The evolution of speech recognition technology
From science fiction to a useful tool for businesses and consumers alike
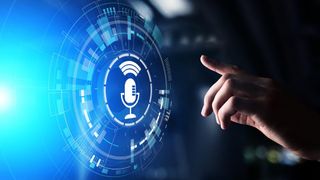
Do you remember when the idea of KITT, the chatty Knight Rider car, still blew you away? Or when Blade Runner Rick Deckard verbally commanded his computer to enhance photos of a crime scene? The idea of being understood by a computer seemed futuristic enough, let alone one that could answer your questions and understand your commands.
Graeme John Cole is a contributor for Rev, creator of the world's most accurate automatic speech recognition engine, Rev.ai.
Today, we all carry KITT in our pockets. We sigh when KITT answers the phone at the bank. The personality isn’t quite there yet – but computers can recognize the words we say near-perfectly.
Michael Knight, the Knight Rider hero who partnered with his intelligent car to fight crime, was skeptical at the thought KITT might understand his questions in 1982. But the development of speech recognition technology had been underway since the 1950s. Here's a closer look at how that technology has evolved over the years. And how our ways of using speech recognition and speech-to-text capabilities have evolved alongside the tech.
The first listening computers, 1950-80s
The power of automated speech recognition (ASR) means that its development has always been associated with big names.
Bell Laboratories led the way with AUDREY in 1952. The AUDREY system recognized spoken numbers with 97-99% accuracy – in carefully controlled conditions. However, according to James Flanagan, a scientist and former Bell Labs electrical engineer, AUDREY sat on "a six-foot-high relay rack, consumed substantial power, and exhibited the myriad maintenance problems associated with complex vacuum-tube circuitry." AUDREY was too expensive and inconvenient even for specialist use cases.
IBM followed in 1962 with the Shoebox, which recognized numbers and simple math terms. Meanwhile, Japanese labs were developing vowel and phoneme recognizers and the first speech segmenter. It's one thing for a computer to understand a small range of numbers (i.e., 0-9), but Kyoto University's breakthrough was to 'segment' a line of speech so the tech could go to work on a range of spoken sounds.
In the 1970s, The Department of Defense (DARPA) funded the Speech Understanding Research (SUR) program. The fruits of this research included the HARPY Speech Recognition System from Carnegie Mellon. HARPY recognized sentences from a vocabulary of 1,011 words, giving the system the power of the average three-year-old. Like a three-year-old, speech recognition was now charming and had potential – but you wouldn’t want it in the office.
Are you a pro? Subscribe to our newsletter
Sign up to the TechRadar Pro newsletter to get all the top news, opinion, features and guidance your business needs to succeed!
HARPY was among the first to make use of Hidden Markov Models (HMM). This probabilistic method drove the development of ASR in the 1980s. Indeed, in the 1980s, the first viable use cases for speech-to-text tools emerged with IBM's experimental transcription system, Tangora. Properly trained, Tangora could recognize and type 20,000 words in English. However, the system was still too unwieldy for commercial use.
Consumer-level ASR, 1990s to 2010s
“We thought it was wrong to ask a machine to emulate people,” recalls IBM’s speech recognition innovator Fred Jelinek. “After all, if a machine has to move, it does it with wheels—not by walking. Rather than exhaustively studying how people listen to and understand speech, we wanted to find the natural way for the machine to do it.”
Statistical analysis was now driving the evolution of ASR technology. In 1990, Dragon Dictate launched as the first commercial speech recognition software. It cost $9,000 — roughly $18,890 in 2021 accounting for inflation. Until the launch of Dragon Naturally Speaking in 1997, users still needed to pause between every word.
In 1992, AT&T introduced Bell Labs’ Voice Recognition Call Processing (VRCP) service. VRCP now handles around 1.2 billion voice transactions each year.
But most of the work on speech recognition in the 1990s took place under the hood. Personal computing and the ubiquitous network created new angles for innovation. Such was the opportunity spotted by Mike Cohen, who joined Google to launch the company's speech tech efforts in 2004. Google Voice Search (2007) delivered voice recognition tech to the masses. But it also recycled the speech data of millions of networked users as training material for machine learning. And it had Google's processing clout to drive the quality forwards.
Apple (Siri) and Microsoft (Cortana) followed just to stay in the game. In the early 2010s, the emergence of deep learning, Recurrent Neural Networks (RNNs), and Long short-term memory (LSTM), led to a hyperspace jump in the capabilities of ASR tech. This forward momentum was also largely driven by emergence and increased availability of low-cost computing and massive algorithmic advances.
The current state of ASR
Building on decades of evolution – and in response to rising user expectations – speech recognition technology has made further leaps over the past half-decade. Solutions to optimize varying audio fidelity and demanding hardware requirements are easing speech recognition into everyday use via voice search and the Internet of Things.
For example, smart speakers use hot-word detection to deliver an immediate result using embedded software. Meanwhile, the remainder of the sentence is sent to the cloud for processing. Google’s VoiceFilter-Lite optimizes an individual’s speech at the device end of the transaction. This enables consumers to ‘train’ their device with their voice. Training reduces the source-to-distortion ratio (SDR), enhancing the usability of voice-activated assistive apps.
Word error rate (WER - the percentage of incorrect words that appear during a speech-to-text process) is improving vastly. Academics suggest that by the end of the 2020s, 99% of transcription work will be automatic. Humans will step in only for quality control and corrections.
ASR use cases in the 2020s
ASR capability is improving in symbiosis with the developments of the networked age. Here's a look at three compelling use cases for automated speech recognition.
The podcasting industry will bust through the $1 billion barrier in 2021. Listenership is soaring and the words keep coming.
Podcast platforms are seeking out ASR providers with high accuracy and per-word timestamps to help make it easier for people to create podcasts and maximize the value of their content. Providers like Descript convert podcasts into text that can be quickly edited.
Plus, per-word timestamps save time, empowering the editor to mold the finished podcast like clay. These transcripts also make content more accessible to all audiences, as well as help creators improve their shows’ searchability and discoverability via SEO.
More and more meetings take place online these days. And even those that don’t are often recorded. Minute-taking is expensive and time-consuming. But meeting notes are an invaluable tool for attendees to get a recap or check a detail. Streaming ASR delivers speech-to-text in real-time. This means easy captioning or live transcription for meetings and seminars.
Processes such as legal depositions, hiring, and more are going virtual. ASR can help make this video content more accessible and engaging. But more importantly, end-to-end (E2E) machine learning (ML) models are further improving speaker diarization – the record of who is present and who said what.
In high-stakes situations, trust in the tools is essential. A reliable speech-to-text engine with an ultra-low WER removes the element of doubt and reduces the time required to produce end documents and make decisions.
On the record
Do you think Knight Industries ever appraised the transcript of KITT and Michael's conversations to improve efficiency? Maybe not. But, turbo-charged by the recent move to working from home, more and more of our discussions are taking place online or over the phone. Highly accurate real-time natural language processing (NLP) gives us power over our words. It adds value to every interaction.
The tools are no longer exclusive to big names like IBM and DARPA. They are available for consumers, businesses, and developers to use how their imagination decides – as speech recognition technology steadies to overtake the promises of science-fiction.
Interested in speech recognition? Check out our roundup of the best speech-to-text software
Graeme John Cole is a contributor for Rev, creator of the world's most accurate automatic speech recognition engine, Rev.ai.