The future of networking is happening at the edge
A world moving to the edge
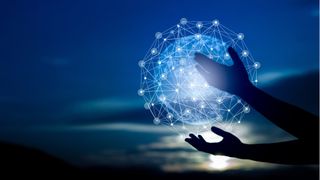
Networks are changing. Its future and much of the innovation and profitability are happening at the edge. There’s a shift starting to take place and it’s one that you can’t ignore if you want to take advantage of the possibilities the network edge provides. Applications are expected to move from data centers to the edge and in doing so, will open up a huge new market opportunity.
Julius Francis, Director of Product Management & Marketing, Juniper Networks.
The numbers tell a compelling story. The global edge computing market is expected to grow at a compound annual growth rate of 34 percent between 2018 and 2024, hitting a value of nearly US$7 billion. Europe is one of the major players in the space. But at the heart of this growth forecast is the importance of intelligence in making edge networking work to its full potential.
Fueling the boom in edge computing is the rapid adoption of the internet of things (IoT), autonomous vehicles, high-speed trading, content streaming and multiplayer games. These applications share one specific need – near zero-latency data transfer, usually defined as less than five milliseconds.
For many emerging technologies, even five milliseconds is too high, which is why it makes sense to move business applications and data as close as possible to the data ingestion point. This reduces the overall round-trip time and as such, applications such as autonomous vehicles can access information in real-time to navigate effectively and avoid collisions.
Overcoming obstacles
Edge computing isn’t without its challenges, however – for telecommunications service providers in particular. One complicating factor that we’re seeing increasingly is network functions moving toward cloud-computing applications deployed on virtualized, shared and elastic IT infrastructure.
Look at most virtualized environments and you’ll see each physical server hosts dozens of virtual machines and/or containers. And these machines are constantly being created and destroyed faster than humans can manage them. Orchestration tools can be useful here because they automatically manage the dynamic virtual environment in normal operation. However, when it comes to troubleshooting, humans are still the ones responsible for manually carrying it out.
And that’s when things can become tough. Service disruptions have a negative effect on service providers so they, in turn, put pressure on the IT management to address any issues as quickly as possible. The information they need to identify the source of the problem and find a solution is already there and the biggest challenge is in fact trawling through reams of telemetry data from the hardware and software components. What they need is a helping hand to work through the data quickly and gain the right insights based on trends they’re seeing.
Are you a pro? Subscribe to our newsletter
Sign up to the TechRadar Pro newsletter to get all the top news, opinion, features and guidance your business needs to succeed!
A data-led response
A data-rich, highly dynamic, dispersed infrastructure is the perfect environment for artificial intelligence, specifically machine learning. Machine learning is effective in wading through pools of data to spot patterns in a way that exceeds the capabilities of network operators.
Tools based on machine learning constantly get better and adapt by self-learning from their experiences and performing speedy human-like analyses as a result. And with automation added to the mix, the insights can be turned into actions. This helps overcome the challenge of arriving at concrete outputs in the dynamic, disaggregated world of edge computing.
Deploy machine learning and real-time network monitoring and the information you get at the other end will power automated tools that can provision, instantiate and configure physical and virtual network functions. What’s more, this process will be done much faster and more accurately than if a human were to manually carry out the task. By using the smarts and efficiency of both machine learning and automation, you’ll save considerable staff time, which can be used for leading strategic initiatives that contribute more directly to building the bottom line.
Building and scaling in the cloud
Machine learning can play a key role in app development in an edge computing context. Telcos have largely moved away from waterfall software development where lengthy sign-off stages between each department meant that apps could take years to complete. Cloud-native development relies more heavily on agile development and DevOps, which means multiple releases can be rolled out in a week.
However, the move to the edge poses challenges for scaling cloud-native applications. If you’re used to an environment that consists of a few centralized data centers, you’ll know that human operators can feasibly determine the optimum performance conditions of the virtual network functions (VNF) that make up the application.
As the environment disaggregates into thousands of small sites, it’s a different story altogether with more sophisticated needs that need to be catered for. That’s because each of the small sites have slightly different operational characteristics. Human operators simply don’t have the bandwidth to cope. This is where machine learning is required. Unsupervised learning algorithms can run all the individual components through a pre-production cycle to evaluate how they will behave in a production site. This gives operations staff the confidence that the VNF being tested will work as desired at the edge.
Using AI to removing the trouble in troubleshooting
Another area where AI and automation can add value is in troubleshooting within cloud-native environments. Let’s say for instance, the VNF for a cloud-native application running at an edge location is performing at a level below other instances of the application. The initial fact to establish is whether there is actually a problem because some performance variation between applications isn’t unusual.
Answering the question requires a determination of the normal range of VNF performance values in actual operation. One way of finding that out would be for a person to take readings of a large number of VNF instances and use these readings to calculate acceptable key performance indicator values. This approach isn’t recommended for a few reasons. It takes a long time, it’s error prone and it has to be repeated every time there are software upgrades, component replacements or traffic pattern variations.
AI, on the other hand, works differently. It can determine KPIs quicker and more accurately, while also adjusting the KPI values as needed when parameters change. This all happens without the need for human intervention. Once AI determines the KPI values, automation takes over. Using an automated tool, it’s possible to continuously monitor performance and identify underperforming VNFs.
From there, the information can be reviewed to see whether a new VNF or a new physical server is needed. The powerful combination of AI and automation ensures SLA compliance is watertight and also reduces the burden on human operators.
A future at the edge
A new normal is being created around us. Service providers are increasing their use of edge-oriented architectures and as such, IT groups are using AI and machine learning to find new ways to optimize network operations, troubleshoot underperforming VNFs and ensure SLA compliance at scale.
To accelerate the journey to this AI-driven future at the edge, technologies are improving at pace, enabling new benefits. For instance, various systems and devices can provide high-fidelity, high-frequency telemetry that can be analysed, highly scalable message buses such as Kafka and Redis can capture and process that telemetry, and compute capacity and AI frameworks such as TensorFlow and PyTorch create models from the raw telemetry streams. It’s a robust set of tools that can see in real time if production systems are running as they should and also find and remediate problems that arise in operations.
All said, there’s plenty for service providers to explore in order to gain an advantage with edge networks and they should see the move towards the edge as an opportunity. By deploying machine learning and automation, they can tighten up their processes to reduce time consuming workloads. And they can also change workflows all together by using new insights they could never access before.
- We've featured the best in online storage.
Julius Francis is the Senior Director of Product Management & Marketing at Juniper Networks. He provides leadership, vision, and strategy to plan and execute Juniper’s Cloud, Automation & Disaggregation business growth. Julius drive initiatives for Juniper’s early wins in 5G, Cloud, AI by developing an integrated vision, marketing strategy, messages, stories, positioning, sales enablement and triggering joint-innovation showcases, virtual/in-persons events and roadshows.
Russia’s shadow war against Europe has begun as cyber attacks abusing Microsoft infrastructure increase
Corel is giving Citrix users free licenses worth thousands of dollars in a bid to migrate them to Parallel RAS — Canadian developer highlights lesser complexity, single licensing model and lower costs