"The kitchen sink of the atomic world": Affordable material could pay crucial role in reducing AI energy use by a factor of 90 — memristors may help current AI system mimic biological neural networks function
Researchers want to give AI chips a sense of time
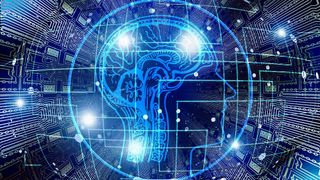
Researchers at the University of Michigan have developed a memristor with a tunable relaxation time, potentially leading to more efficient artificial neural networks capable of time-dependent information processing.
Published in Nature Electronics, the study highlights the potential of memristors, electronic components that function as memory devices and can retain their resistance state even when the power is turned off.
Memristors work by mimicking key aspects of the way artificial and biological neural networks function without external memory. This property could significantly reduce energy needs in AI, which is important as the technology’s electricity consumption is projected to rise significantly in the coming years.
Kitchen sink of the atomic world
“Right now, there’s a lot of interest in AI, but to process bigger and more interesting data, the approach is to increase the network size. That’s not very efficient,” said Wei Lu, the James R. Mellor Professor of Engineering at U-M and co-corresponding author of the study with John Heron, U-M associate professor of materials science and engineering.
The research team achieved tunable relaxation times for memristors by varying the base material ratios in the superconductor YBCO. This superconductor, made of yttrium, barium, carbon, and oxygen, has a crystal structure that guides the organization of other oxides in the memristor material.
Heron refers to this entropy-stabilized oxide as the “kitchen sink of the atomic world” because the more elements that get added to it, the more stable it becomes.
By changing the ratios of the oxides, the team achieved time constants ranging from 159 to 278 nanoseconds. The team then built a simple memristor network that was capable of learning to recognize the sounds of numbers zero to nine. Once trained, the network could identify each number even before the audio input was complete.
Are you a pro? Subscribe to our newsletter
Sign up to the TechRadar Pro newsletter to get all the top news, opinion, features and guidance your business needs to succeed!
The study's findings represent a significant step forward in neuromorphic computing, as memristor-based networks have the potential to significantly improve energy efficiency in AI chips by a factor of 90 compared to current GPU technology. “So far, it’s a vision, but I think there are pathways to making these materials scalable and affordable,” Heron said. “These materials are earth-abundant, nontoxic, cheap and you can almost spray them on.”
More from TechRadar Pro
Wayne Williams is a freelancer writing news for TechRadar Pro. He has been writing about computers, technology, and the web for 30 years. In that time he wrote for most of the UK’s PC magazines, and launched, edited and published a number of them too.