If you want to run AI training and inference on almost any GPU, then this startup may be the answer
Akash augments existing IT infrastructure to optimize it for the AI age
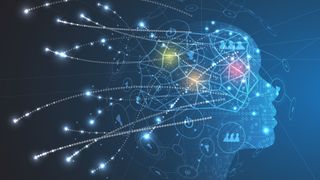
With the usage of AI in enterprises across the world continuing to boom, many organizations are hoping to get in on the action. But the technical, resource, and financial barriers to running a generative AI system like a large language model (LLM), let alone training one, remain prohibitively high for many companies.
The vast majority of businesses getting involved in training and managing generative AI models, however, will need to tap into the power of the best GPUs — and effectively replace their IT stack with graphics cards optimized for AI. Currently, Nvidia is the industry leader and manufactures several high-end GPUs. The Nvidia A100, for example, powers ChatGPT – while the follow-up GPUs including the H100 and H200 chips can run workloads even faster and more efficiently.
While other companies like IBM and AMD are also attempting to engineer components that can do the job, these aren't quite at that level. The catch, however, is there's a GPU shortage given the rapidly expanding demand in recent months.
That's where cloud startup Akash is stepping in. In September 2023, the firm launched its Superlcoud for AI platform – which lets businesses tap into a GPU network remotely to run AI workloads, while others can also contribute GPUs from their servers for businesses to lease. But it also incorporates all kinds of GPUs – not just those high-end Nvidia monsters that are pretty hard to come by.
Akash CEO and founder Greg Osuri spoke to TechRadar Pro to discuss how we could, as a result, see a democratization of AI should this idea take off.
The one thing that piqued my interest about Akash is the fact that it allows GPUs of any ilk to be used for GAI (General Artificial Intelligence). Why haven’t others done this before?
The world’s biggest cloud providers are indeed struggling to keep up with the demand for access to GPUs, which is driving up costs and pushing some small players out. This undermines innovation, especially since GPUs used for training are hard to come by if companies aren’t buying large quantities.
Building a decentralized cloud computing platform like AkashML is a technically complex endeavor. Akash’s software is a dashboard that enables a standardized control layer to manage a heterogeneous and distributed network of computational (GPUs and more) resources. The fact that we’ve been a permissionless, bootstrapped network since day one and are community-driven is a big part of how we achieve all this; the community helps us constantly improve our technology and expands the team’s ability to build faster. We’ve been able to manage supply and demand, as it’s difficult to aggregate a large enough number of lower-end GPUs to be meaningful enough for heavy AI workloads, which is why we’ve prioritized high-powered, high-density GPUs like A100s. This has helped us overcome cold-start challenges, unlike others in this space.
For the AkashML network, we battle-tested our GPU capability over a three-stage testing period before our recent Mainnet, which enabled us to incorporate user feedback in near real-time. We saw significant adoption with 1,300+ participants and a total of 3,100+ deployments.
Unlike other offerings that force businesses to swap their existing infrastructure or are only optimized for short-running batch jobs, we augment existing IT stacks and offer developers more control over where their application runs.
Two examples of how our network has been successful include:
- We’ve begun the large-scale effort to re-train a pixart-alpha model with a CC0 data set––marking the first time this has been done on a decentralized platform––helping to address copyright concerns, in partnership with genAI platform Thumper.ai. This serves as a way to further pressure-test the Akash Network, by providing a cluster of 32 Nvidia A100s (80GB) via a single provider to Thumper.ai to code, train, and open source the model. This experiment will showcase that big, heavy production workloads in AI can be done on Akash.
- We’ve also deployed SDXL (Stable Diffusion XL 1.0) on NVIDIA A100s, RTX-A6000s, and RTX-3090s, to empower developers to achieve high-performing and cost-effective inference of 1024×1024 images. This proves that SDXL is capable of running on a decentralized computation network.
How does it work when the concept (which is not different for neophytes from say cryptomining, torrent seeding, or distributed computing - SETI) seems to be at odds with traditional AI setups (data centers, local GPU, etc.)?
We rely on a decentralized network of providers who contribute their idle computing resources, such as CPUs, GPUs, and memory, to our network. Users can access the Akash marketplace to access these resources. As an example, NVIDIA H100s are available for as low as $1.10/hour. The network seamlessly coordinates the process of resource allocation, pricing, and payments.
The ultimate goal of Akash is to provide open access to GPUs, giving power back to developers so that one day, people will leverage the Tesla in their garage, their Xbox or PS5, on Akash to help accelerate AI workloads. We’re laser-focused on unlocking more cost-effective, scalable, and widely accessible AI capabilities compared to traditional, centralized data center setups.
Beyond AI training (Compute), what other categories is Akash looking at?
Akash Network is a general-purpose computational network and operates like the next evolution of the cloud — a Supercloud. Akash already supports validator nodes and frontend hosting, and will soon easily enable video encoding (which requires intensive CPU/GPU resources) and visual asset rendering/modelling.
Akash can run all of the same applications as traditional public clouds. This is why Akash is often referred to as a public utility. One that keeps costs down for businesses and consumers alike
Does Akash plan to work (or indeed works already) on onboard GPU, which is what the vast majority of computers (and smartphones) have?
Akash currently supports all NVIDIA GPU models and is working to add support for AMD chips in the near future. Onboard GPUs on smartphones and home computers leverage a different style of chip and are not optimized for AI/ML training––meaning that they are less powerful and capable when compared to even a single cluster of high-end GPUs, though this is something that Akash is looking to explore down the line. Right now, the team is focused on prioritizing support for high-performance data center-grade GPUs (with high-performance consumer-grade GPUs second) as those are the highest in demand for developers training their AI deployments.
More from TechRadar Pro
- These are the best GPUs out there
- Meet the Nvidia GPU that makes ChatGPT come alive
- Amazon wants to train millions of people in basic AI skills
Are you a pro? Subscribe to our newsletter
Sign up to the TechRadar Pro newsletter to get all the top news, opinion, features and guidance your business needs to succeed!
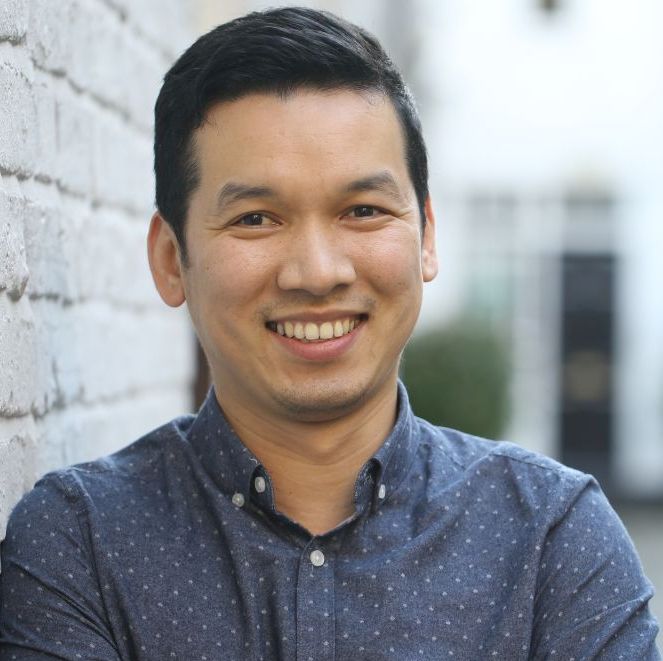
Désiré has been musing and writing about technology during a career spanning four decades. He dabbled in website builders and web hosting when DHTML and frames were in vogue and started narrating about the impact of technology on society just before the start of the Y2K hysteria at the turn of the last millennium.
- Keumars Afifi-SabetChannel Editor (Technology), Live Science
This is the tablet with the biggest battery ever — small-time Chinese firm promises rugged device with a staggering 33,280mAh battery, three times the capacity of Apple's iPad Pro, and much cheaper as well
Apple’s third-party Safari integrations rolled out with “catastrophic security and privacy flaws”