AI: Navigating the perils of data mismanagement
Data is the fuel that powers AI
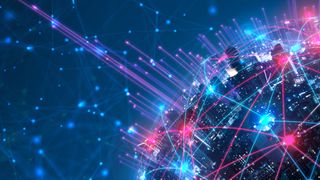
The prevalence of AI is growing in today’s modern world. Since being considered an obscure, futuristic concept as little as ten years ago, it is now making headlines globally with advanced generative AI tools such as ChatGPT entering the public discourse. Bodies and regulations – such as the Council of Europe and Artificial Intelligence, and pro-innovation regulations around AI in the UK - now exist solely to manage the technology.
AI has also become increasingly entrenched in businesses. A recent MIT report, titled “CIO Vision 2025: Bridging the gap between BI and AI” – which surveyed CIOs, CTOs, Chief Data and Analytics Officers, and other senior and data technology executives - found that well over half of executives expect AI use to be widespread or critical in business functions by 2025. As such, we can expect AI’s business use cases to expand.
However, this journey will not necessarily be smooth sailing. There are hurdles that organizations must overcome to ensure AI progresses. To tap into the full benefits AI offers, organizations must first ground themselves in their data.
Data: the lifeblood of AI
Data really is the fuel that powers AI. Without data, AI cannot learn, and without learning, it cannot become intelligent. It is somewhat paradoxical, then, that data can also be AI’s undoing. The MIT report found that 72% of C-level respondents believe that problems with data management will jeopardize future AI achievement. Imagine a car that runs on diesel sat by a pump in a petrol station.
If its driver reaches for the correct pump and feeds it what it needs – diesel – it will run perfectly. But if the driver is careless and reaches for the wrong pump, giving the car petrol, then that car won’t be going anywhere. It’s the same with AI. The right kind of data, that is good quality and easily shareable, will fuel it. Data that has been handled carelessly, and that has errors or duplications, will stop AI in its tracks.
Robin Sutara is the Field Chief Technology Officer at Databricks. Prior to Databricks, she was the Chief Data Officer at Microsoft UK.
As we can see from the results of the MIT report, data mismanagement is a widespread problem. Part of this is down to the fact that many organizations have not yet built a robust data culture. Whether this be due to a lack of leadership buy-in, a lack of skills, or a lack of understanding of the value data can hold, a weak data strategy and weak data culture can seriously impact AI.
Additionally, and often part and parcel of a weak data culture, many organizations currently operate on legacy data architectures, such as data warehouses, which are costly and resource-intensive to manage. The complexity of these legacy structures can make delivering a data strategy challenging – they can cause information silos to form, and prevent data from being easily distributed. Furthermore, inaccurate datasets that contain duplicated or outdated information may be shared, causing larger problems down the line. In these conditions, it’s near impossible to scale up the use of AI across a business.
Embracing a modern approach to data
To truly begin to move the dial when it comes to AI, organizations must first look at their data and how they, as a business, handle it. There are many elements that need attention when building a data culture, from securing leader buy-in to employing or growing the right skills. But perhaps the best place to start is with the foundations. In the policy framework for the National Data Strategy, the first focus is to “establish foundations'', which is sound advice. Organizations should look to build strong, modern data foundations from the outset – such as a data lakehouse, which brings together the best of data lakes and warehouses, removing much of the complexity typically associated with these legacy architectures and enabling the timely flow of accurate data.
Modern architectures such as this reduce the number of different platforms needed, easily storing data for AI and ML use cases and making rolling out a data strategy far easier. The MIT report found that, when asked which aspects of their company’s data strategy is most in need of improvement in order to support their AI goals, speed of data processing was a priority for respondents. With this in mind, employing a platform that easily stores data for analysis, and allows for the timely and accurate flow of data, will be key in laying down the path for successfully scaling AI and ML use cases.
The value of being open
Crucially, the MIT report also found that the vast majority of respondents recognize the value that operating on open standards provides for AI development. This is because one of the main barriers to organizations succeeding with their data – and, therefore, with their AI strategies - is challenges around sharing data. There are many frustrations around how data can be shared and, once it has been shared, there are real challenges with maintaining value. Waiting for data to be shared can be slow and, in the time that passes, conditions may change and momentum may stall – rendering the data useless. Data sharing shouldn’t be a barrier to innovation in this way.
Fortunately, we’re seeing a worldwide shift towards open-source tools and technology. Open-source makes accessing, distributing, reusing and modifying datasets quick and simple. As such, being willing to embrace open-source is key for any organisation seeking to harness the value of their data to drive AI. Adopting a single platform – such as a data lakehouse – that is able to securely share data in real time, will be key. To complement this, there will need to be a focus on encouraging an organisational culture shift to being “data positive” and embracing openness. This will help drive the success of AI across the enterprise.
As an increasing number of organizations seek to accelerate, and expand, the use of AI across the business, data cannot be overlooked. Adopting a modern, open approach to data management – fueled by the building of a positive, robust data culture – will lay the groundwork for AI to take off, and bring the business with it.
Are you a pro? Subscribe to our newsletter
Sign up to the TechRadar Pro newsletter to get all the top news, opinion, features and guidance your business needs to succeed!
Robin Sutara, Field CTO, Databricks.