The key to unleashing generative AI’s potential
In-house models could unlock generative AI's potential
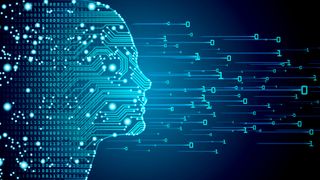
The pace of AI advancement has increased significantly in the last year. The arrival of OpenAI’s ChatGPT in November 2022 attracted significant consumer attention, with organizations quickly realizing the earning potential that could come from implementing generative AI applications into their offering. In tandem, technology leaders have invested significantly in the large-language models (LLMs) that power generative AI, with the rate at which organizations produced AI models increasing by 411% in the last year.
As this trend continues its upward trajectory, many executives are considering how to bring generative AI into their organization for the very first time. Leaders are looking to cut through the noise and create a generative AI strategy that is cost effective, delivers business value, and protects their data. Could building models in house be the answer?
Bringing generative AI into the fold
For most businesses, AI itself is not necessarily new, but rather an existing tool that has become a higher priority in the last year. For example, in 2022, before the advent of ChatGPT, data from MIT Technology Review demonstrated that 94% of organizations were using AI in some way. That same survey also shows that only 14% of these organizations aimed to achieve the implementation of “enterprise-wide AI,” defined as having AI being a critical part of at least five core functions, by 2025. As such, during this period, the use of AI was rarely standardized across organizations, and significant benefits were therefore left untapped.
Since then, generative AI has become a must-have tool for many organizations, and it’s easy to see why. Recent research from McKinsey and Company estimates that generative AI could boost the global economy by $2.6 trillion to $4.4 trillion annually (£2 trillion to £3.4 trillion). Generative AI is set to have a significant impact across all sectors, in small and large businesses alike. To unlock its full potential, executives need to implement a robust generative AI strategy that comprises well-structured models, highly-skilled teams, and top-notch data infrastructure.
Richard Shaw is the AVP Field Engineering for Northern Europe at Databricks, the data and AI company.
All AI is not created equally
Powered by the potential of newly emerging use cases, such as image generation and personalized content creation, generative AI is well on its way to becoming a generalized capability integrated into the fabric of organizations. Technology teams rarely have to sell its value to other departments, instead seeing significant demand across all aspects of their organization. Often therefore, the question is not about whether generative AI has value, but rather how it can be implemented efficiently, safely and effectively.
Bigger models are not always more reliable, especially when it comes to data governance. Some of the most popular generative AI tools, like ChatGPT and Google’s Bard, require that users grant access to data collection by the model’s creator. This personal data is then used for further training of the model, which can be especially concerning in an enterprise context. For example, if an employee inputs sensitive company data into a third-party generative AI application, this data may form the basis of the chatbot’s response to another user later down the line. Smaller, open-source models can mitigate many of these concerns. When using a small, in-house model, organisations never have to share their data with a third party, keeping it secure. Over time, this data can be leveraged to train the model, greatly improving its performance and reducing the likelihood of inaccurate or irrelevant outputs.
Bigger does not always mean ‘more accurate’
The generation of inaccurate or unreliable outputs is a further concern for many organizations. Huge datasets are required for the training of the industry’s largest LLMs, so third-party organizations often scrape the web to collect data. Inevitably, this data can therefore be tainted by the wealth of factual inaccuracies present online. Equally, the scale of this training can present additional concerns in the ESG arena. Researchers at the University of Massachusetts found that training the average large AI model can emit more than 626,000 pounds (284kg) of carbon dioxide equivalent, which represents nearly five times the lifetime emissions of the average car.
More efficient smaller models, which can mitigate concerns around inaccuracy, may be a promising alternative. Organizations can choose from the range of open source models on the market, which are often tailored to very specific use cases. This differs from the larger models developed by third-party providers, which are trained on data from the web and their users. Such a large dataset may be useful for consumer use, but within the enterprise, it will be far more useful for a model to know about internal concepts and data. Small models bring that unique knowledge, thanks to the fact that they are trained on the organization's proprietary data.
Small, open-source models are set to rival the performance of large models and allow practitioners to innovate, share and collaborate. As organizations increasingly buy into the value of generative AI, it is important that they consider how to leverage generative AI tools effectively and responsibly. Indeed, there are many important use cases for the largest models on the market, but for many organizations, it is worth looking at smaller models. When building in house, organizations enjoy greater control over their sensitive data, reduced costs associated with generative AI deployment, more accurate outputs, and a greener approach. To ride the generative AI wave without compromising on profitability, efficiency, and security, small models are the answer.
Are you a pro? Subscribe to our newsletter
Sign up to the TechRadar Pro newsletter to get all the top news, opinion, features and guidance your business needs to succeed!
Richard Shaw is the AVP Field Engineering for Northern Europe at Databricks, the data and AI company.