Why are enterprises slow to adopt machine learning?
A lack of understanding about machine learning is holding enterprises back from adopting this emerging technology
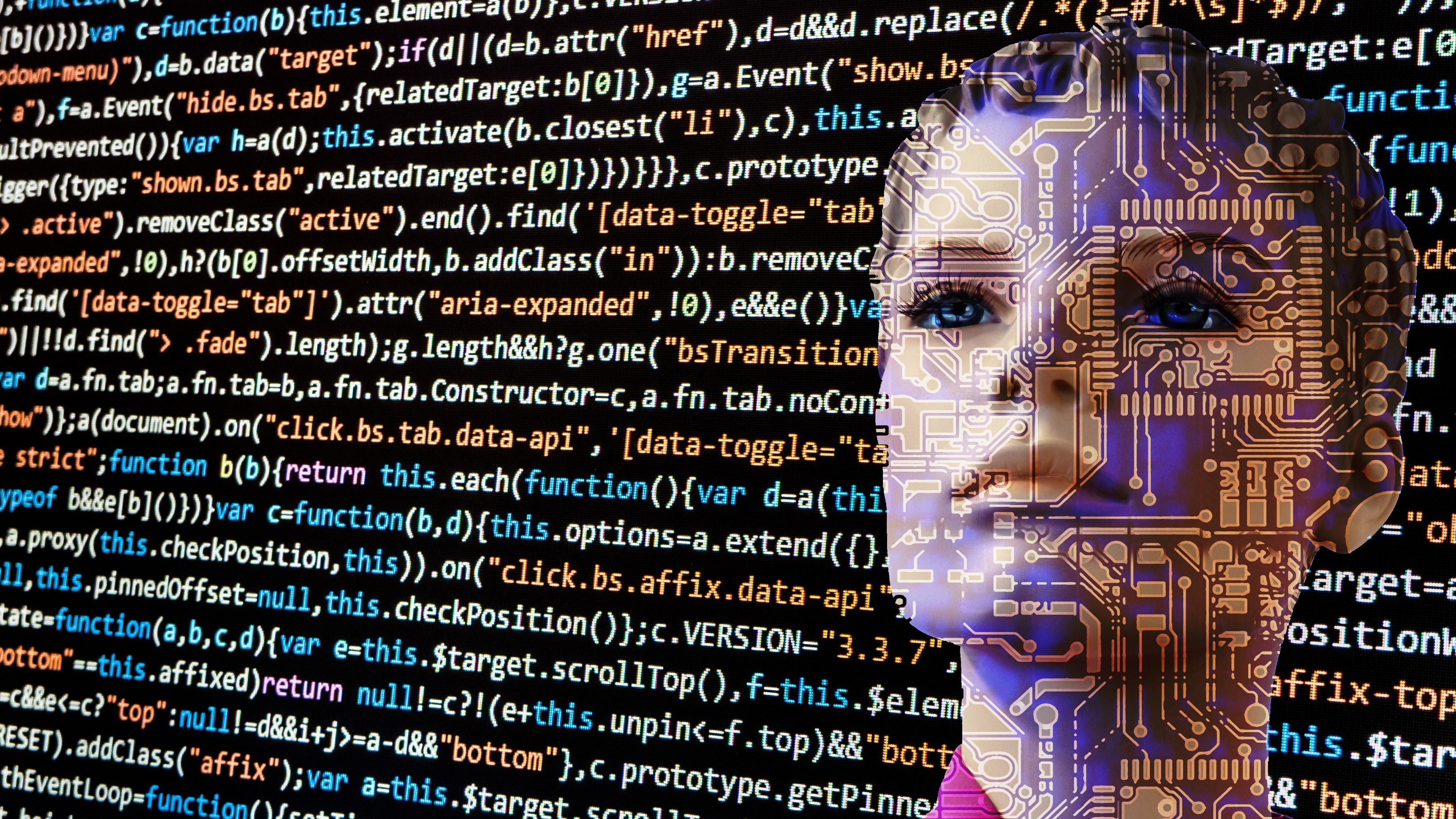
Machine learning has the potential to transform the way organisations interact with the world, to move faster and to provide better customer experience. But while machine learning’s long-term potential certainly looks bright, its adoption in the enterprise may advance more slowly than originally thought. So what’s the holdup? John Rakowski, Market Specialist for Application Performance Management and Analytics, at AppDynamics discusses the challenges for enterprises when adopting machine learning technology.
- Also check out the best AI platforms for business
What are the main challenges standing in the way of widespread adoption of machine learning in the enterprise?
Part of the challenge is a lack of understanding around what machine learning is. Machine learning is an application or subset of AI, which is generally thought of as higher-order decision-making intelligence. Machine learning is really about applying mathematics to different domains. It locates meaning within extremely large volumes of data by cancelling out the noise. It uses algorithms to parse the data and draw conclusions from it, such as what constitutes normal behaviour.
Do you think that the lack of understanding stems from uncertainty about what machine learning can do?
It’s important to understand that machine learning algorithms don’t enter chess tournaments. What they are really good at is adapting to changing systems without human intervention while continuing to differentiate between expected and anomalous behaviour. This makes machine learning useful in all kinds of applications - think everything from security to healthcare -as well as classification and recommendation engines, and voice and image identification systems.
Consumers interact daily with dozens of machine learning systems including Google Search, Google ads, Facebook ads, Siri and Alexa, as well as virtually any online product recommendation engine from Amazon to Netflix. The challenge for enterprises is understanding how machine learning can add value to their business.
So, how can machine learning be introduced to an enterprise?
Machine learning is usually introduced into an enterprise in one of two ways. The first is that one or two employees start applying machine learning to gain insight into data they already have access to. This requires a certain amount of expertise in data science and more importantly, domain knowledge. An understanding of the business value and the customer need for digital services (applications) that are utilised is fundamental— but these skills are often in short supply.
The second is by purchasing a solution, such as security software or application performance monitoring solution, that uses machine learning. This is by far the easiest way to begin to realise the benefits of machine learning.
For example at AppDynamics, we apply machine learning to understand what constitutes a ‘healthy’ application from a performance and user experience perspective. We utilise dynamic baselining to work out how each step in a user journey for an application should perform. For example, in an e-commerce application, this could include steps such as login, or search for product. These algorithms also take into account business variables such as important times of the year like Black Friday, and then alerts are generated when performance deviates. This saves organisations time in terms of manually working out acceptable performance thresholds and also ensures that our solution provides fast ROI in any complex, enterprise environment.
Sign up to the TechRadar Pro newsletter to get all the top news, opinion, features and guidance your business needs to succeed!
Is there a challenge with data preparations for enterprises introducing machine learning?
Machine learning can sound deceptively simple. It’s easy to assume that all you have to do is collect the data and run it through some algorithms. The reality is very different. Once you have collected the data, you then have to aggregate it. You need to determine if there are any problems with it. Your algorithm needs to be able to adapt to missing data, outlying data, garbage data, and data that’s out of sequence.
Is there a big issue for machine learning from the lack of public labelled datasets?
There is, yes, because for an algorithm to make sense of a collection of data points, it needs to understand what those points represent. In other words, it needs to be able to apply pre-established labels to the data.
The availability of publicly labelled datasets would make it much easier for companies to get started with machine learning. Unfortunately, these do not yet exist, and without them, most companies are looking at a ‘cold start’.
There’s a need for domain knowledge too, is this another challenge?
At its best, machine learning represents the perfect marriage between an algorithm and a problem. For example, at AppDynamics, we apply dynamic baselining algorithms to ensure that our customers get alerted early on emerging application performance problems. This means domain knowledge - knowing what is a problem - is a prerequisite for effective use of the technology. Unfortunately, in a number of enterprise IT use cases, knowledge is built up in siloes within organisations, resulting in disparate pockets of knowledge a lack of business context.
Are there cultural changes needed for an organisation to successfully adopt machine learning?
Companies need to accept that they need to move faster as a digital business, and machine learning and automation is a prerequisite for success. Data is at the heart of machine learning, and those companies that culturally react to the importance of real-time insight that can be trusted and acted upon quickly, are those that will succeed and thrive.
John Rakowski, Market Specialist for Application Performance Management and Analytics at AppDynamics
John Rakowski is Director of Product Marketing for Application Performance Management (APM) and analytics at AppDynamics. Prior to his current role, John was the lead analyst for APM and IT Operational Analytics (ITOA) at Forrester Research helping clients with their APM and analytics strategies.
He puts together and delivers high scoring keynotes, coached exec leaders on how to present to internal and external audiences.