for a successful AI project - namely an agile IT infrastructure, suitable internal skill sets and a realistic transformation plan - are often not in place. Without these necessary elements there are genuine and very severe business risks: namely around bias, data governance and transparency.
Already we’ve seen high-profile cases such as the ‘Savey Meal-Bot’ in New Zealand, where an AI chatbot, trained on ‘bad data’, recommended recipes like a “bleach-infused rice surprise” as a “surprising culinary adventure” to users.
Enterprise Architect, SnapLogic.
Particularly recently, with the UK's Post Office scandal emphasizing on a national scale the very real consequences of a poorly-managed legacy modernization project, it’s become vital for IT decision makers to step back and ensure that all the necessary elements are in place for the successful implementation of GenAI.
Unfortunately, many businesses lack these core elements. According to one Gartner analyst, this has meant that AI projects could have a failure rate of up to 85%. While this number appears shocking, the issue here is not the AI tools themselves, it is an issue of planning and preparation.
Are you a pro? Subscribe to our newsletter
Sign up to the TechRadar Pro newsletter to get all the top news, opinion, features and guidance your business needs to succeed!
Crossing your As and dotting your Is
Businesses today must get their house in order before they implement AI. Large enterprises have the data that is needed to be successful with GenAI projects, but success requires harnessing the data, not just having it in a data lake.
To do this, firstly, the GenAI tools they implement must be able to pull the accurate data they need to service a prompt. For instance, if I asked my GenAI tool to analyze my sales figures in the last year – does it easily have access to this data, is it connected to my analytics tool, and most importantly, am I sure that the original data is accurate?
GenAI can only work on the data it is fed, so duplicated data, inaccuracies and inconsistencies can all drastically reduce the effectiveness of the tools, and stop AI-driven digital transformation in its tracks. Even when these problems are addressed and data is in good shape, if it isn’t readily available and accessible, it is ultimately not usable for an AI tool.
An integration platform will be crucial here, connecting data across an organization and creating one cohesive infrastructure which GenAI tools can utilize. These platforms can also help establish good data governance frameworks to manage, store and secure business data and even have the capabilities to normalize, clean and deduplicate data across the business.
Good integration platforms can also knit together legacy and modern systems, whether in on-premise or cloud environments, and even cross barriers between applications and data. This seamless integration makes AI-driven digital transformation projects far easier, and the tools themselves much more effective.
Next, look to AI platforms that maximize efficacy whilst minimizing the skill demands on IT teams. For instance, no-code GenAI development products can allow businesses to integrate AI with enterprise data without the need to involve data scientists or coding experts at every step. These products put the power of large-language models (LLMs), and the scale of AI, where it matters most: in the hands of the employees who are closest to the business and understand what is required for success.
With these tools, something that would have been incredibly complex, like developing customer support automation, can be undertaken directly by business users without any coding knowledge at all. This approach allows anyone to leverage the power of AI to automate tedious repetitive portions of their work, regardless of technical expertise.
Patience is a virtue
Finally, consider the expectations and timeframe of a GenAI transformation. While GenAI does have massive potential, it is not a miracle solution for all business problems, and as such, organizations need to set clear and realistic goals for their AI implementation, with the understanding that these tools will develop and improve over time.
For instance: currently, there is no set rule book for achieving great results with GenAI LLMs; there are tips and tricks you can deploy for better or faster results, of course, but overall the process is largely driven by trial and error. Interacting with GenAI in its current iteration is like a science experiment – you come up with a hypothesis and continue to test different manners of prompts until it produces the result you’re looking for.
There is one danger that is unique to AI projects. The nature of this technology makes it very easy to come up with a compelling demo — but because of that very ease, there is a far greater than usual gap between pilot and full-scale production implementation. A big part of that gap is due to the difficulty of coming up with a full-scale, complete, and high-quality data set to feed the model. Without the data, the GenAI project will be unable to deliver the hoped-for business impact.
Companies that want to apply GenAI to their products will need to think about how they carry forward and evolve prompts that can improve results directly. The key is to put a plan in place to evaluate the responses from the model, and to use that data to inform prompts further.
However, qualitative and quantitative improvements can only be brought about by reevaluating the approach to AI application and development. In other words, businesses need to develop an AI-centric mindset, not only planning how they will adopt and prepare for AI tools, but also how they will learn from and with them.
Preparing today for success tomorrow
GenAI will undoubtedly have massive benefits and implications for businesses, customers, and employees. To make the most out of these tools, organizations must reassess their thinking around Artificial Intelligence. Like any digital transformation initiative, there must be a clear plan and realistic objectives, but unlike a ‘normal’ digital transformation project, there are additional steps, expectations and structures to consider. Success in this new domain requires a different, AI-centric mindset.
Without these core foundations, AI transformation initiatives will likely fail to deliver on expectations. However, with all these elements in place, the potential of GenAI can truly be realized.
We've featured the best business intelligence platforms.
This article was produced as part of TechRadarPro's Expert Insights channel where we feature the best and brightest minds in the technology industry today. The views expressed here are those of the author and are not necessarily those of TechRadarPro or Future plc. If you are interested in contributing find out more here: https://www.techradar.com/news/submit-your-story-to-techradar-pro
Dominic Wellington, Enterprise Architect, SnapLogic.
No 'independent chip makers and system makers and storage makers': Industry expert paints bleakest picture of future of IT — insatiable tech appetite of Google, Microsoft and other hyperscalers could kill off competition forever
'Inspired by the human brain': Intel debuts neuromorphic system that aims to mimic grey matter with a clear aim — making the machine exponentially faster and much more power efficient, just like us