Why clean data underpins all AI-enabled decision intelligence
Clean data underpins the ability to react to customer needs
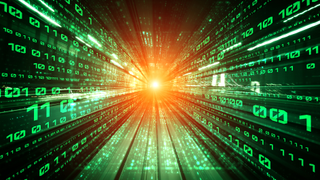
Global economic volatility, new regulations, and changing customer and citizen expectations are putting organizational leaders under ever-greater pressure to deliver results. According to Gartner, 65% of respondents say the decisions they make are more complex than two years ago, with 53% facing more pressure to explain or justify decision making. Which is a clear indicator as to why 80% of executives think that automation can be applied to any business decision. Individuals and businesses are under pressure to make fast, consistent and fact-based decisions, so called ‘decision intelligence’.
Although AI and machine learning (ML) have clear scope for improved decision making and value-add, organizations are putting the cart before the horse. They are asking and expecting AI and ML to solve problems before considering that there’s more than just a few complicated strings of code at the heart of every automation challenge; it’s the input data.
A poodle walks into an Italian restaurant... paw data is the punchline
AI models are only as good as the data inputted into them. Which is why every data scientist’s ambition is to work with trustworthy, quality data. For instance, if you build a classifier to distinguish between photos of cocker spaniels and poodles, data scientists would ideally like an input image dataset certified by breeders. If that was unavailable, one may try to use the internet, but this could be subject to entry error and images could be mislabeled.
Another data challenge is understanding what a data point truly is. In many countries, it is common to find organizations named after individuals. For example, self-employed medical professionals run a business registered with their own name – so models struggle to differentiate a business from an individual. In this case, medical qualifications are often included as a name suffix to make identifying the entity as an individual easier. In a similar vein, in Italy, it is common for Italian restaurants to be named after individuals. These names have no obvious characteristics to help a model detect what type of business it is without first supplying additional information. This missing data is known as context and the issue at play is what we call ‘the Italian restaurant problem’.
There is also the challenge of user-entered data or using different names for the same entity. Take the name John Andrew Lewis’. He may appear as J.A. Lewis; John Lewis; Lewis, John Andrew and so on. Similarly, businesses can appear as their full legal name (e.g., Quantexa Limited) or by a heavily abbreviated version (e.g., Quantexa, or even Q). It is essential that the algorithm can learn from a full range of different names and formats.
The scale of creating good datasets becomes clear when we combine these examples. Knowing that John Lewis is a single person is obviously essential for decision making across all organizations from banking to the public sector, yet is a fiendishly complicated task. It’s even more difficult when we consider how many people, and organizations, are called John Lewis. Understanding this, is called context.
Dan Higgins is Chief Product Officer at Quantexa.
Clean, contextual data is key to unravelling the data paradox
Research by Quantexa suggests that fewer than a quarter of IT decision makers believe their organization trusts the accuracy of the data available to them. Indeed, one in nine customer records is a duplicate – meaning that countless organizations cannot tell the difference between J.A. Lewis, John Lewis and John Andrew Lewis. Despite being an organization’s greatest asset, data can paradoxically become the biggest barrier to transformation efforts.
Dell Technologies 2020 Digital Transformation Index revealed that “data overload and the inability to extract insights from data” is the third highest barrier to digital transformation, up from 11th place in 2016. A problem that increases as the expanding on-demand economy creates yet more data, across different locations, further fueling expectations that data is processed, understood and acted upon.
In a banking context, an organization may have data relating to the same customer arriving from various CRM and product-specific systems across different divisions. For example, a small business bank and retail bank. This is the very nature of siloed data. There may be linkage via transactions between them but overall the graph is sparsely linked and lacks context for analysis, data science or operational decision-making.
To get a complete view of a customer and to do so at the scale needed by modern organizations, simply eyeballing the data and confirming J.A. Lewis and John Lewis are the same person is slow, laborious and still prone to error. Traditional technologies such as Master Data Management (MDM) generally haven’t been able to deliver complete views of different entities like an individual customer. However, there is a category of product that does – entity resolution.
Entity resolution is the answer to providing rich context
Entity resolution parses, cleans and normalizes data and uses sophisticated AI and Machine Learning models to infer all the different ways of reliably identifying an entity. It clusters together records relating to each entity; compiles a set of attributes for each entity; and finally creates a set of labelled links between entities and source records. It’s dramatically more effective than the traditional record-to-record matching typically used by MDM systems.
Rather than trying to link all the source records directly to each other, organizations can add new entity nodes that act as a nexus for linking real world data together. High quality entity resolution means not only your own data can be linked, but also high value external data such as corporate registry information that in the past would have been difficult to match reliably.
Quantexa’s same research shows that just over one in four (27%) organizations utilize entity resolution technology to master data and provide the ability to create the analytical context needed for timely and effective decision making. With data duplicates pervasive across organizations’ data lakes, warehouses and databases, organizations are being laden down with time-consuming data reconciliation and remediation. Entity resolution technology is vital to decision intelligence. Without it, any decision making will be made on inaccurate or incomplete data.
Why all decision intelligence leads back to clean data
Organizations are overwhelmingly aware of the need to improve decision-making with the use of data. To those uninitiated with the complexities rife with dissolving data siloes, these issues can seem basic. Yet different iterations of a name, changes in address or multiple phone numbers are creating these replications. Duplicated data can cause a domino effect in inefficiency and confidence in decision making. This becomes a costly waste of resources across data, IT, and business teams and it stops businesses from creating the necessary agility and resilience to being able to identify risk fast and serve customers at the highest levels. To achieve decision intelligence, first start with your data.
Are you a pro? Subscribe to our newsletter
Sign up to the TechRadar Pro newsletter to get all the top news, opinion, features and guidance your business needs to succeed!
Dan Higgins is Chief Product Officer at Quantexa. Prior to joining Quantexa Dan spent over two decades at EY, serving a range of leadership roles including Global Consulting Executive, Global Client Service Partner.