The unintended consequences of the data boom: ageing data
Realising real-time data
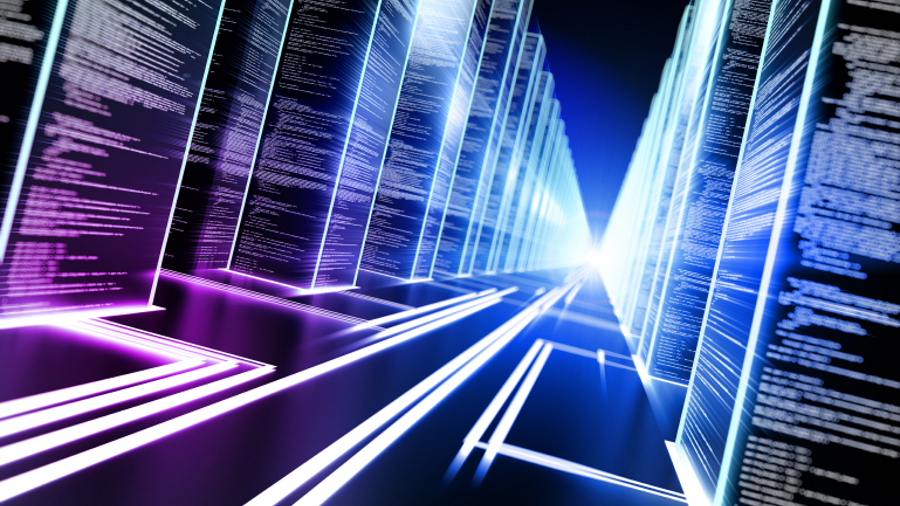
Data is central to every technology we touch, and as we go about our daily lives we consume and generate data at an incredible rate. Even something as simple as paying for lunch in a café with a debit card generates data.
Recently, the volumes of data that we're producing has exploded, so we're no longer talking about data easily managed in a spreadsheet, but about big data, which drives the need for sophisticatedintelligence systems.
Big data evangelists have been touting the benefits of collecting more and more data, citing that size is good and bigger is better. This tidal wave of data was designed to make us smarter, enable us to make near real-time decisions and maybe even predict future behaviours.
However these seductive claims about big data hide the fact that if collected within the current infrastructure at most companies, the data deluge is more likely to make an enterprise slower, less responsive and – in the long term – less 'intelligent'.
Why is this happening?
It's because processing terabytes of information on the already-taxed legacy systems many businesses run on takes longer and longer as data volumes increase.
As a result, the data organisations end up using for business-critical reports, or to test new applications, isn't real-time at all, it's old and it's only getting older as the following types of additional IT requirements exacerbate the problem:
Data migration: Businesses often run a large number of enterprise apps (those in the banking industry can count them in the thousands), and they have complex processes for data to complete before it gets to the business intelligence software for analysis.
Sign up to the TechRadar Pro newsletter to get all the top news, opinion, features and guidance your business needs to succeed!
The data must move from applications into operational data stores before it ends up in a data warehouse. There is usually a limited window of time in which this process needs to be completed, and when data volumes were smaller, it was a fairly manageable task.
If one of these projects is going on concurrently with BI projects, it's possible that suddenly, rather than having day-old data in the reporting environment, analysts end up with data that was in some cases weeks old. One of our customers calculated the cost of this wait for old data at 50% of their BI investment.
Database replication: Many large organisations need to manage multiple instances of single databases. These databases are used for a multitude of business processes, including test and development, quality assurance (QA), training, and back-up and disaster recovery.
As a result, on average, each database is replicated eight to ten times. These replications act like a sea anchor on any business intelligence system; it takes huge amounts of time and effort to crunch through the replicated data, producing a drag on the whole process.
Data masking: New EU regulations will soon require any organisation that deals with customer data to mask the sensitive data they collect no matter if it is used for development, testing and QA, or if it's simply stored and monitored for business intelligence purposes.
While the process of data masking is straightforward, organizations often have trouble with data delivery. As the organizations are required to mask not just one set of data, but every copy made, these projects stack up at a rapid rate.
A host of compromises
So, what's the solution to this aging data problem? Traditionally, in most cases it involves a lot of compromises. For example, some companies try to address this problem by choosing to work with smaller subsets of data.
Other organizations prioritise which data really needs to be real-time and which can be delivered weekly, monthly or quarterly. However, by moving away from legacy architectures and prioritizing the integrity of their data, many organizations are finding that they're able to avoid taking those compromising measures.
To prioritise data, first organizations need to make that data agile. Techniques of virtualization are now being applied to entire applications stacks, which allows even the most expansive data sets to take up a fraction of the space, meaning that the data can now be delivered anywhere within the organization within minutes.
Organisations that have put their data first by deploying virtualization technology have seen processing times shrink dramatically from weeks to a few hours, meaning that the data doesn't have the chance to become stale that it once had. One of our clients was able improve performance to such an extent that the data arrived in minutes rather than days.
Most IT leaders already understand the agility and mobility benefits virtualisation can provide with their servers. However, by expanding the possibilities for virtualisation to the application stack, organisations can begin to achieve the types of insight and business intelligence that 'big data' has always promised, whilst still being able to develop, test and deploy new applications efficiently.
Ageing data makes us slower, not smarter; but with the right infrastructure in place, the big data boast - mine's bigger than yours - might finally start to acquire some real meaning.
- Iain Chidgey has over 20 years of experience in the IT Industry and is currently the EMEA VP and General Manager of Delphix, a leading global provider of agile data management platform to enterprise companies all over the world.