AI can now play Minecraft just as well as you - here’s why that matters
OpenAI has developed a neural network capable of playing Minecraft to a human-level standard
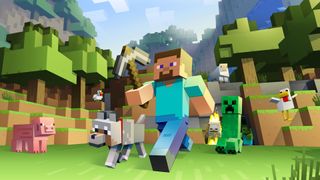
Experts at OpenAI have trained a neural network to play Minecraft to an equally high standard as human players.
The AI model was trained on 70,000 hours of miscellaneous in-game footage, supplemented with a small database of videos in which specific in-game tasks were performed, with the keyboard and mouse inputs also recorded.
After fine-tuning, OpenAI found the model was able to perform all manner of skills, from swimming to hunting for animals and consuming their meat. It also grasped the “pillar jump”, a move whereby the player places a block of material below themselves in mid-air in order to gain elevation.
Perhaps most impressive, the AI was able to craft diamond tools (requiring a long string of actions to be executed in sequence), which OpenAI described as an “unprecedented” achievement for a computer agent.
- Check out our list of the best Minecraft server hosting
An AI breakthrough?
The significance of the Minecraft project is that it demonstrates the efficacy of a new technique deployed by OpenAI in the training of AI models - called Video PreTraining (VPT) - which the company says could accelerate the development of “general computer-using agents”.
Historically, the difficulty with using raw video as a source for training AI models has been that that what has happened is simple enough to understand, but not necessarily how. In effect, the AI model would absorb the desired outcomes, but have no grasp of the input combinations required to reach them.
With VPT, however, OpenAI pairs a large video dataset drawn down from public web sources with a carefully curated pool of footage labelled with the relevant keyboard and mouse movements to establish the foundational model.
Are you a pro? Subscribe to our newsletter
Sign up to the TechRadar Pro newsletter to get all the top news, opinion, features and guidance your business needs to succeed!
To fine tune the base model, the team then plugs in smaller datasets designed to teach specific tasks. In this specific context, OpenAI used footage of players performing early-game actions, such as cutting down trees and building crafting tables, which is said to have yielded a “massive improvement” in the reliability with which the model was able to perform these tasks.
Another technique involves “rewarding” the AI model for achieving each step in a sequence of tasks, a practice known as reinforcement learning. This process is what allowed the neural network to collect all the ingredients for a diamond pickaxe with a human-level success rate.
“VPT paves the path toward allowing agents to learn to act by watching the vast numbers of videos on the internet. Compared to generative video modeling or contrastive methods that would only yield representational priors, VPT offers the exciting possibility of directly learning large-scale behavioral priors in more domains than just language,” explained OpenAI in a blog post.
“While we only experiment in Minecraft, the game is very open-ended and the native human interface (mouse and keyboard) is very generic, so we believe our results bode well for other similar domains, e.g. computer usage.”
To incentivize further experimentation in the space, OpenAI has partnered with the MineRL NeurIPS competition, donating its contractor data and model code to contestants attempting to use AI to solve complex Minecraft tasks. The grand prize: $100,000.
Joel Khalili is the News and Features Editor at TechRadar Pro, covering cybersecurity, data privacy, cloud, AI, blockchain, internet infrastructure, 5G, data storage and computing. He's responsible for curating our news content, as well as commissioning and producing features on the technologies that are transforming the way the world does business.