Techradar pro survey: tell us about your office applications and grab an office suite worth £45 for free
Word processing, crunching numbers and presentations
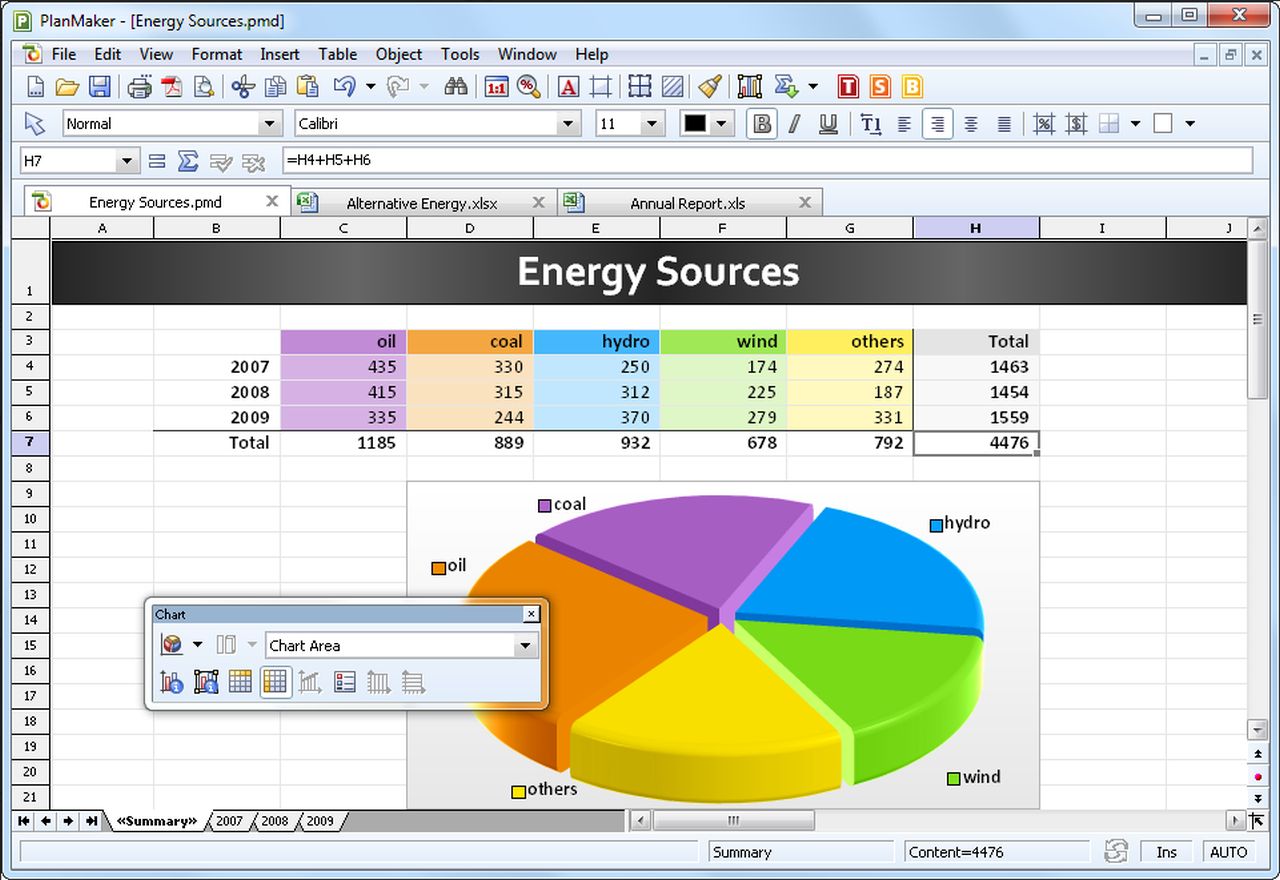
Techradar pro has teamed up with SoftMaker, a company that publishes an office application package, to find out how our readers use office applications, whether for business or otherwise.
Whether you're a fan of word processors, an afficionado of Excel or go bonkers on presentation packages, let us know by completing our survey below.
And unlike competitions, everyone who fills in the survey is a winner! You get a free copy of SoftMaker Office 2012 worth £45, which is roughly equivalent to Microsoft's Office Home and Student 2013.
Another good news is that everyone – not just UK residents – can can take part in the survey. The survey will close at 11.59pm on December 11 2015, and the codes will be sent shortly afterwards.
SoftMaker 2016 – which is also sold as Ashampoo Office 2016 – is the latest version of the business application suite, and costs as little as $39.95 (£21) if you upgrade from Office 2012.
You will also be able to run it on up to three computers.
Instructions for the reader when they receive the unlock codes are:
Are you a pro? Subscribe to our newsletter
Sign up to the TechRadar Pro newsletter to get all the top news, opinion, features and guidance your business needs to succeed!
- Go to http://www.softmaker.com/unlock
- Fill out the registration form
- Check your inbox for an email from SoftMaker with the download address and your personal serial number.
PS: The 2012 version uses dual code: unlock-code + serial number (was done for copy protection and technical reasons). So the user receives unlock code and after registration (name + e-mail + unlock-code) the system here generates the serial number (the user receives e-mail with serial number and download link).
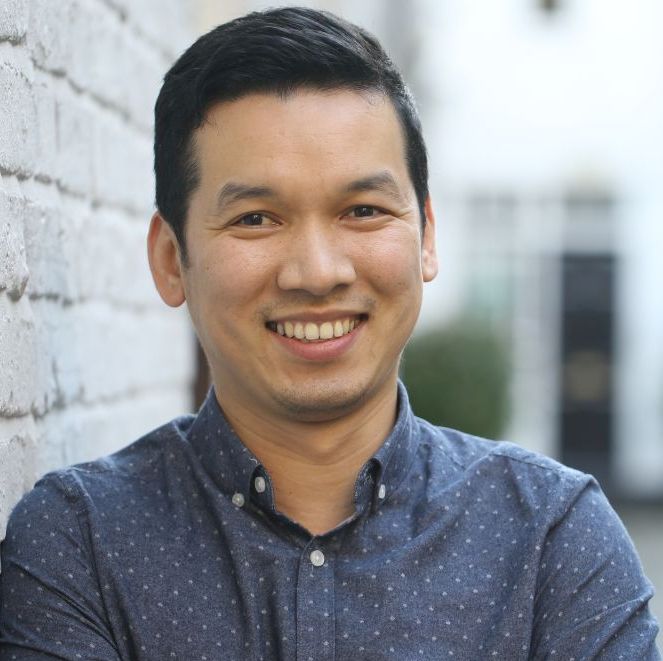
Désiré has been musing and writing about technology during a career spanning four decades. He dabbled in website builders and web hosting when DHTML and frames were in vogue and started narrating about the impact of technology on society just before the start of the Y2K hysteria at the turn of the last millennium.