How AI can create limitless boundaries for location intelligence
Location-aware AI creates opportunities
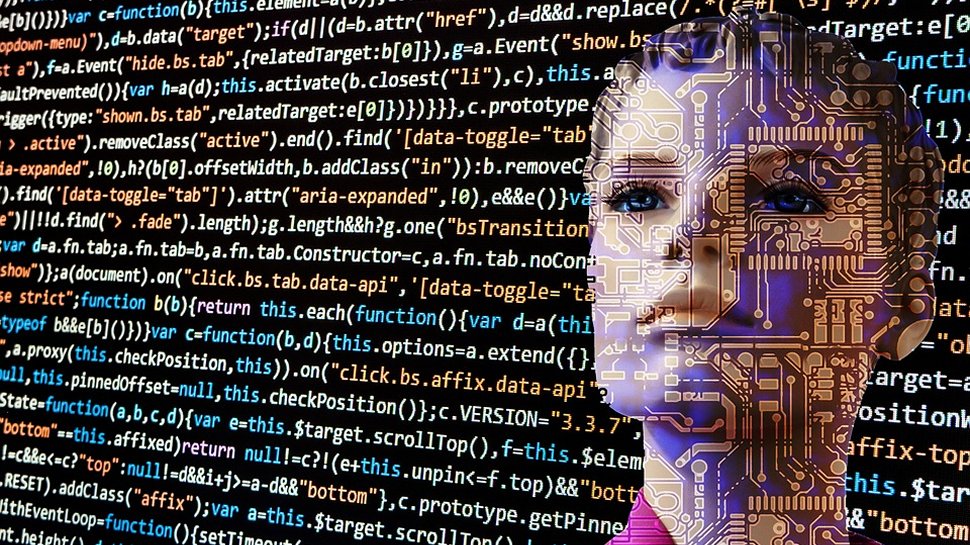
It is no secret that the growth of IoT is fueling a data explosion, with the International Data Corporation forecasting that by 2025 there will be over 41 billion connected IoT devices generating just under 80 zettabytes of data. This unprecedented influx of data, coupled with developments in AI to extract insights from it, will forge a mobility industry that is vastly better informed than before.
AI is typically deployed to automate the creation of maps using location intelligence data, creating an opportunity for businesses to enhance the insights they are getting by using truly location-aware AI. This means AI that is able to understand the properties of location information and allows developers to leverage these location insights into their applications and products.
Location data-infused AI is used to create pattern recognition and location signatures from the data it collects, which can support in generating HD maps and realistic simulators to visualize this data. From understanding how consumers move, to knowing where is best to drill for oil, these intelligent visualizations are creating an exponential amount of use cases across a multitude of industries.
The value in building reliable AI-based location intelligence services
The AI value chain has dramatically shifted over the past few years. We are moving from traditional machine learning (ML), where the value is mostly around solution model architecture and algorithms, to a phase where the value lies in having a benchmarked model working on your data and being able to evolve, compose and scale it.
We have become used to the standardized deployments of AI and ML for gaining location intelligence, and it is now commonplace for sensors, aerials or satellites to be used to produce standard definition maps, such as those people have on their smartphones. Increasingly we are moving towards high-definition (HD) maps, which are built by machines to serve machines, which make it possible to deploy this data for specific use cases, such as building algorithmic solutions for autonomous driving. This new approach makes it possible to conflate multiple sources to recognize features and patterns, dealing with both static and real-time events to predict behaviors and conditions.
A typical example of this is AI/ML powered maps in which the end-to-end process creates a self-healing map that relies on continuously gathering ‘low-level’ observations and ‘high-level’ aggregated map features, which are both automatically learnt features. These work in unison to evolve and adapt each map feature, such as signs, lanes, pavements and are learnt for each geographic region. Where this falls short, however, is in its rigidity, as it negates the nuances around the collection of the data itself, which is why location data platforms are innovating to create location aware AI.
AI making predictions possible
Put simply, this is AI that is designed to understand the dependencies and properties of the location information it receives and generate more advanced insights. Real time semantic relationships across physical objects are a key element of this and can be used to build location graphs, which are geospatio-temporal representations of the world.
Are you a pro? Subscribe to our newsletter
Sign up to the TechRadar Pro newsletter to get all the top news, opinion, features and guidance your business needs to succeed!
These representations can be used to make informed predictions through the use of real time data, such as weather, traffic or sensor data. AI/ML will facilitate the understanding of location and movement in a contextual manner and this will give us fundamentally new approaches that change how location information is gathered, represented, and utilized.
Location data-infused AI can discover new data patterns and generate more accurate samples of the data it collects. This means it can be used to expose key features and be composed with other data in ways that are unachievable with traditional AI approaches, such as using location AI to predict the correlation of NO2 concentration using traffic data.
Benefitting the transport and logistics industry
Data collected through AI can be used to inform and help make decisions for a specific problem, as is seen in its use in the transport and logistics industry. The crucial challenge facing this industry is the need to solve the problem of large-scale optimization. Leveraging vast amounts of data in large supply chain networks creates a many-to-many optimization problem for data providers due to the sheer volume of providers, consumers and modalities involved. While inroads have been made in this space, there is room for development through the use of location-aware AI. This technology is increasingly being embedded throughout supply chain networks, proliferating the number of interfaces for tracking and monitoring.
Reinforcement learning (RL) has the potential to be a quantum leap in the transport and logistics industry. This technology enables simulation and sensitivity analysis which can be used to create predictive models and simulations. RL can help us find new ways of controlling fleets which would allow for more optimal fleet traffic. An example of its use could be for planning and managing traffic flow in large smart cities with the ambition of minimizing CO2 emissions, while also guaranteeing maximal safe and efficient mobility. The insights learned from these models enable data-informed decision-making and results for an efficient distribution network.
Significant margin for growth
The potential for location-aware AI is significant, and no one can claim to have fully utilized its capabilities in the transport and logistics, automotive or smart cities spaces. The greatest advances in AI/ML may not happen in closed laboratories but only through a powerful set of open ecosystems and open innovation.
Collaboration between location platforms and academic or government institutions can play a key part in this, as it becomes possible to leverage AI in an effort to advance many use cases for a ‘smarter planet’. Location platforms can deploy smart city initiatives related to public safety, or provide prime insights to companies in the automotive and mobility sectors. These insights can be considered as a new currency within the mobility space and used to make important strides to build reliable and key real time AI-based location intelligence services. These services, in turn, will enhance products across cloud, edge and device deployments for years to come.
- Giovanni Lanfranchi, CTO and SVP Development at HERE Technologies.
- We've featured the best business intelligence tools.
Giovanni Lanfranchi, CTO and SVP Development at HERE Technologies.