Generative AI race sparks urgent demand for data management solutions
Evaluating current AI readiness
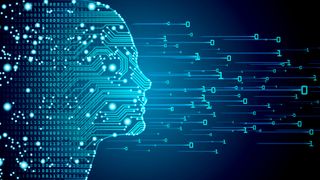
At the core of this strategy lies the imperative task of establishing the right data foundations for AI and machine learning (ML) endeavors. Business leaders must navigate these hurdles as they embark on the journey of training industry specific specialized LLMs used for generative AI. The key to training high-quality models lies in ensuring that your data house is in order. Below are four actionable tips to strategize for the future.
Implement a data strategy
At the core, the foundation of generative AI lies in the formulation of a comprehensive data strategy. For organizations that are accustomed to managing their data in silos, where different units, departments or applications exist in relatively isolated analytical environments, this can be a huge challenge. It can also be a hurdle for organizations that have grown through acquisition, where data assets reside in multiple systems and locations.
Establishing a cohesive data strategy that encompasses data ingestion, processing, and the creation of experimentation sandboxes across the enterprise is a critical first step to being able to use generative AI most effectively. Additionally, consideration should be given to the optimal placement of data scientists within this framework.
It may be tempting to bypass this step and run headlong into AI projects without taking into account the broader data strategy, but organizations who do so are more likely to find their initiatives falling short of the goal.
Hemanta Banerjee is Vice President of Public Cloud Data Services at Rackspace Technology.
Establish an AI data architecture
When a suitable data architecture is lacking, it can result in the emergence of technical debt and its associated consequences of failure to improve inefficient, outdated processes. While a suboptimal architecture might suffice for small data volumes or limited use cases, scaling up can cause increased costs. In the best-case scenario, costs escalate linearly, while in the worst-case scenario, costs surge exponentially in tandem with the data volume.
Furthermore, as the number of data pipelines increases, the complexity of monitoring them intensifies. If pipelines are scattered and lack consistency, the task of monitoring them becomes far more demanding with complexity and associated costs growing exponentially as the number of data assets, and hence the number pipelines, grows. This highlights the significance of designing a robust architecture and leveraging frameworks that fosters systematic data management and streamline Data Ops.
Develop an operating model
An often-overlooked aspect of effective generative AI implementation is the need for a well-defined operating model for the data platform. This model should serve as a set of guidelines that helps organizations to classify and categorize their data sets, reports, and resources and encompass various facets, such as data layering, service utilization, security protocols, governance frameworks, cataloging practices, and the differentiation between enterprise data and experimental datasets.
By documenting the operating model, organizations can establish a blueprint for effective data management, ensuring that data assets are treated with the same level of consideration as any other critical infrastructure component. Most importantly it provides a framework that allows teams to independently experiment with AI without creating a sprawl and increasing costs in the process.
A robust data operating model facilitates efficient data governance, enables consistent decision-making, and promotes a coherent understanding of the organization's data landscape. It becomes a foundational element in driving adoption of AI in the organization and maximizes the data value derived from data assets.
Measure data adoption across the organization
Data adoption is the transformative process through which businesses seek innovative approaches to enhance productivity and proactively address customer needs. Amidst the buzz surrounding generative AI, organizations shouldn't lose sight of the need to drive informed decision-making in a deliberate and systematic manner. For example, we can examine a supply chain scenario where category managers depend on a robust data platform and analytics to identify leading suppliers based on punctual deliveries or monitor suppliers exhibiting consistent delays and error rates. By driving data literacy and data driven decision making the organization creates a culture where AI enabled digital transformation has a significant chance of adoption and hence success.
Herein lies the importance of measuring organizational adoption in a structured manner. After setting a data strategy, implementing the right architecture, and defining the target operating model, only by establishing a mechanism to measure the adoption of data across the organization can companies truly assess the progress they are making and understand where and how they can most effectively leverage AI.
Depending on the foundational maturity of the organization, reaping the full benefits of generative AI may take several months, or even years. It hinges upon organizations ensuring the adoption of best data practices and a continuous evaluation of how those capabilities are being harnessed to drive innovation.
Conclusion
By establishing the necessary frameworks, investing in data management, and fostering a culture of data-driven decision-making, organizations can position themselves to take advantage of the generative AI innovation wave, and make real breakthroughs across the business. By contrast, those companies that rush into generative AI projects without a data first approach are likely to face significant obstacles along their journey and find themselves struggling to make progress.
Are you a pro? Subscribe to our newsletter
Sign up to the TechRadar Pro newsletter to get all the top news, opinion, features and guidance your business needs to succeed!
Hemanta Banerjee is Vice President of Public Cloud Data Services at Rackspace Technology.