Why we need to trust AI before we can optimize it for business
Avoid inadvertently sharing sensitive data
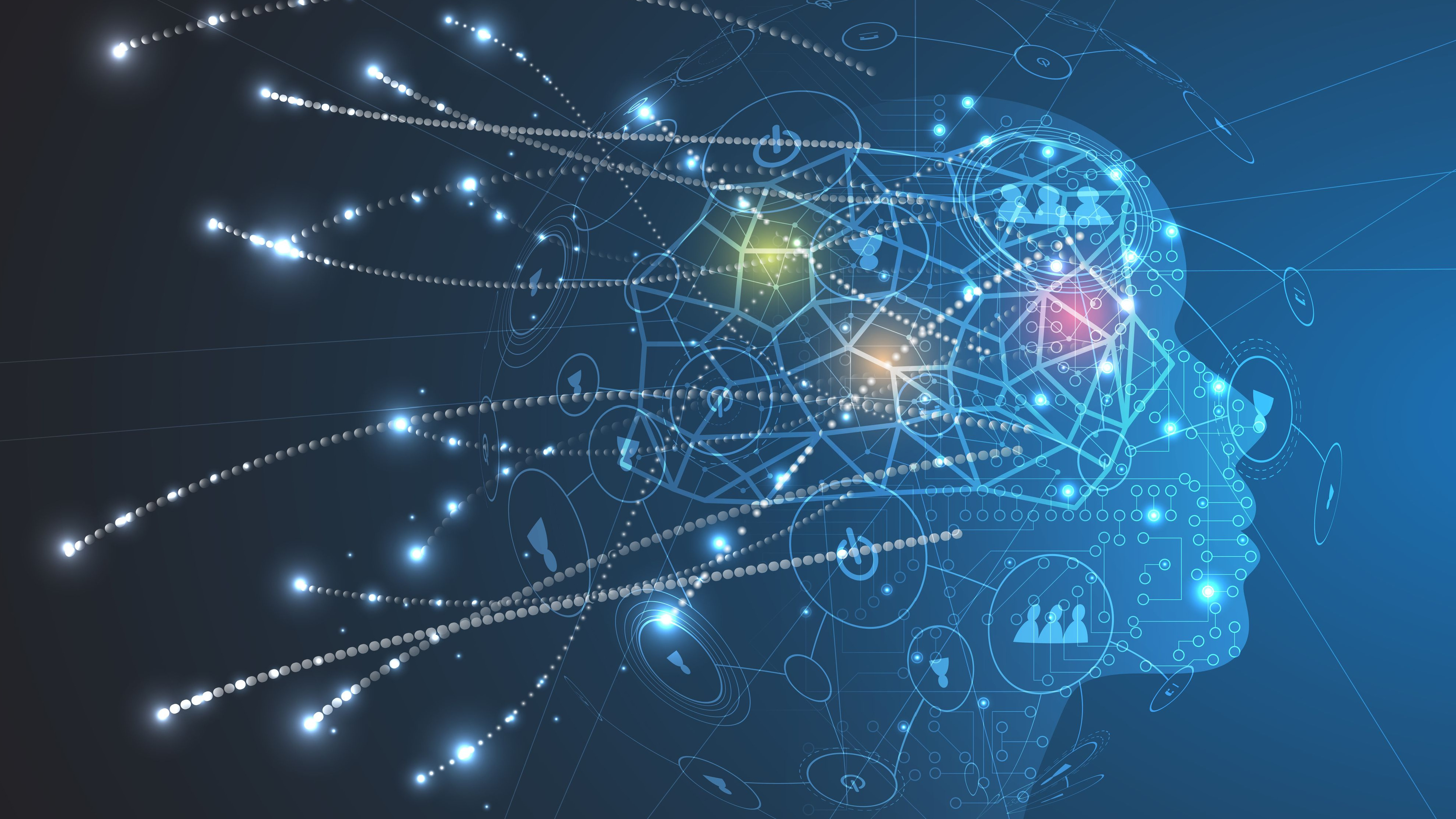
Large language model (LLM)-based AI platforms are the talk of many boardrooms. Due to the breakout success of OpenAI’s ChatGPT, organizations across the globe are discussing how they can leverage the technology to their advantage. The ability to ask a powerful AI interface questions in natural language and receive answers back in plain English will supercharge productivity in coding, customer service, content creation and many more use cases. Yet with any great leap forward in technology, there are risks. And only those organisations able to understand these risks and manage them effectively will be the true winners.
In short, organizations must not only be able to trust the output of LLM-based or generative AI, they need to be sure it won’t inadvertently share important or sensitive data with others. This will require a more considered approach than simply using an off-the-shelf service like ChatGPT can offer, and for the data that is fed into LLMs to not only be relevant, but accessible – regardless of where it resides.
Why we can’t trust LLMs fully
There are two main business risks with LLMs: governance and accuracy. From a governance perspective, data privacy is a critical concern for global organizations. They can’t afford potentially sensitive proprietary information getting into the public domain, or highly regulated personal information to be leaked. Yet the risk with using commercial generative AI tools is exactly that.
Although ChatGPT explicitly warns users not to share sensitive information with it, some engineers at a tech company did not get the memo and used the tool to help optimize private proprietary source code. By using tools like ChatGPT, all of that information has now been retained by OpenAI to train its LLM model – the data has effectively been ‘leaked’. It is partly due to privacy concerns like these which led Italy’s data protection regulator to temporarily ban ChatGPT.
The second major concern with LLMs currently is the accuracy of their output. They are trained on publicly available data scraped in huge volumes from the internet. Not only might this violate third party intellectual property rights but that will make them relevant for some queries, but not necessarily aware of the business context for a specific corporate user. The result is that, when applied to this data, LLMs will often create hallucinated responses that sound plausible but are in fact false. Alternatively, LLMs may return factually correct responses which are simply not contextually relevant to the query, as they simply don’t understand the nuanced background of the data and the request.
The bottom line is that commercial LLMs can’t always be trusted to provide 100% accurate and truthful answers, as they’re not trained on proprietary business data. And if any users accidentally share anything private to the organization, there could be serious compliance repercussions. So, what’s the alternative?
EMEA Field CTO, Cloudera
The future’s open
If LLM-based AI is to be trusted by the organization, it must be trained on their own proprietary data. But how? The answer is powerful prototypes which tap the wisdom of the open source community and are being released with growing frequency on sites such as HuggingFace. These pre-trained foundation models are easily accessible, and the technology is sound: even Google has tacitly acknowledged they represent a serious threat to the tech giants’ business models.
There are two benefits for organizations looking to use these models to accelerate development of their own LLMs. It would help eliminate privacy concerns, as all interactions with the tool would be kept in house, and it has no scope to effectively leak data. And it would enable the model to be trained with proprietary enterprise data to ensure accurate, contextual responses, that haven’t ingested data from outside an organization. No hallucinations. All without the need to spend significant money on R&D or hosting infrastructure. That’s true Enterprise AI.
However, to turn this from vision into reality, organizations need to build on solid foundations. That means having a unified data platform capable of unlocking data from across the enterprise—from on-premises datacenters to disparate cloud environments and that enforces strict governance to ensure data compliance. With this foundation in place, organizations can build enterprise AI applications, powered by an open source LLM of their choice—trained on their own internally hosted data.
That’s the way to extract real value from generative AI—avoiding data privacy risks and hallucinated responses, and reducing the time and cost associated with training LLMs.
Evolution, not revolution
Whilst there are clear benefits of AI for analytics, organizations must have a thorough understanding of the risks involved before they implement it. Ensuring trust in data through strong governance and interpretability is vital, but it relies on giving AI access to the latest in-house data and the ability to respond to any changes to that data set in real-time.
Organizations need to foster a data-driven culture to get the most out of AI. Seeing data as a product will help to generate reliable data sources that are of the necessary quality to enable AI to thrive. Ultimately, if AI innovation is to succeed, organizations must build from a foundation of trust, governance and data accessibility.
Sign up to the TechRadar Pro newsletter to get all the top news, opinion, features and guidance your business needs to succeed!
Chris Royles is Field CTO for EMEA at Cloudera.