McLaren harnesses AI to power real and virtual Formula 1 teams
AI can be the secret weapon to F1 success
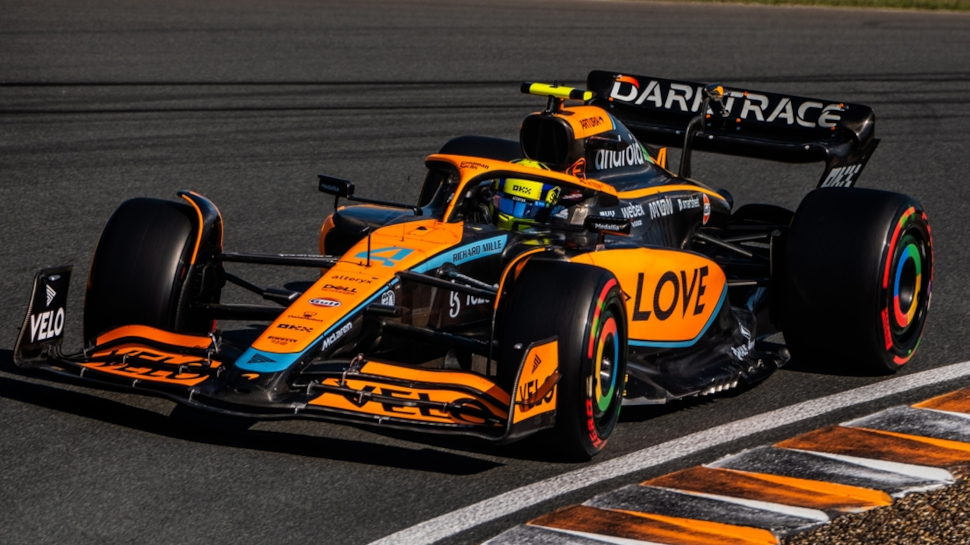
The digital systems used to inform and protect the McLaren F1 and esports teams are seeing a significant boost from using AI and machine learning to help get a leap on the competition.
From telemetry to cybersecurity, the amount of data harvested in Formula 1 is colossal, and understanding that often very complex data is crucial, especially in an environment where speed is of the utmost importance.
TechRadar Pro had the chance to speak to Ed Green, Head of Commercial Technology at McLaren, and James Hodge, GVP & Chief Strategy Advisor of the team's data platform provider, Splunk, about where AI fits into the equation, how it can help to protect the company's digital world and enhance it's decision making - as well as its limitations.
Security and decisions
As you might imagine, security is important for McLaren in all its operations. For its McLaren Shadow esports team, Green described a typical setup:
“If you’ve got eight gamers on stage, that means eight PCs behind them, and probably a further four directing and cutting the show, and so you end up with 24 PCs all involved."
To protect all these machines, Green explained that “we have standard endpoint protection we put across the estate. We use tools internally through various cybersecurity partners to monitor how our traffic moves, and we’ve got firewall providers to see exactly where the traffic is going”.
Although McLaren kept quiet about the exact software it uses, it is known that Darktrace is used in their security posture to certain degrees.
Are you a pro? Subscribe to our newsletter
Sign up to the TechRadar Pro newsletter to get all the top news, opinion, features and guidance your business needs to succeed!
Cybersecurity also has to be light to avoid sapping power from simulation rigs. “Lots of things are normally quite lightweight, so people don't want lots of agents on their machines doing bits and pieces”, says Green.
“We have natural endpoint clients we use across McLaren, they report up into a serious of dashboards which can be useful - I can get a view of that to monitor during the race”.
Green also explained that AI and machine learning is used for for the team's cybersecurity, not just for race data:
“We’ve used a lot of machine learning and AI across [the cybersecurity] space, and in years gone that would mean our cybersecurity team would be full of lots and lots of graduates; its a really tedious and boring job to sit there looking through lines and lines of cybersecurity information."
"Now, through the use of a lot of machine learning and AI, we don’t have as big a cybersecurity team, but they've got more relevant context, so they can see where the information is going, so embracing machine learning and AI is really important for us.”
He added that “when you look at AI in cybersecurity, or in general, it’s either there to help you be more efficient, to help you merge and solve really big complex challenges, or its there to provide you with additional assistance."
"In cybersecurity, in the race team, in strategy in particular, AI is there as an aid to decision making; it's not executing for you. So it might be that you’re under really sensitive time pressures - you can have three seconds to make a decision for a pit stop - so by giving those people the next best decision or helping them simulate what might happen, that means when the time pressure is on, we can make the right decision."
Even though AI in this context is used predominantly for the real-life Formula 1 team, Green did suggest that it may come into play for the esports F1 team as well in the future.
The importance of data
Data provider Splunk began its relationship with the McLaren Formula 1 team in 2020 as a platform for supplying the all important telemetric data of the cars, before later being signed up to help support the Shadow McLaren esports team.
Hodge explained how more advanced and predictive computations can be made using its AI tools. He mentioned the example of predicting tire degradation, which can be affected in the game by numerous factors such as the virtual track temperature and the level of driving aggression:
“We can start to do predictive analytics to say ‘where do we think we're going to get to a certain point at which the tires are no longer performant against coming in to the pit stop’, and so that’s where we started to look at the telemetry in the game to help with race decisions.”
Hodge echoed how AI can be an aid to decision making rather then being the decision maker. When it comes to AI's involvement in pit stop strategy, for instance, Hodge said:
“You might not want AI to flash up to say 'pit now'. You’ll probably want a human in the loop to say, ‘actually, we couldn’t add this data feed to that model, so it’s not quite right.’”
In explaining why automating decision making is so difficult, Hodge gave the hypothetical example of using AI to control your lights at home:
"It starts off simple: when I walk in the room I want them on. OK, how long should they stay on for? Until you see no motion, or they should stay on till midnight because I always go to bed at 11.30pm. Well, you’ve stayed up late to watch a film, so its twelve o’clock and they’ve gone off; I’m watching a film so I wouldn’t have moved, so the lights have gone off. So actually, what’s seemingly a simple problem becomes very complicated. Now, when you think about that in enterprise technology, it gets even harder."
He stressed the importance of having adequate data built up before relying on AI tools. And even besides AI, traditional statistical methods of prediction still have their place:
“I think its about layer upon layers upon layers [of data]. So when we look at, say, cybersecurity, can we first observe everything in the whole world? - this is where we are starting to see different security teams and IT monitoring teams coming together a lot more, because they all want to observe everything digital that’s happening and put context on top of it."
"Now lets look at statistical outliers. That’s normally a great place to start. Then can we add a bit of more basic ML-bound predictive modelling, to then, in a cybersecurity context, look at taking lots of different indicators together, and saying, 'do these potential statistical compromises now mean there is a higher likelihood of James being a bad actor?' That’s when you get more into the AI space.”
He also cautioned to keep in mind practical concerns when developing AI:
“You’ve also got to look at how far you want to push it and where is the best amount of effort for investment. Because quite often the statistical side gets you close enough to where you need to be. You can spend too long getting the perfect AI model, and almost wasting effort and money doing that."
"I am a big believer in getting the basics right, because no company in the world gets the basics perfect. The more you can do that, the more you can push decision making to the frontline staff to do what they’re employed to do.”
- Is data just as important to your business as well? Then you'll need the best data loss prevention services
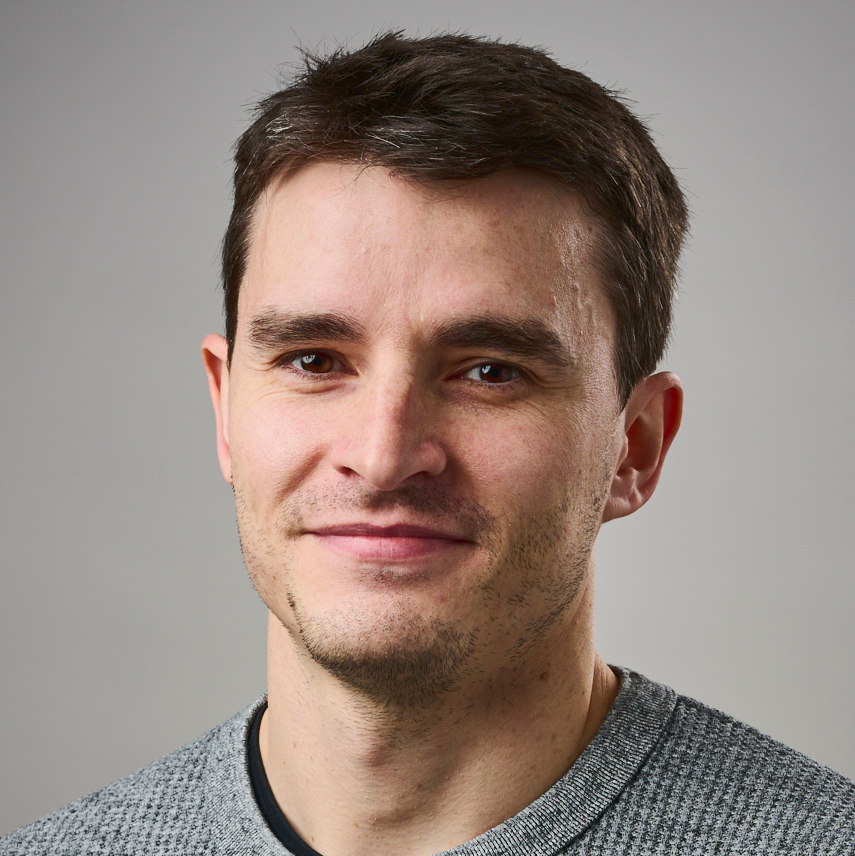
Lewis Maddison is a Reviews Writer for TechRadar. He previously worked as a Staff Writer for our business section, TechRadar Pro, where he gained experience with productivity-enhancing hardware, ranging from keyboards to standing desks. His area of expertise lies in computer peripherals and audio hardware, having spent over a decade exploring the murky depths of both PC building and music production. He also revels in picking up on the finest details and niggles that ultimately make a big difference to the user experience.