Improving business decisions with data and analytics
Leaders must learn to deploy data and analytics better
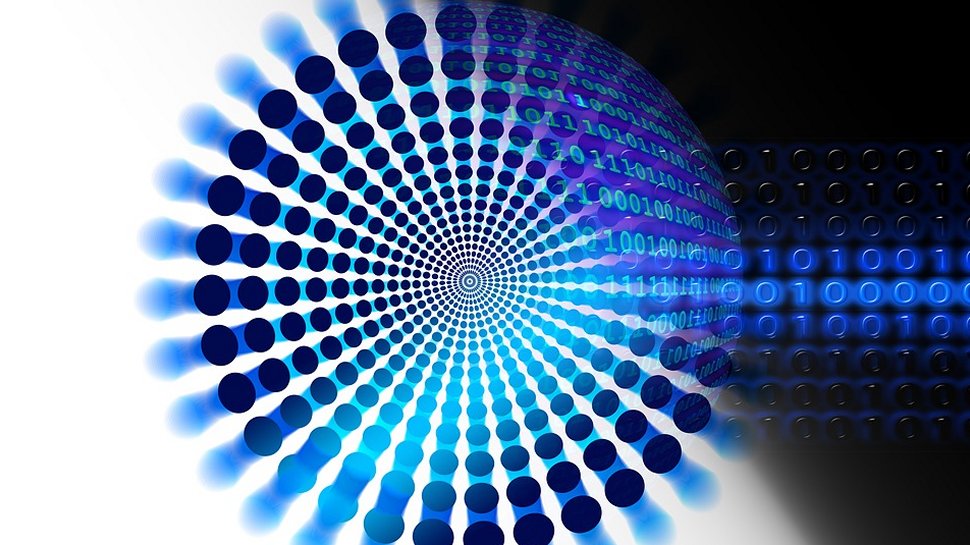
Organizations today make a variety of difficult decisions. Be it strategic and tactical, or operational and production-based – each require different approaches and considerations.
The increasing pace of business has compressed the timelines for making these decisions, with world and market events putting greater pressure on executives and managers to decide faster. In fact, organizations can often save money or gain competitive advantage by responding to or making decisions on operational opportunities and threats earlier or anticipating them before they happen.
Roy Schulte is vice president analyst at Gartner.
Data and analytics (D&A) play an essential role in improving the accuracy and effectiveness of business decisions by expanding the information that is available to decision-makers, whether those decision-makers be executives, line personnel or machines.
Application leaders should take several key actions to better use D&A to reinforce organizational decisions at speed. These include aligning the decision approach to the decision type, using the decision structure to better determine the right analytic technique, automating decisions where possible and employing external expertise where necessary.
Align the decision approach to the decision type
Organizational decisions vary widely - a strategic decision for example might be made through an ad hoc decision process spanning months. By contrast, an operational production decision may be executed entirely by a computer in a few milliseconds. Between these extremes, lie tactical and operational management control decisions, which call for a mix of decision processes and analytics.
An effective approach to decision engineering starts with an analysis of the business requirements, developed in conjunction with business stakeholders. Analysts contemplate the purpose of the decision and its role in your company’s operating model. This means understanding who will make the decision and what the time constraints are which surround it.
More specifically, it is important to use D&A to supply your executives and senior business managers with the right information and resources – data, analytics tools, data literacy training and specific analytics solutions – so that they can approach decisions confidently.
Are you a pro? Subscribe to our newsletter
Sign up to the TechRadar Pro newsletter to get all the top news, opinion, features and guidance your business needs to succeed!
Utilize the decision structure to determine the right analytical technique
A decision is structured if an analyst can develop an explicit decision model that documents the decision process and logic. Unstructured decisions are those where the decision process and logic are unclear, for example the criteria are subjective, or the algorithm is too complex to model. Tactical decisions for example are more structured than strategic decisions and typically make substantial use of data warehouses, reports, machine learning (ML) and “what-if” analysis.
For decision analytics to be most effective, every system that provides decision intelligence should be supported by a process of continuous decision improvement. The input and output of each decision analytic should be recorded, along with the business outcome of that each decision to monitor the effectiveness of the system. This should be done in collaboration with business managers, operations staff, and application developers. Decision improvement may involve innovating with new kinds of input data, new decision models, redesigned or retrained analytical models, or revised rules.
Automate decisions where possible
Organizations are finding ways to automate more and more decisions, primarily those in production but also some management control decisions. This is achieved by re-engineering unstructured or partly structured decisions into fully structured decisions as part of digital transformation and hyper automation initiatives.
Decision automation can reduce staffing costs, make decisions more consistent and explainable, and improve the speed of decision-making. Assuming the developer has implemented the analytics and rules well, an automated system will make fully structured decisions as well as, or better than, a person can. They are also less likely to be biased than human decisions because their rules and analytical models are generally visible, even before the systems are deployed.
When a decision cannot be fully automated, D&A teams can play a crucial role by helping automate some sub decisions and provide information on the final decisions that will be made by people.
Employ outside expertise to close skills gap
Some aspects of decision engineering are relatively new, and many organizations lack the skills that are essential to the field. D&A teams may have limited or no experience with decision modelling, business rule processing or optimization. Building virtual or real decision intelligence teams by acquiring skills in these disciplines is key to building great decision intelligence systems.
Your organization may need to hire business process modelers or management science experts or contract with outside firms who have expertise in these subjects. This allows the creation of a virtual or real decision intelligence team. These decision intelligence teams work with business managers, data engineers, ML engineers and application developers to model decisions and implement and deploy runtime software decision services.
At TechRadar Pro, we've featured the best business intelligence platform.
Roy Schulte is a distinguished vice president analyst at Gartner. His research focuses on best practices, design patterns and technology selection for real-time analytics and decision intelligence.