Dispelling three popular myths about AI
Are these misconceptions holding back your organization’s AI strategy?
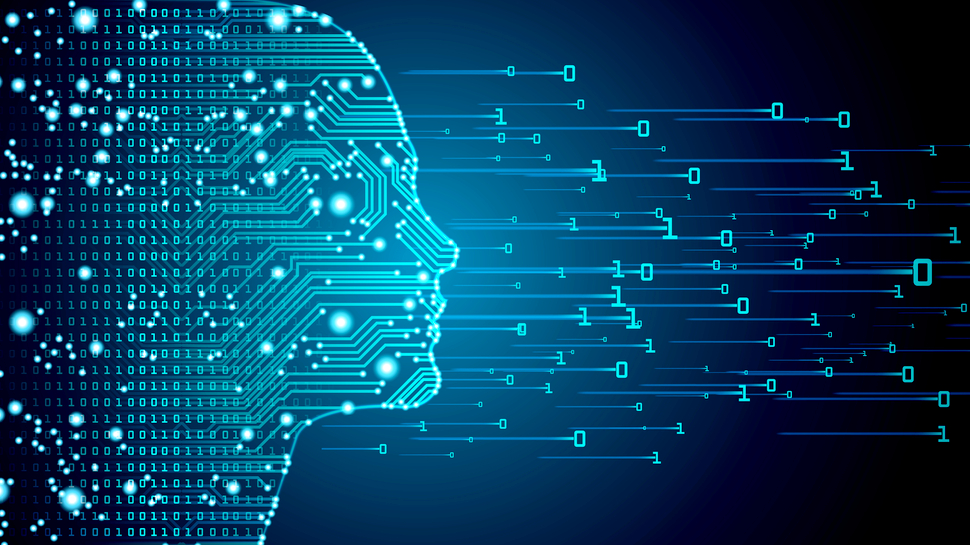
The many benefits of artificial intelligence (AI) can no longer be denied which is why more organizations are now turning to this emerging technology to remain nimble in the face of change. However, implementing an AI strategy without knowing what you want to achieve can not only be costly but ultimately sets a business up for failure.
To learn more about some common misconceptions that may be holding organizations back from yielding the full potential value of their investments in AI, TechRadar Pro spoke with global consulting data and analytics leader at EY, Beatriz Sanz Saiz.
- We've put together a list of the best cloud computing services
- These are the best AI platforms for business
- Also check out our roundup of the best workstations
Can you tell us about your background and when you first became interested in artificial intelligence?
I am a mathematician as a background, and I’ve been a partner at EY for the last 15 years. I’m currently leading data analytics and artificial intelligence (AI) for consulting globally. In my role, I lead more than 9,000 professionals driving the strategy to infuse analytics and AI into our work. Our clients come to us to help them solve their toughest challenges, to establish analytics and innovation, and help them navigate the hurdles, opportunities and ethics behind AI and other emerging technologies.
My interest in AI peaked when I began to realize there is a unique opportunity to challenge the status quo by harvesting analytics and AI to reinvent the traditional approach to problem solving. My ambition is to open the eyes of organizations by helping them understand that greater adoption in AI in turn leads to new mindsets and encourages a transformation culture that can truly benefit their core business.
What are the biggest hurdles holding organizations back from adopting AI today?
Looking at the findings of our recent EY Tech Horizon survey, businesses told us that the biggest barriers to embracing emerging technologies, such as AI, are lack of transformation culture (32%), legacy technology (32%), lack of collaboration across departments (30%) and lack of skills internally to compete in the digital economy (27%). Looking at the health sector in particular, lack of skills seemed to be an issue, with 78% of health companies agreeing that skills shortage is a major problem.
How can AI help businesses drive growth and when can they expect to see a return on their investment?
Businesses that want to transform to remain competitive need to embrace technologies such as AI, while leveraging three critical value drivers: placing humans at the center of their organization, leveraging technology at speed and enabling innovation at scale.
There are many long-term benefits to enabling and accelerating an AI strategy, such as radical customer centricity, agility, insights and prediction, efficiency, and growth. The best approach to get started consists of businesses asking themselves some key questions, such as “Will we still be relevant in two, five- or ten-years’ time?,” and “What kind of growth do we want to achieve?”. Then, by working through future-back scenarios and thinking strategically around how they incorporate AI, businesses can then work out what they need to modify to ensure they’re following a path to maintain relevance today, and 15 years from now.
Why do some companies still believe AI should be kept away from their core operations and data?
There is still a lack of understanding of the potential of AI, how it works and what it requires in terms of data infrastructure, trust and change management. If companies believe that AI comes with security risks, they may apply AI in a separate business unit, rather than implementing it in the heart of the operations. However, our survey found that 45% of organizations will invest in scaling AI in the next two years. Actually, this is the future. Using AI to run core processes to learn by the second, learn with every single transaction or customer interaction. This is the way to gain competitiveness and efficiency.
By building the AI strategy into their legacy applications and infrastructure, businesses can start to see the value gains far quicker. In order to gain better control over the risks implicated with AI, leaders should look to offer incentives to their IT staff to encourage them to future proof the workforce against potential security attacks and deploy robust training programs in AI competencies to help build skills for staff in-house.
How do AI and IoT overlap and what are the advantages of using these two emerging technologies together?
AI and IoT technologies are maturing exponentially and it is clear in our survey that the two technologies correlate. Over half (54%) of AI-focused companies also invested in IoT in the last two years, compared to 50% of overall corporates. Albeit a very slight uplift, this indicates that businesses willing to invest in AI are also aware of the benefits to implementing IoT, and these investments are effective, with 48% of AI-focused companies stating that IoT is having a very positive impact on their ability to innovate.
To get the most value out of these technologies, they should be used in tandem. IoT can help businesses collect data for every sensor enabling AI better measure processes, people’s behavior or an asset’s condition. Then, when this data is sent to the cloud, AI will enforce the correct algorithms and methods to process this information. For example, if you look at an autonomous vehicle, IoT collects the necessary data such as location, speed and proximity to objects, while AI will then translate this data into tangible actions, telling the car to slow down, turn a corner or stop.
Starbucks is also combining the technologies to great effect. The coffee giant has invested in connected espresso machines that can alert the company when they need tuning or maintaining, reducing downtime and improving the customer experience.
Companies embracing these crossovers are gaining expertise in the capabilities when AI and IoT are used simultaneously, allowing them to replicate techniques and gain value in other areas of their business. By examining which parts of the business are benefiting most and building proof-of-concepts, leaders can explore new solutions that deliver to their customers' needs.
What steps can organizations take to ensure that they’re using trusted data from a reliable source?
Data can be sourced from anywhere, such as business tools and applications, website analytics, industrial equipment, wearables, social media – its sources are limitless. However, to build a trusted AI system, data must be compliant, accurate, clean, and most importantly, relevant for the intended purpose. Data has to have meaning. It’s the most important thing to build trusted data and build AI at scale. Unreliable data can derive from poor data-collection practices such as customer relationship management (CRM) and enterprise resource planning (ERP) or from call centers, to name a few. Businesses that use practices such as these aren’t necessarily aiming for qualitative data.
To overcome this challenge, it's imperative that companies build a “business data fabric” and execute a strong data governance, as an integral part of the fabric, to help them monitor the data they use to train AI models. However, our research shows that only 8% of corporate companies have a governance function already established, so this is an area on which businesses need to be advised.
Consequently, AI itself can also be part of the solution to fix data quality, with algorithms being able to extract information from handwritten forms, amend data entry errors and match information across systems. AI can be used to self-discover data, enabling an automated matching between technical terms and business terms, so that data can be meaningful and trusted. Companies will still need human validation checks, but machine learning will “learn” from these human interventions with the system becoming smarter and smarter over time. In this way, businesses can ensure AI applications leverage only trusted data.
In fact, our Trusted AI Platform provides insights to organizations on the sources and drivers of risk and guides an AI design team in quantifying AI risks. By developing a quantitative score of an AI system’s residual risk, the EY Trusted AI Platform calculates the residual risk of an AI system. Based on the anticipated impact on users, stakeholder risk acts as a multiplier to technical risk, considering social and ethical implications. The evaluation of governance and control maturity acts as a mitigating factor to reduce residual risk of an AI system.
What advice would you give to a company trying to implement an AI strategy for the first time?
Firstly, businesses who are unskilled in this area should take their lead from organizations who said in our survey that they are implementing new incentives to encourage their workforce to upskill (58%) as well as enforcing mandatory training programs (57%). By taking this approach, they can begin by training the right employees before getting started.
Secondly, as previously mentioned, understanding the overall goal of what you want AI to achieve to take your business to the next level, then working out what that strategy looks like is the next obvious step. Once this has been initiated, companies must start walking the talk, identifying the right use cases and measures of success. Then they can progressively rethink their tech and data frameworks, data fabric and modernize their legacy technology infrastructure accordingly.
How do you think AI will evolve in the next five years and will the technology become as ubiquitous as cloud computing is today?
Just as the COVID-19 pandemic has accelerated the pace of change towards digital transformation, AI adoption will clearly speed up. AI will consolidate as the technology that will sit are the critical paths of any process of an Enterprise, whether is customer facing or back office operations.
AI will drive significant changes in the majority of industries’ value chains, and we are starting to see that now: look at health and biotech, retail, manufacturing and the public sector. These are just a few examples. It’s actually hard to think of an industry that will not be impacted. Even sports such as international automobile racing and other competitive sports are now driven by end-to-end data analysis.
- We've also highlighted the best business laptops
Are you a pro? Subscribe to our newsletter
Sign up to the TechRadar Pro newsletter to get all the top news, opinion, features and guidance your business needs to succeed!