Transform your generative AI roadmap with custom LLMs
Could smaller models be the answer to business' gen AI woes?
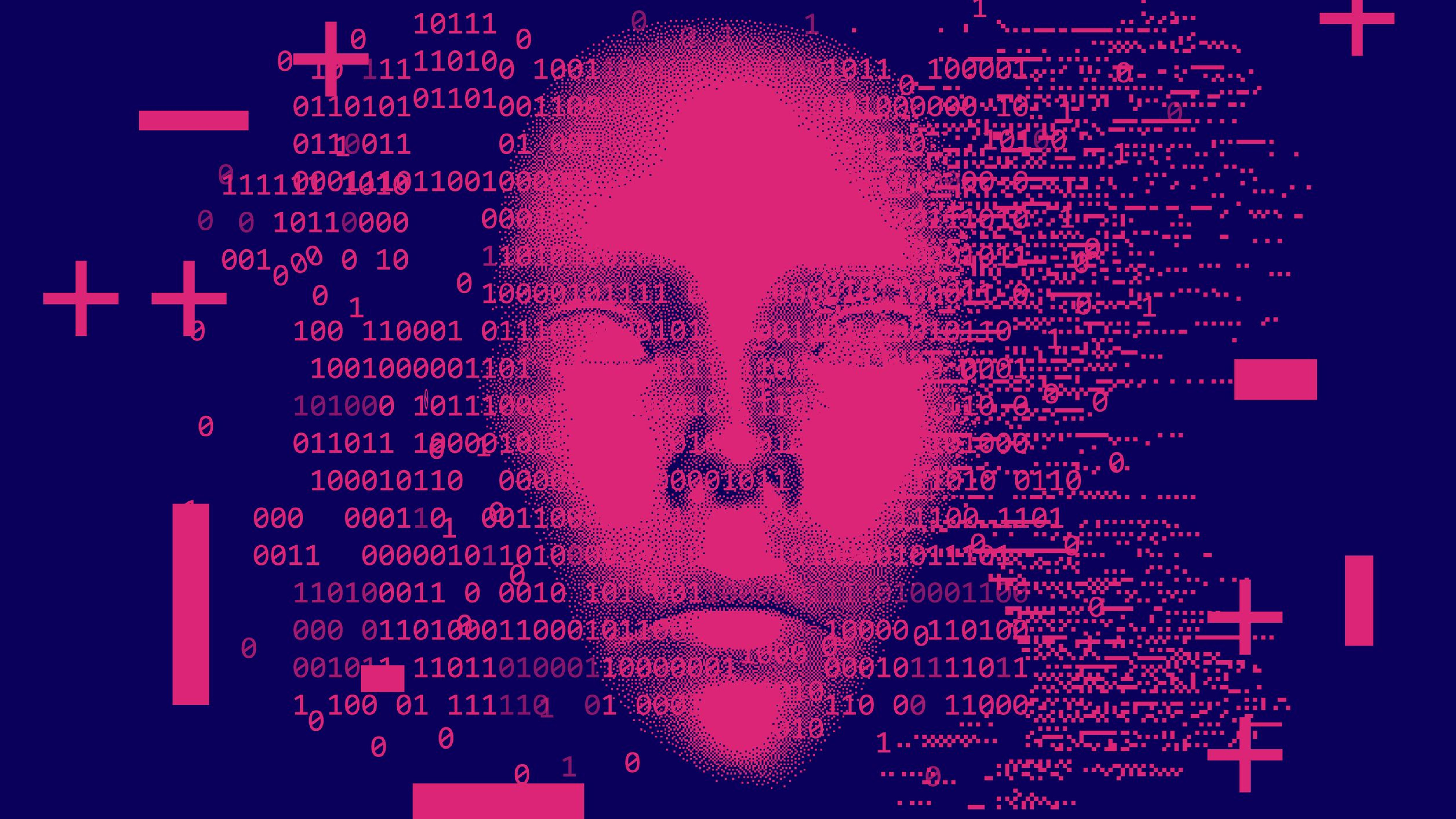
When it comes to generative AI models, bigger isn’t always better. What’s more important is using a tool that’s purpose-built for the task you’re trying to accomplish. A large language model (LLM) trained on a huge dataset may seem attractive for organizations looking to leverage the most amount of data possible, but it may not deliver the most accurate results. A smaller, customized model that is trained on a more targeted dataset, however, can enable businesses to control their data and ensure quality - something that is crucial when scaling generative AI use cases to gain the competitive edge.
VP Field Engineering for EMEA at Databricks.
Customization is key
The last year has thrown the spotlight on LLMs as the engines behind generative AI applications. Whilst these are appropriate for a consumer audience, there are many reasons that off-the-shelf, general purpose LLMs are not always ideal for an enterprise. For example, organizations can’t always inject their own data into these LLMs, so the model’s responses are not always relevant in an internal context. Additionally, many larger LLMs require that users grant access to data collection by the model’s creator, which can raise concerns about data privacy.
Smaller, customized language models can mitigate many of these concerns. Organizations can train smaller, customized models using their own data, meaning that responses account for internal context and are more relevant. An additional benefit of this approach is that organisations never have to share their data with a third party, keeping it secure, whilst also ensuring they can adhere to regulatory requirements. Smaller, customised models can also alleviate efficiency concerns, as training a generalised LLM can require significant time, money, and computing power. For instance, the larger the model, the higher the number of Graphics Processing Units (GPUs) are needed for its functioning. But GPUs have become scarce - and with demand outstripping supply, they’re expensive to obtain. Businesses, of course, want to keep costs low, so the fewer GPUs required to train and run inference on a model, the better.
Collaborate to encourage further innovation
As the majority of senior leaders look to scale generative AI projects efficiently, and consider the benefits of customized models, cross-organizational collaboration will also be essential. The generative AI boom is like any other industrial revolution; teams across the organization can benefit from working together to innovate and move forward. This approach also allows for gains in research and development.
Compared to general purpose LLMs, these custom-built models let developers create more highly specific systems with unique use-cases in mind. These allow organizations to focus on generative AI projects that are not only scalable, but have a higher-likelihood of producing a better return on investment because the model is built to address a specific problem or opportunity for the company.
The foundations matter
When building smaller, customized models, organizations should use an open, unified foundation for all its data and governance. One such way of doing this is through a data intelligence platform, which helps to ensure the quality, accuracy and accessibility of the data behind language models. Seeking ways to democratize this data will also be key, enabling employees across an enterprise to easily query corporate data with just their natural language. Through this, even non-technical staff can get the same insights as skilled data scientists and analysts, and quickly, thereby freeing up the time of in-house experts to focus on higher-level, innovative and even business-critical tasks. For enterprises who have previously relied upon third-parties to analyze their data, this approach frees up time and cuts down costs - putting innovation into the hands of their own employees. Providing access to data intelligence, and using smaller models, therefore encourages innovation and facilitates data-driven decision-making at scale.
Close collaboration across the industry is vital in facilitating the growth of generative AI globally. The more we collectively understand about the technology, the more we can use it productively. Equally, as senior leaders continue to focus on efficiency, they should consider the benefits of right-sized, customized open source models. These models are effective tools for business growth, allowing organizations to keep costs down, control their data and ensure quality as they continue to scale their generative AI use cases. Investing in an efficient generative AI strategy will future-proof organizations, helping streamline processes for years to come.
Are you a pro? Subscribe to our newsletter
Sign up to the TechRadar Pro newsletter to get all the top news, opinion, features and guidance your business needs to succeed!
We've featured the best AI website builder.
This article was produced as part of TechRadarPro's Expert Insights channel where we feature the best and brightest minds in the technology industry today. The views expressed here are those of the author and are not necessarily those of TechRadarPro or Future plc. If you are interested in contributing find out more here: https://www.techradar.com/news/submit-your-story-to-techradar-pro
Toby Balfre is VP Field Engineering for EMEA at Databricks.