What is DataOps and why does it matter?
DataOps adds value to an organization
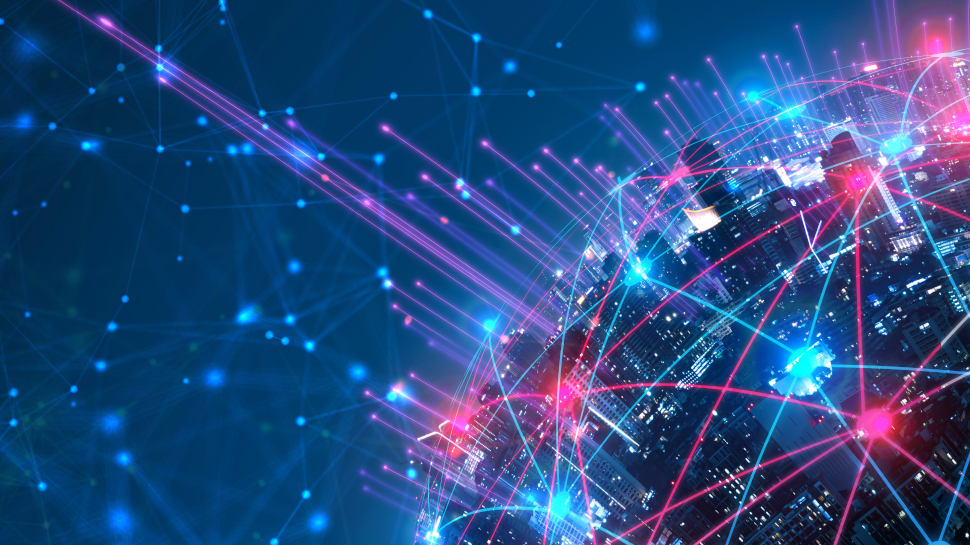
The challenge of not yet knowing how to integrate their enterprise-scale data platforms is one that many organisations are now dealing with. Their failure to make their operations data-driven hinders their capacity to accomplish strategic goals.
Alan Hayward is the Sales and Marketing Manager at SEH Technology UK.
That is where the solution of DataOps comes into play; the process of increasing the value of data in a dynamic context by automating the distribution of that data with the proper level of cybersecurity and quality.
Introducing DataOps
The first objective with DataOps is to satisfy the customer by promptly and consistently delivering insightful analytic data, which might range from a few minutes to a couple of weeks. The extent to which insightful analytics are supplied, combining correct data on top of reliable frameworks and systems, is the key performance indicator for data analytics.
Accepting changing consumer demands allow organizations to gain a competitive edge. Face-to-face conversations are the most successful, efficient and agile forms of communicating with clients. There will always be a range of responsibilities, abilities, preferred tools and titles in analytical teams.
Throughout the project, daily collaboration between the operations, analytical teams and customers is required. Self-organizing teams produce the best analytical insights, algorithms, architectures, requirements and designs.
Analytic teams should work to eliminate heroism and develop sustainable and scalable data analysis teams and procedures as the pace and scope of the need for analytic insights continue to grow. They should improve their operational performance, by periodically reflecting on input from their clients, themselves and operational statistics.
To access, integrate, model and visualize data, teams employ several separate tools. In essence, each of these tools creates configuration and code that outlines the activities applied to data to provide insight.
Are you a pro? Subscribe to our newsletter
Sign up to the TechRadar Pro newsletter to get all the top news, opinion, features and guidance your business needs to succeed!
Analytics is manufacturing
An important factor in the effectiveness of analytics is the seamless orchestration of the data, tools, code, environments, and the work of the analytic teams. Due to the need for reproducible outcomes, all information needs to be managed, including data, low-level hardware and software configurations, and the code and configuration unique to each tool in the toolchain.
By providing analytical team members with disposable technical environments that are simple to establish, safe, and isolated and replicate the production environment, it's crucial to keep the cost of experimentation for analytical team members to a minimum. Agility is enhanced by the constant focus on technical quality and sound design, and simplicity — the art of maximizing the amount of effort avoided — is also crucial.
Lean manufacturing lines and analytical pipelines are comparable. Focusing on process thinking to obtain continual efficiencies in the production of analytic insight is a basic component of data operations.
The basis of analytical processes should be able to automatically detect anomalies in code, configuration, and data and should be able to continuously report back to operators to help them avoid making mistakes.
To identify unexpected variations and provide operational statistics, it is imperative to have performance and quality measurements that are regularly monitored.
Avoiding the repeat of prior labor by the person or team is a fundamental part of analytic insight manufacturing efficiency. The time and effort it takes to transform a client demand into an analytical concept, develop it, deploy it as a repeatable production process, and then rework and reuse that product should be as brief as possible.
Later, it developed to the point where it could stand alone as a brand-new data analytics methodology. DataOps understands the interconnectedness of the data analytics team and information technology operations and applies it to the whole data lifecycle, from data preparation to reporting.
Integrating DataOps with DevOps
DataOps integrates agile software development, DevOps, and the statistical process control used in lean manufacturing to data analytics from a process and technique viewpoint. Consequently, DataOps may be described as an agile technique that strives to optimize the creation, maintenance, and analysis of data-driven systems.
Big Data ventures needed a technique that would increase their chances of success, which is why DataOps was developed. The cause was the extremely poor data findings that businesses were displaying.
Nearly 60% of Big Data projects, according to a 2016 Gartner estimate, failed. Instead of declining in 2017, this percentage increased to 85%. A Harvard Business Review poll conducted in 2021 revealed that just 24% of businesses awarded the outcomes of their analytics programs a 7 or above. This number raises serious concerns. Data must be regulated and presented in reports and dashboards in addition to being simply kept, for it to be a dynamic component of business activities.
Many organizations discover that they have issues with their data and how to make the most of it. One of the keys to encouraging improvements that support a company's objectives is DataOps. By eliminating data duplication, it makes it easier to produce more useful, high-quality, and operationally available data products. Creating a data strategy helps teams collaborate at all phases so that data is available more rapidly.
Final thoughts
Making use of machine learning algorithms allows better data analytics allows for the collection, processing, and analysis of massive volumes of data. There is also optimum operational efficiency in terms of speed, agility, security, and revolutionary change.
Advanced processes allow businesses to execute their digital transformation objectives and migrate more rapidly to the cloud. Support for automation technologies by automating operations that formerly required manual labor, excellent data supply is made possible.
DataOps is a very helpful tool, but it's crucial to understand the requirements and goals of each company before using it. To ensure the success of the investment, it is crucial to have a partner that can provide that viewpoint.
Alan Hayward is the Sales and Marketing Manager at SEH Technology UK.