CIOs must make the call on AI-based app development
Developing an effective strategy for investing in AI applications
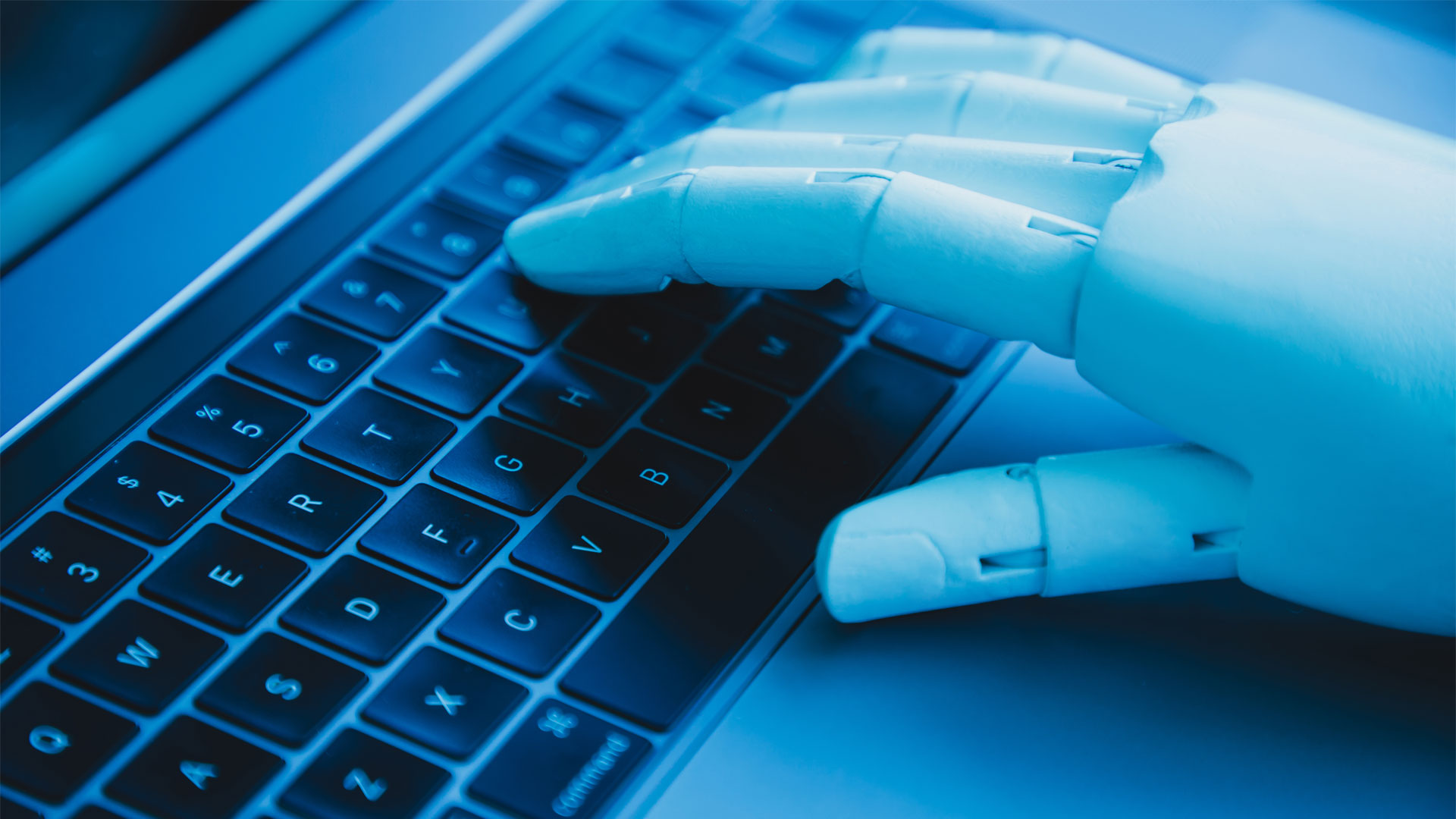
After bubbling under the surface for decades, AI has become a popular topic of public conversation. The possibilities of services such as ChatGPT and DALL·E have piqued everyone’s interest. But AI has much more to offer than writing student essays and generating uncanny artwork. It can have a significant impact on industry by empowering companies through applications such as computer vision, natural language processing, and product recommendation systems. CIOs must now decide which approach to take when developing in-house AI-based applications to harness it effectively.
The potential applications for AI are wide-ranging. In the automotive sector, computer vision can enable automatic safety systems that recognize pedestrians on the road. Natural language processing can facilitate in-vehicle voice commands. In manufacturing, computer vision can monitor quality and suggest proactive maintenance. In retail, checkout can be streamlined via automatic product and customer recognition. Financial services can detect transaction anomalies to prevent fraud. Medical companies can improve the speed and accuracy of diagnoses. Every type of company can enhance the richness and quality of their enterprise search, making valuable internal data easier to find.
Matt Foley is Director of EMEA Field Application Engineering at AMD.
Machine learning
There are two main phases to the machine learning lifecycle: training and inferencing. The training phase ingests huge amounts of data and implements AI to recognize patterns and build models. This involves high-performance computing data canter servers equipped with best-in-breed CPUs and data canter accelerators. The inference phase involves applying the model on real world data to produce an actionable output. This may employ similar hardware to the training phase, or it could operate via embedded devices.
To be effective, AI applications need comprehensive models derived from rich data. However, while pre-built tools have progressed significantly in terms of providing models that are trained on public, or commercially obtained, external data, the most valuable data for an organization lives inside its walls. This will necessitate some in-house development, where deploying the most cost-effective hardware and software ecosystem will be essential.
An AI-based application can provide more relevant insights if its models are customized with the organization's own data. This will allow it to deliver results that are more relevant to the organization's needs. However, as AI becomes pervasive, a blend of approaches that build on public Software as a Service (SaaS) solutions alongside in-house apps will likely deliver the most powerful results. The key here is to have consistent access across the stacks used for training and inferencing. These, in turn, can be integrated seamlessly with a CPU stack, a GPU stack, or an embedded stack.
Investing in AI apps
When CIOs are developing a strategy for investing in AI apps, they must ensure they are spending the company’s money effectively to achieve sufficient returns. Process efficiency improvements, productivity, and the resilience of the IT infrastructure are central to measuring the benefits. CIOs must choose the best architecture for their solution, which can be implemented quickly. This is why having a wide range of AI stacks to choose from is so important. CIOs will also need to conduct an impact assessment across the app lifecycle, to ensure it is securely managed for responsible innovation, and also complies with privacy governance regulations and frameworks such as NIST. This is because the AI models will most likely be derived from sensitive or proprietary data, or both, the confidentiality of which must be protected as valuable Intellectual Property. The cost implications of all these factors mean that CIOs must invest in those areas where the benefits are clear, long-lasting, and provide foundational improvements to productivity.
However, the algorithms underlying AI models are both time-consuming and costly to build. They depend on the availability of increasingly large datasets, and AI architects that can work on a diverse range of use cases. This is another reason why a consistent unified platform is essential. The parameters for models have increased in size from the thousands to the hundreds of billions in just a decade, which is a staggering exponential rise in quantity. Training a model with this many parameters requires a large enough amount of data and conscientious data governance. It’s also fundamental to guard against data bias, which could skew results. This has become a regular criticism of generic AI implementations trained on public datasets. Correcting this bias requires significant curation. All this means that model training takes time and expense.
Where AI is needed
The key decision-makers in determining an AI-based enterprise app investment strategy will be the executive team. They must consider where AI is needed, inventory the use cases, categorize the risk levels, and then assess how mature existing AI solutions are for their requirements. If these are well-established and feature-rich, there may not be a need to build bespoke in-house AI applications from scratch. Either way, enlisting a flexible existing platform and optimizer libraries ensures agility, so that the benefits of tried-and-tested code can be combined with business-specific customization on the right platform to match investment levels.
AI is still in that phase of early adoption and has already undergone multiple generations of ups and downs. But today’s AI promises to be the beginning of a cycle fed by extremely large models and large data sets. This “big data” phase for AI-based solutions, be they apps developed in-house, or application frameworks provided by the industry, is now in a clear growth stage. It’s a virtuous circle. As more business adoption of AI feeds into better apps, this in turn means better frameworks will be developed, increasing the efficiency of business processes. These improvements and benefits will further accelerate app development, increasing benefits, and reducing the cost of adoption. As soon as the benefits outweigh the costs for an organization, AI-powered apps should be on every CIO’s agenda.
Sign up to the TechRadar Pro newsletter to get all the top news, opinion, features and guidance your business needs to succeed!
Matt Foley is Director of EMEA Field Application Engineering at AMD.