Even the biggest data brains need a body
Modern data infrastructure has a problem
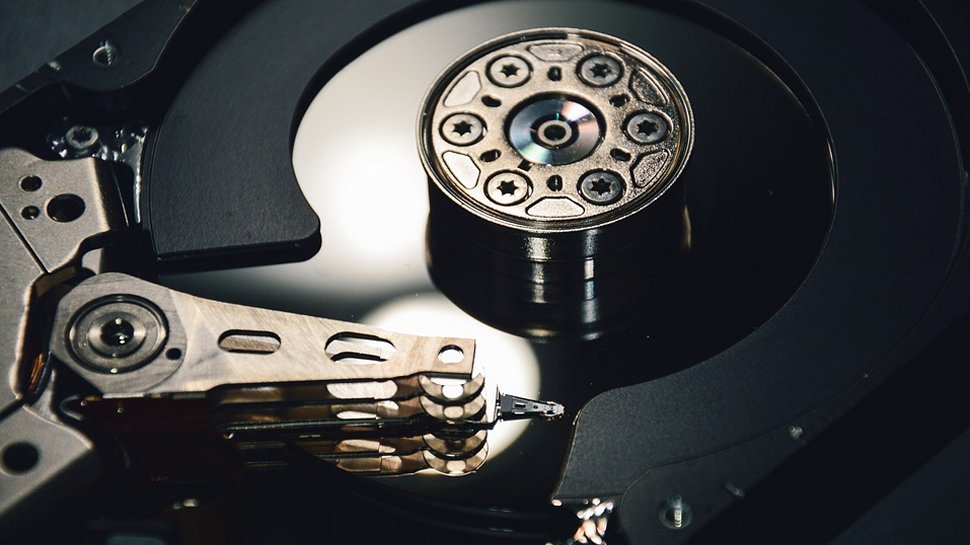
Modern data infrastructure isn’t shaping up well. Eager to base decisions on solid insights, companies have tasked their analysts with gathering information from almost any process: winding up with huge data pools, but often lacking the means to use them. Or in simple terms: creating massive data brains yet lacking the body needed to take action or connect the information into a strategy.
These knowledge stores are frequently leaving powerful knowledge untapped, which may be a major part of the reason why recent Gartner research found less than half (44%) of data and analytics teams effectively provide value to their organization. To avoid this waste, firms must re-prioritize the ultimate purpose of data collection and start building an efficiently integrated pipeline that brings actionable insight to the fingertips of every user.
Cameron Beniot is Director of Solutions Consulting for US at Adverity.
Laying the right pipeline
Advances in no-code development have brought us closer to self-service data interrogation and query resolution, with not-so-technical-users being able to manage and adjust solutions directly. Most standard data-as-a-service offerings, however, still don’t cover the whole orchestration process. Instead of delivering functioning warehouses where users can retrieve boxes from organized shelves, basic funnels and API connection hubs provide chaotic amalgamated data that requires manual sorting.
Although frustrating, these restrictions aren’t necessarily the key difficulty here; issues are more about unrealistic expectations. Companies can’t assume onboarding any ETL system will guarantee neatly packaged data. To leverage data brains efficiently, they need to build a strong body to ensure efficient pipeline configuration:
1. Efficient transformation means a better tomorrow
Transferring raw data into systems tends to pass on more problems. Building setups that simply collect a pile of data directly from multiple APIs may seem like a smart way of driving fast access and activation, but such corner-cutting increases how long it takes to mobilize data swamps. Ultimately, time invested in earlier cleansing and consolidation saves on effort and prevents inefficiency for all business users.
Deeper consideration of integration is therefore vital. Going back to our body analogy, connections between bones, tissues and vessels will obviously vary. To match data flows with cross-business needs, users should be involved in the initial stages of engineering: giving feedback around key attributes and dimensions to inform where pipelines are laid and linked, as well as which insights appear in final dashboards.
2. Pipeline blueprint
Once unique user requirements are factored in, focus can then move to configuring pipeline construction for minimal friction and maximum value. And to do so, there are four core traits every pipeline should have:
- Automated data onboarding: Whether data is gathered via API, database, file upload, or another option, streamlining importing is key to speed up overall processing.
- Smart unification: Similarly, building setups capable of instantly combining, syncing, harmonizing, and unifying multi-source data will better support agile transformations, where users can shift, slice and dice data for different activation specifications.
- Simple access: Cleansing and storing data for immediate availability is paramount for users to quickly answer important questions. Typically, this need is best served by a database or tool with an integrated storage component that scales well (not relying on spreadsheets).
- Prioritize user experience: Making insight retrieval, application, and visualization easy is just as vital to fuel productive daily operations. In addition to finding — and filling — gaps between what data sources provide and users need, this can include removing unnecessary and duplicate data, building specialized calculations for specific use cases, and mapping data under category fields or groups that users can instantly recognize and filter by. The most useful tends to be specializing your campaigns and sneaking dimensional values into your campaign names broken up by a delimiter, splitting the campaign name into individual columns, then using these columns as newly created filters on your dashboards for personalized analysis.
3. Scale-ready Standardization
The headline advantage of automation is, of course, making data transformation easier and doing so at scale. While it’s probable that customized data segments will need to be built out for a certain number of users, say 20%, an even bigger portion will be using slight variations on the same sources, which creates scope for reusable automation across the wider 80%.
Finding overlap in data needs and use cases will allow data specialists to boost efficiency; investing initial time in establishing core transformation processes that are then rolled out to the majority of business users. From there, they can identify which aspects of standardized flows need custom adaptation. Moreover, additional elements of automation can also lighten the load of data coordination for all users.
Introducing data schema mapping, for instance, will help tackle minor issues that significantly increase time to value, including instantly filing similar fields under a single column to fix discrepancies created by different naming conventions.
In my role, I’ve talked to hundreds of businesses experiencing data challenges and most know they have a problem. Many can even pinpoint the specific data cleansing method or transformation type that’s lacking in their current setup. What few recognize, however, is the reason they’re coming up against these blockers, again and again.
Building systems for pure volume almost invariably means companies will find themselves with an immense data brain, but little means of using it. That’s especially true if they expect too much from data management tools. Successfully applying data brains means more focus on time plotting out the anatomy of their data setup: working on identifying, building, and standardizing the processes that will bring each user a daily dose of fresh, usable data.
Sign up to the TechRadar Pro newsletter to get all the top news, opinion, features and guidance your business needs to succeed!
Cameron Beniot is Director of Solutions Consulting US at Adverity, joining in May 2020. Prior to joining, Cameron provided consulting services for some of the world's biggest brands, overseeing large-scale process mining projects focused on minimizing manual tasks by pinpointing opportunities for enhanced automated efficiency.