How businesses can overcome the AI implementation gap
The AI implementation gap
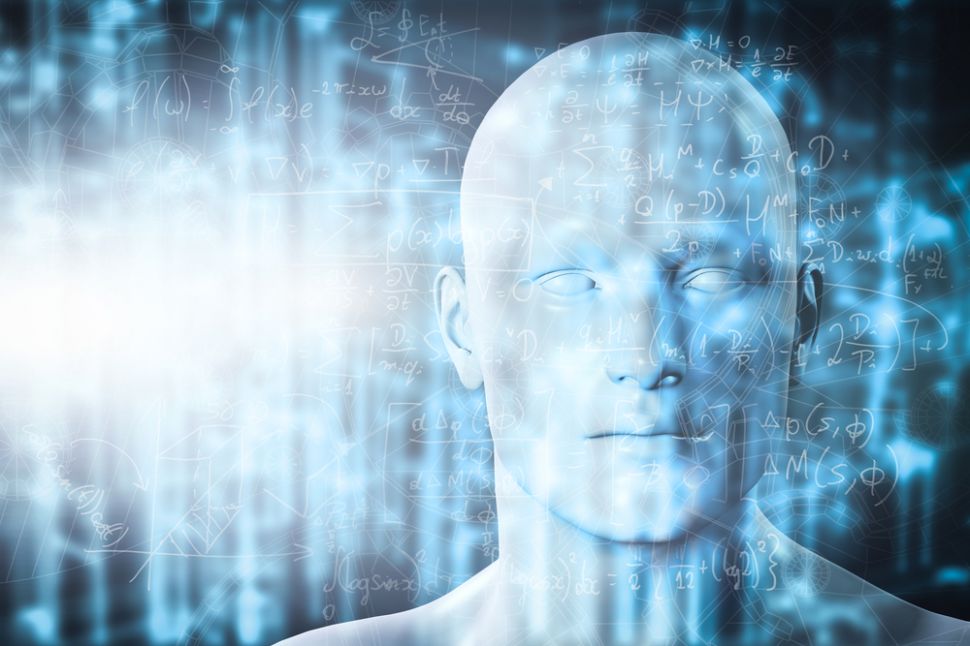
Artificial Intelligence (AI) is on the cusp of becoming mainstream. But research suggests that barriers such as a lack of data quality and technical expertise in the deployment of live applications are creating an implementation gap that will leave some organizations lagging behind in the AI race.
Paul Clough is Head of Data Science and AI at Peak Indicators.
In the 2020s AI looks more and more like the internet did in the 1990s. Every day it’s becoming less of a fringe topic discussed and understood only by the tech-savvy and more like one that businesses across all sectors and of all sizes can’t afford to ignore. The next five years will see more and more businesses use AI to deliver insights and automation more widely across their operations.
However, shifting from AI talk to trials and then onto effective implementation is no easy task. Even for IT literate, technology-focused businesses, successfully implementing AI so that it makes a significant impact on the organization can be an uphill struggle. For other businesses it can feel like a mountain to climb.
Moving from AI talk to trials
Recent research conducted by Opinium for an AI in Business report captures this difficulty and dichotomy – talking the talk is one thing, but effectively using AI is quite another. So, while four out of ten firms are investigating the benefits of AI, only one in 30 is actually actively using AI in at least part of their business. True, this figure is driven by low rates of adoption by micro businesses, but even amongst the largest businesses only ten percent are currently using AI widely, while 35 percent are using AI in a limited way.
The distinction is even more marked when looking at SMEs. Only one percent of small businesses and two percent of medium-sized businesses are widely using AI within their organizations, while 18 percent of small businesses and 32 percent of medium-sized ones are testing it in a limited way. And around a quarter of SMEs and a little under a fifth of large businesses are still just at the stage of talking about AI.
Looking at this data, it’s clear that there’s an implementation gap - and not just across smaller businesses. The willingness is there, but many organizations are struggling to move beyond investigating AI technology.
Only 16% of large businesses have strong data foundations
Overcoming this AI implementation gap means tackling a number of issues within an organization, including technical and cultural. But the most important step is arguably getting the data foundations for AI within a business right including data quality and governance. Research shows that even among large companies only 16 per cent have the right data foundations in place for AI.
Sign up to the TechRadar Pro newsletter to get all the top news, opinion, features and guidance your business needs to succeed!
Strong data foundations give a business a single reliable version of the truth on which decisions can be made, and around which AI-powered solutions can be built. A data-driven business has three key characteristics:
- Data insight is easily available at all levels within an organization and is used to back up every decision
- Data is combined in one single architecture to enable deeper analysis
- Advanced analytics and AI guide actions across multiple business processes
In contrast, organizations with poor data foundations will make decisions using intuition and gut feel. They do not systemically collate data and tend to have data that is unreliable and time-consuming to collate.
A first step to move towards being a data-driven business with strong foundations for AI is to understand what data an organization holds, and what it’s being used for. This can often be challenging, but auditing and cataloguing datasets can help.
Next, identifying attributes of data quality through profiling and mapping out data journeys for specific uses will help in the drawing up of ideas around what good data quality might mean, both generally and for specific uses, where the issues might be, and what steps will need to be taken.
The next stage in the road to achieving a high level of data quality is looking at how it is gathered. Unsurprisingly, the biggest cause of poor quality data during collection are errors in manual data entry. This could be by customers or employees. One way to minimize these is through validation mechanisms, such as not letting users proceed until all required fields have been filled in a proper format.
This brings up a key problem with addressing data quality - it's a people problem not just a technological one. We can use tools to fix technical problems, but we also need to adapt and change culture and business processes.
By establishing a strong culture of data governance (and by extension data quality), people will spot data issues more easily, understand the impact of problems on the business fully, and work to address them.
As well as culture, any organization must establish rules (based on the attributes they want their data to have) to ensure it keeps its quality through the data lifecycle. There are lots of open-source tools and data analytics companies that can help with this.
The final stage is monitoring and reporting. Ensuring data quality is an ongoing process. Not only are you always collecting new data, but the quality of that you do have could change as you use it for different purposes. Data-quality dashboards for specific jobs, functions and departments, could be produced to measure quality and ensure its fitness for purpose.
Data is key to unlocking AI potential
There are of course other technical barriers to AI adoption within a business. Many businesses lack expertise in AI deployment. Again, this is also a people problem - or more specifically a recruitment problem - as well as a technical issue.
Another issue is failure to bake-in user experience at the start of any AI initiative. If end users choose not to use a solution for whatever reason, adoption rates will stay low, the return on investment will be zero and the business will see no benefit. So it is critical from day one to understand how users want to use the data and their preference for interacting with the data or dashboards.
Added to this are cultural and business barriers that inhibit progress from talk to trials: a lack of real appetite for and support for AI initiatives at board level; failure to create a compelling business case for AI by those lower down the organization; competing investment initiatives and so on.
All of these issues need to be addressed and worked through. But without strong data foundations at its heart any AI project will struggle to move from talk to trails and beyond to successful implementation.
At Techradar we've feature the best small business software.
Paul Clough is Head of Data Science and AI at Peak Indicators and Professor of Search and Analytics within the Information School at the University of Sheffield.