Ushering in the third wave of AI
Breaking down the language barrier between AI and humans
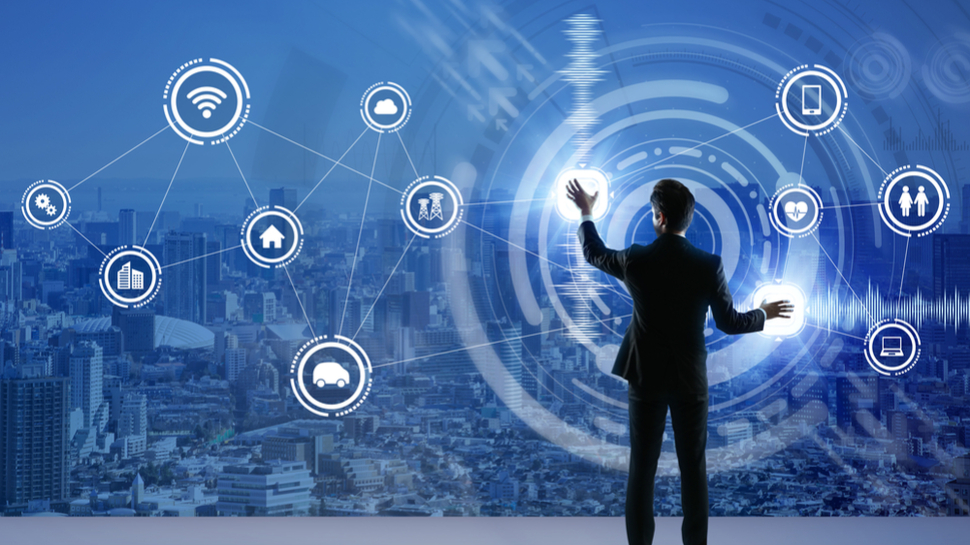
Today, artificial Intelligence (AI) helps you shop, provides suggestions on what music to listen to and what shows to watch, connects you with friends on social media and even drives your car.
Tolga Kurtoglu, Head of Global Research at Xerox.
As more companies focus their efforts on AI-based solutions, 2020 is shaping up to be a turning point as we begin to witness the third wave of AI — when AI systems not only not learn and reason as they encounter new tasks and situations, but have the ability to explain their decision making.
Where We Are Now
The first wave of AI focused on enabling reasoning over narrowly defined problems, but lacked any learning capability and poorly handled uncertainty. Financial products like Turbotax and Quickbooks, for example, are able to take information from a situation where rules have previously been defined and work through it to achieve a desired outcome. However, they are unable to operate beyond the previously defined rules.
The second wave, which we are in the midst of right now, is AI that has nuanced classification and prediction capabilities, but no contextual capability and minimal reasoning capability. Major machine learning-based AI platforms like IBM’s Watson and Salesforce’s Einstein are good examples as they are able to synthesize large amounts of data to provide insight and answers, but are not able to truly understand or explain how they got to that answer.
The Third Wave: AI That Understands and Reasons in Context
So how do we progress from the second wave to the third wave?
The starting point is to make AI explainable, more transparent. AI algorithms will continue to increasingly interact with humans across many industries — in our homes, our cars, and our clothing. If they continue to evolve the way they have been, and they are black boxes in nature, there is a potential concern with transparency and eventually with trust. To avoid these concerns, these systems should be transparent in a way that they can explain their work, including the assumptions they made, the different options they considered and eventually why they came up with the answer that they provided.
Right now, there is an opportunity to establish trust between the system and the human. Explainability and transparency are a starting point to something much broader—the collaboration between humans and computers to solve the most complex problems in the world.
Sign up to the TechRadar Pro newsletter to get all the top news, opinion, features and guidance your business needs to succeed!
Partnering with AI to Solve Intractable Problems
Transparency leads to trust. Once you have trust, you can begin thinking about how we become effective partners with AI agents as humans, and how to work in a truly collaborative fashion to solve some of the most intractable problems.
As AI becomes more involved in making critical decisions alongside humans, it is important for AI researchers to consider how these decisions are made in real time, sometimes under duress. For example, if someone is about to get into a car accident, the driver and AI need to make decisions under tough circumstances together. Just like two humans, the human and the AI system need to be able to understand each other and trust each other. Enabling translation between how humans represent information and how machines represent information will help facilitate this collaboration and ultimately enable AI to explain itself.
To help accelerate the third wave of AI, the Defense Advanced Research Projects Agency (DARPA) announced last year that it would be investing more than $2 billion in its AI Next campaign. As its research with universities and private companies progresses, we should start to see this program make strides toward its objective of transforming computers from specialized tools to partners in problem-solving.
Widespread Adoption of AI Will Require a Hybrid Approach
Today, most AI is solely based on machine learning. The main problem with this approach to AI is that it requires thousands, if not millions, of data examples to function properly. As we enter the third wave of AI, in order to enable widespread adoption, AI systems need to shift away from this data-heavy approach.
Rather than continuing to focus on models that require massive amounts of data, the way that we will truly see widespread adoption of AI is to focus on methods that use a combination of approaches, including systems modeling and human-machine collaboration.
This hybrid approach will give organizations and individuals the ability to reap the benefits of modern techniques with only a fraction of the data that is required for large machine learning platforms and help solve problems for which large datasets do not exist.
Looking ahead to 2020 and beyond, we are at a critical inflection point in the progress and development of AI systems. This exciting third wave of artificial intelligence holds the promise of radically shifting the way humans perceive AI agents as a trusted partner in solving some of the world’s most complex challenges.
- We've featured the best in cloud computing services.
Tolga Kurtoglu is the Head of Global Research at Xerox.
He is a business executive with strong experience in corporate innovation, R&D, and technology commercialization.
Tolga served in various leadership roles focusing on R&D management, product strategy, strategic alliances and partnerships, and technology transfer.