Why deepfakes and AI trust issues impact businesses
What does it mean for enterprise AI?
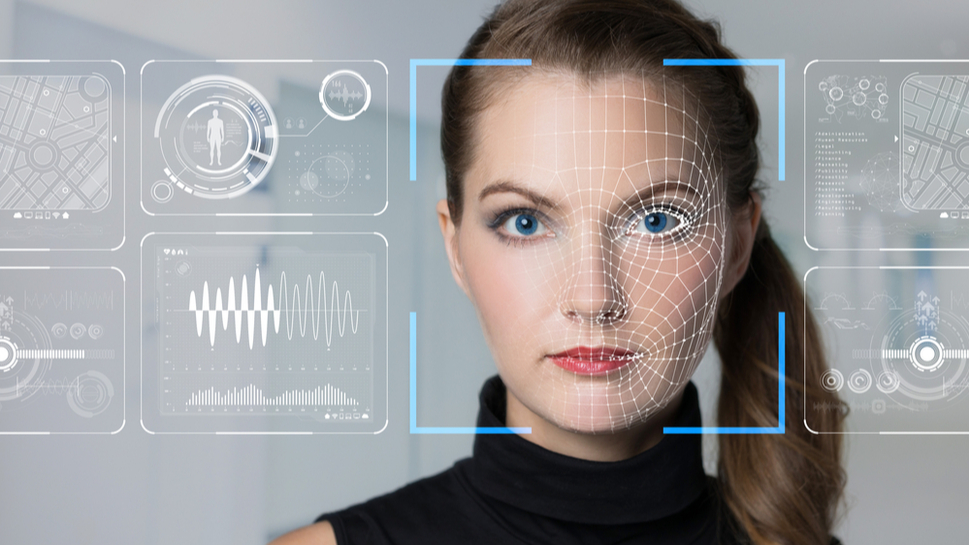
From the now-infamous Mother’s Day photo from Kensington Palace to faked audio of Tom Cruise disparaging the Olympic Committee, AI-generated content has made headlines recently for all the wrong reasons. These instances are sparking widespread controversy and paranoia, leaving people questioning the authenticity and origin of content they see online.
It’s impacting every corner of society, not only public figures and everyday internet users, but also the world's largest companies. Chase Bank, for instance, reported being fooled by a deepfake during an internal experiment. Meanwhile, a report revealed that in just one year, deepfake incidents skyrocketed in the fintech sector by 700%.
Today, there's a critical lack of transparency around AI, including whether an image, video, or voice was AI-generated or not. Efficient methods of auditing AI, which unlock a greater level of accountability and incentivizes companies to more aggressively root out misleading content, are still developing. These shortcomings combine to exacerbate the AI trust problem, and combating these challenges is contingent on bringing more clarity to AI models. It’s a primary hurdle for companies looking to tap into the massive value of AI tools but concerned the risk may outweigh the reward.
CEO and founder of Casper Labs.
Can business leaders trust AI?
Right now, all eyes are on AI. But while the technology has experienced historic levels of innovation and investment, trust in AI and many of the companies behind the technology has steadily decreased. Not only is it becoming harder to distinguish human- and AI-generated content online, but business leaders are also growing wary of investing in their own AI systems. There’s a common struggle to ensure the benefits outweigh the risk, all compounded by murkiness around how the technology actually works. It’s often unclear what kind of data is being used to train models, how the data impacts generated outputs, and what the technology is doing with a company's proprietary data.
This lack of visibility presents a slew of legal and security risks for business leaders. Despite the fact that AI budgets are set to increase up to five times this year, rising cybersecurity concerns have reportedly led to 18.5% of all AI or ML transactions in the enterprise being blocked. That’s a whopping 577% increase in just nine months, with the highest instance of this (37.16%) being in finance and insurance — industries that have especially strict security and legal requirements. Finance and insurance represent harbingers of what could come in other industries as questions around AI's security and legal risks grow and businesses have to consider the implications of using the technology.
Even while itching to tap into the $15.7 trillion in value AI could unlock by 2030, it’s clear that enterprises can’t fully trust AI right now, and this roadblock could only grow worse if issues aren’t addressed. There’s a pressing need to introduce greater transparency to AI to make it easier to determine when content is AI-generated or not, to see how AI systems are using data, and to better understand outputs. The big question lies in how this is accomplished. Transparency and waning AI trust are complex problems that don’t have a single smoking gun solution, and progress will require collaboration from sectors around the globe.
Tackling a complex technical challenge
Luckily, we’ve already seen signs that both government and technology leaders are focused on addressing the issue. The recent EU AI Act is an important first step in setting regulatory guidelines and requirements around responsible AI deployment, and in the U.S., states like California have taken steps to introduce their own legislation.
Sign up to the TechRadar Pro newsletter to get all the top news, opinion, features and guidance your business needs to succeed!
While these laws are valuable in that they outline specific risks for industry use cases, they only provide standards to be upheld, not solutions to implement. The lack of transparency in AI systems runs deep, down to the data used to train models and how that data informs outputs, and it poses a thorny technical problem.
Blockchain is one technology that’s emerging as a potential solution. While blockchain is widely associated with crypto, at its heart, the fundamental technology is built on a tamper-proof, highly serialized datastore. For AI, it can boost transparency and trust by providing an automated, certifiable audit trail of AI data — from data used to train AI models, to inputs and outputs during usage, and even the impact specific datasets have had on an AI’s output.
Retrieval augmented generation (RAG) has also quickly emerged and is being embraced by AI leaders to bring transparency to systems. RAG enables AI models to search external data sources like the internet or an enterprise’s internal documents in real-time to inform outputs, meaning models can ensure outputs are grounded in the most relevant, up-to-date information possible. RAG also introduces the ability for a model to cite its sources, letting users fact-check the information on their own rather than necessitating blind trust.
And when it comes to tackling deepfakes, OpenAI said in February that it would embed metadata into images generated in ChatGPT and its API so that social platforms and content distributors could more easily detect them. The same month, Meta announced a new approach to identifying and labeling AI-generated content on Facebook, Instagram, and Threads.
These emerging regulations, governance technologies, and standards are a great first step in fostering greater trust around AI and paving the way for responsible adoption. But there’s a lot more work to be done across the public and private sector, particularly in light of viral moments that have heightened public discomfort with AI, upcoming elections worldwide, and growing concerns over AI’s security in the enterprise.
We’re reaching a pivotal moment in the trajectory of AI adoption, with trust in the technology holding the power to tip the scales. Only with greater transparency and trust will businesses embrace AI and their customers reap its benefits in AI-powered products and experiences that spark delight, not discomfort.
We list the best AI website builders.
This article was produced as part of TechRadarPro's Expert Insights channel where we feature the best and brightest minds in the technology industry today. The views expressed here are those of the author and are not necessarily those of TechRadarPro or Future plc. If you are interested in contributing find out more here: https://www.techradar.com/news/submit-your-story-to-techradar-pro
Mrinal Manohar is CEO and founder of Casper Labs.