How to navigate the new age of data integration
Not as easy as it seems
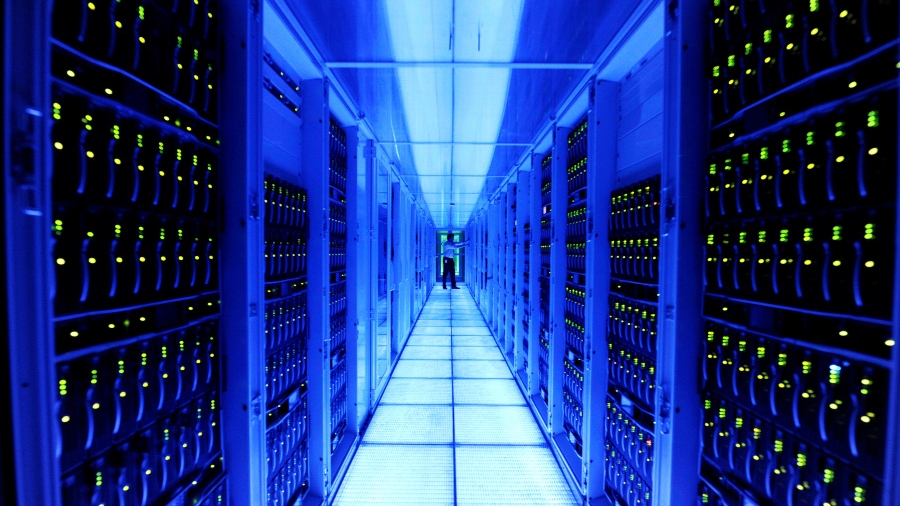
Most companies have come to understand that there is value in big data, yet many continue to struggle today with all of the market buzz surrounding the term. This is especially true for nuances like integration and optimization.
Many organizations know they should be doing something to that end, but aren't sure what. It's obvious that you want your big data project, which typically require a good amount of integration, to bring most value and best performance at the lowest total cost – but how do you get there?
This question gets an added layer of confusion when we consider that the data integration landscape is undergoing some change. Today, inexpensive and powerful platform alternatives are available, making even yesterday's best data integration practices in need of re-evaluation for big data types, processing and systems. A few aspects of the ever-changing landscape:
Explosion of need for analytics: As analytics takes center stage, organizations are seeking more headroom for analytics and questioning every non-analytical function that is run.
Faster delivery mechanisms: One issue for many companies comes in regards to data integration runtime performance – whether because of missing SLA's or updates that are too slow. Yet there is the desire for real-time, or at least near-real-time, updates.
New data types: Companies must integrate non-traditional forms of data from internal and external sources.
More data volume: And as data volumes continue to explode – even within existing sources, data archiving and purging data can become an issue, along with the need to offload dormant data.
Are you a pro? Subscribe to our newsletter
Sign up to the TechRadar Pro newsletter to get all the top news, opinion, features and guidance your business needs to succeed!
What to do?
So how do companies address data integration in the face of these issues and changes? In a nutshell, they need to do their due diligence. Data integration is a process that can't be rushed, and one that comes with a lot questions.
A few questions that are usually front of mind these days include: How should I offload to Hadoop? What do I offload, exactly? And, more importantly, how do I go about planning this out? The issue is not whether or not to offload some of the extract, transform and load (ETL) processes, as doing so helps form the lowest total cost of ownership (TCO) calculation. Instead, organizations need to look at the environment they have, as all environments are different, and look at all viable alternatives to reduce the cost of the data integration process.
This analysis and assessment should be done using a fact-based approach to identifying, tuning and moving data integration processes to improve efficiency and meet business requirements…all while recapturing valuable system resources for high impact business analytics.
This process helps to decide whether to modify ETL code, re-architect ETL processes, and/or extend architecture with systems such as Hadoop. In many cases, organizations need some help understanding the best data integration solution … not only for today, but for the future as it is all about using the right piece of the data ecosystem for the right job.
For instance, many companies want to expand their environment with Hadoop in order to offload ETL processes. This was the case in a recent client engagement. But once the client was encouraged to do their due diligence and assess the ETL code and analytic environment, it was also discovered that a significant amount of ETL code was inefficient. As a result, we recommended the company modify that ETL code in addition to offloading some of the lower-value ETL work. This dual-pronged approach freed up capacity for additional analytics, and shows why you can't just pick a solution without doing your homework.
The lesson: Data integration optimization is important, but it's not easy. Make sure you do all the legwork to ensure you're getting the most bang for your buck.
- David R. Schiller is CCP at Teradata Products and Services Marketing