Meet your new weather forecaster: the supercomputer
Supercomputers transforming our ability to predict weather
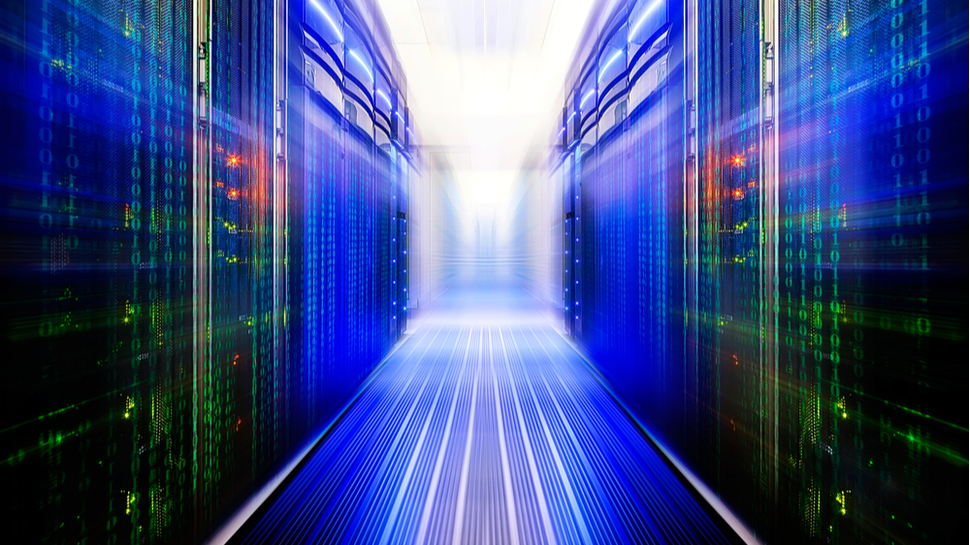
Predicting the weather has a long history. As far back as 650BCE the Babylonians were using cloud patterns and astrology to make forecasts. But it was only in the 1800s that the science of weather forecasting truly started.
Zaphiris Christidis is Segment Leader, Worldwide Weather at Lenovo.
It began with the sinking of the Royal Charter ship off the coast of Anglesey, an island in Wales, in October 1859. In response, an English Royal Navy officer Robert FitzRoy developed weather charts that he called “forecasts”. FitzRoy went on to set up 15 land stations, which used a telegraph to transmit daily weather reports and so the modern science of weather forecasting was born.
Fast forward 150 years and the science of weather forecasting has changed beyond recognition. Today, thanks to supercomputers, it’s possible to make much more accurate predictions about the weather.
Faster, more accurate results
So, how do these supercomputers make their predictions? First, it makes sense to explain what a supercomputer is. Put simply, it is a large array of smaller computers and processing equipment aggregated to make one large, smart and very powerful computer. Frequently found in the science, engineering, and business sectors, they can reduce the time taken to solve problems to days rather than months.
But for supercomputers to make weather predictions, they need to obtain data from somewhere. The data used in weather forecasting comes from a wide variety of sources – it’s supplied by satellites, weather stations, balloons, airplanes and even ships. Forecasters also have access to the Global Telecommunication System (GTS), which collects and disseminates data four times a day in six-hour intervals. The data supplied by these sources can be anything between 500 gigabytes and one terabyte. To put this into context, around 130,000 digital photos would require one terabyte of space – almost 400 photos every day for a year. But before the data can be used, it must be put through a process of quality control. Once that process has been completed, mathematical models are then used to make forecasts. Known since the 19th century, these are equations that describe the state, motion, and time evolution of various atmospheric parameters such as wind and temperature.
Massive compute power
Turning these equations into accurate forecasts requires an additional factor – compute power. To understand how this works in practice, it makes sense to use a simple illustration. If the United States were divided into a mesh of 10km blocks, then a certain level of compute power would be needed to provide localized forecasts inside each block. The difficulty arises, however, when the size of the blocks is reduced. Thunderstorms, tornadoes and smaller scale effects are very much linked to local weather, and with a large mesh it’s easy to miss them. It’s similar to being a fisherman – a much denser net is needed to catch small fish.
But going down to a smaller mesh requires an extraordinary amount of compute power. Taking the same example, 100 computational nodes might be needed to carry out a forecast on a grid of 10km blocks. Doing a forecast on a 5km mesh of the same area would involve increasing the compute power by a factor of 16 and using 1,600 nodes – servers that network together to form a cluster. And going down to an area of 2.5km would involve boosting the compute power by another factor of 16.
Enter AI
Because of the enormous amounts of compute power involved in making these calculations, scientists are now looking at how other technologies like artificial intelligence can improve forecasting. Instead of using brute-force computation to forecast weather based on present conditions, AI systems review data from the past and develop their own understanding of how weather conditions evolve. And they are already having a significant impact on forecasting. For example, the UK’s Meteorological Office recently carried out a pilot of AI technology to predict flash floods and storms. Using radar maps from 2016 to 2018, the system was able to accurately predict patterns of rainfall in 2019 for 89% of cases. Advancements in technology mean its four-day forecast is now as accurate as its one-day forecast was 30 years ago.
Bumping up against the Butterfly Effect
New technologies will undoubtedly usher in an era of more accurate forecasting, but they will never be able to make long-term predictions about the weather with 100% accuracy. That is because the equations that are used to make weather forecasts are non-linear – they have a degree of chaos embedded in them. As early as the 1960s, Edward Lorenz, an MIT meteorologist, was arguing that it was fundamentally impossible to predict the weather beyond ten days. Central to his argument – which later became known as chaos theory – was the claim that small differences in a dynamic system like the atmosphere could trigger completely unpredictable results. The most famous formulation of this theory was Lorenz’s 1972 academic paper ‘Predictability: Does the Flap of a Butterfly’s Wings in Brazil Set Off a Tornado in Texas?’
Setting aside chaos theory, there’s another reason why forecasting may take time to become more accurate – the science itself. Although compute power doubles every two years or so, weather science takes longer to catch up. Supercomputers were first used in the US in the 1960s and 70s, but it took between ten and 20 years for forecasts to become much more accurate.
Still, the compute power that is now available has massively improved forecasting. When weather predictions were first made in the 1950s, the results were highly inaccurate because of the limited computational power available. To give an example of how things have moved on, a weather model that would have taken 600 years to run on computer systems in the 1960s now takes just 15 minutes on a standard Lenovo ThinkSystem server.
There is every reason to believe that as compute power increases in the next few years alongside our scientific knowledge of weather patterns, it will be possible to make even more accurate predictions. And with the ability to predict extreme weather, supercomputers have the power to save lives and make a profound impact on the world.
Are you a pro? Subscribe to our newsletter
Sign up to the TechRadar Pro newsletter to get all the top news, opinion, features and guidance your business needs to succeed!
Zaphiris Christidis is Segment Leader, Worldwide Weather at Lenovo.